Issue:March 2016
CLOUD COMPUTING - Using Quantum Molecular Design & Cloud Computing to Improve the Accuracy & Success Probability of Drug Discovery
INTRODUCTION
Organizations that develop drugs cannot afford to stick to traditional costly and time-consuming methods of drug discovery. Nor can they remain mired in outdated thinking that focuses on the past failures of computational drug discovery. A number of newer approaches are yielding faster, more accurate, and more affordable results.
Cloud computing combined with the continued influence of Moore’s Law has provided an unprecedented opportunity to apply inexpensive high-performance computing to drug discovery. This combination has now made the cloud the most promising venue for drug discovery and offers new techniques that can be combined to achieve success at a higher level.
The costs and timelines to develop effective medicines using traditional drug discovery methods are much too high, exacerbated by outrageous failure rates – resulting in very high costs that are passed onto consumers and threatening the financial stability of our healthcare system.1,2 It’s well documented that out of 10,000 discovered compounds, less than one makes it past preclinical screening and clinical trials to achieve FDA approval. This high failure rate drives up much of the cost in traditional drug discovery and development. Methods like high-throughput screening (HTS) offer some promise to reduce failure rates and improve time to market. But this process does not generate novel molecules – so we’re back to high costs and staggering timelines, and challenging IP scenarios. Previous in silico methods seemed to offer the promise of a better way but have not been accurate or robust enough.
Cloud computing offers a solution by enabling more sophisticated computational chemistry coupled with rapid synthesis and optimization that helps companies identify compounds that are better suited for development from the outset. Selecting compounds with a higher probability of success then acts as a lever to reduce overall cost, which is a necessity if we’re to achieve personalized medicine or “niche buster” drugs.
HOW CLOUD COMPUTING IMPROVES UPON TRADITIONAL METHODS
Deeper computational chemistry via cloud computing helps address two of the major problems with traditional drug discovery. It helps improve efficiency by picking better compounds to bind with biological targets and have good “drug-like” properties. But the goal is to not only reduce cost and improve speed. It’s about producing much improved compounds for really hard-to-drug targets.
Cloud computing helps in the design of compounds for hard-to-drug targets by providing extensive computing power so that more time-consuming but atomically accurate in silico design methods can be deployed. It identifies molecules from novel chemical space – not the same screening decks that have been used before and failed. Targets that were once difficult to drug are showing promise through the use of more computational power via cloud computing. For example, traditional algorithms are ineffective on targets with limited structure information such as G-protein-coupled receptors (GPCRs). However, deploying sophisticated homology modeling with molecular dynamics methods helps gain structural information, that when combined with quantum chemistry in the cloud, as well as searching molecular space for novel molecules, can result in promising compounds for previously undruggable targets.3
A NOVEL APPROACH TO DRUG DESIGN & DISCOVERY
One approach that illustrates some of the ways the cloud is helping to improve drug design and discovery is based on pioneering work developed at Duke University. The Beratan and Yang groups at Duke University had developed novel algorithms to search chemical space. In its first implementation, the linear combination of atomic potentials (LCAP) used molecular characteristics as a function of parameters that define the contribution of a specific chemical group at a particular chemical site in a molecule (Figure 1).4
This method enables the construction of a potentially enormous novel space of chemical structures at a cost far below the factorial cost of individual structure evaluation. The LCAP method has been published4-6 and experimentally tested7 as well as shown to have several advantages, such as multiple search methods and ease of parallelization. Initially starting with the LCAP method, more sophisticated search algorithms for accessing novel chemical space have been developed by Cloud Pharmaceuticals.
Cloud Pharmaceuticals has improved and revised Duke’s original approach to allow versatile, multiple, and chemically diverse molecules with its Quantum Molecular Design process. The Quantum Molecular Design algorithm is a novel implementation that is based on a proprietary combination of transformation between chemical and vectorial space and integer-programming methods to result in better identification of totally novel compounds that result in reduced medicinal chemistry effort and greater freedom to operate. Cloud Pharmaceuticals’ objective is practical drug design, as opposed to academic pursuits which often take researchers into expensive and intractable directions.
SPEEDING THE DISCOVERY OF NOVEL DRUG CANDIDATES
Quantum Molecular Design uses molecular modeling in the cloud to design drug candidates and identifies companion biomarkers through a partnership with Genomeon. Quantum Molecular Design improves upon traditional methods in three ways. First, it offers a novel way of searching for new molecules. It identifies molecules that are designed directly from the characteristics of the binding pocket of the biological target using a proprietary AI / “big data” approach.
Second, Quantum Molecular Design offers very accurate prediction of the binding affinity between a protein and a small molecule (or peptide). The process uses an optimal combination of methods adapted to the target class and molecule type, which includes one or more of: the quantum mechanics/molecular mechanics (QM/MM) method, molecular dynamics (MD), linear interaction energy (LIE), and/or free energy perturbation (FEP), with parametric adaptations. Results have repeatedly yielded correlations of 0.7–0.9.8,9 Specifically, it describes with atomic details the protein, molecule, and water environment. It accurately represents the role of water in solvation and in the protein active site, including a high sampling rate. It samples conformation of the protein-binding pocket as well as the small molecule and water molecules, and accurately predicts the interaction between them.
Third, Quantum Molecular Design filters molecules for chemical properties that are desirable for drugs. Results then yield only molecules that make it through all of the filters. For example, you can filter molecules based on solubility, synthetic tractability, or if the molecule crosses the blood-brain barrier. There may be molecular weight constraints for some designs that need to be filtered. Or, there may be side chains that are unstable or undesirable that you want to filter out of some designs for biological reasons. The property-filtering tools in Quantum Molecular Design help yield only “good” molecules that substantially reduce the work of the medicinal chemist during optimization.
ACCURATELY PREDICTING LIGAND BINDING
Using the methods above helps improve binding accuracies by employing a multi-scale, multi-resolution approach. This approach has a ligand solvation term calculated with implicit solvation with a high sampling rate and flexible protein, flexible ligand, explicit water model.
For example, this methodology was applied to the Janus family of kinases in order to discover novel inhibitors of the JAK3 enzyme that is selective against other proteins from the Janus family.10
Due to different activities, each JAK protein is targeted toward different diseases: JAK3 for rheumatoid arthritis, immunosuppression, and inflammation; while JAK2 is targeted for cancer. Cloud Pharmaceuticals has designed inhibitors targeting JAK3 that do not target other members of the family, which produce more focused results with fewer side effects.
CASTING THE NET INTO MOLECULAR SPACE
Quantum Molecular Design searches novel space by extracting a binding pattern from an x-ray structure of the target (Figure 2). This pattern is based upon the 3-D shape of the target’s binding pocket. The pattern is then queried against an enormous and growing database of scaffolds that contains all scaffolds published in literature, many theoretical scaffolds created combinatorically, and includes liberal hopping of those scaffolds to make many others. After querying that database with the binding pattern, you get a list of molecular matches. Any of those matches are scaffolds that have been stripped down to extract only the root of the scaffold.
Quantum Molecular Design then expands the scaffolds by varying the functional groups in every position of all of the scaffolds that produced a good match to create a novel chemical space, including only stable functional groups. This approach results in 10 million to a billion molecules depending upon the depth of study and the number of scaffolds or roots considered. This fast and efficient algorithm is done in the cloud and produces a novel, diverse, and relevant virtual molecular space exponentially faster.
QUANTUM MOLECULAR DESIGN WORKFLOW
The way Cloud Pharmaceuticals applies this process is to first select the target and establish a 3-D model of the target from its x-ray structure or from homology. If training data is available, it is applied to help improve results (Figure 3). The next step is chemical space generation, using the method described in the previous section. The process then ventures into the cloud to identify molecules with the strongest match to the desired properties.
At each step, several thousand molecules are scored using an optimal binding affinity and solvation algorithm. Based on the calculated affinities in each step, the head node redirects the search, based on the previously explained search algorithm, and continues until achieving optimum results in rank order.
The process can typically be completed in 1 week with 3,000 cloud nodes or 3 weeks with 1,000 nodes – the process is embarrassingly parallel and scales accordingly.
The next step is the beginning of the preclinical optimization process in which you take the rank-ordered hit list of matches, filtered for drug-like properties, and work with a medicinal chemist to pick the molecules to synthesize. Many of Cloud Pharmaceuticals’ projects begin with synthesizing a small number of molecules – perhaps 10 or 20 with the highest scores. This preliminary experimental work includes synthesis, chemical characterization and measurements, and conducting binding assays and cell-based assays. If the results are not satisfying from the first batch, you can then analyze additional molecules systematically in rank order, accounting for diversity.
Typically, de novo design of drugs requires 500,000 CPU hours in the cloud, but this may vary based upon the depth of coverage desired. The Quantum Molecular Design process has been deployed on multiple cloud services: Amazon Web Services, ProfitBricks, Cloud & Heat, Google, and Microsoft Azure. Due to the method flexibility, it can run on almost any cloud with minimal reconfiguration.
Because Quantum Molecular Design generates novel molecules – not those from an existing screening deck – the process provides much greater freedom to operate than traditional HTS. This method has been validated by correlation with reported lab data, and by synthesizing totally new compounds and successfully conducting assays showing activity. This results in lead identification, producing compounds suitable for further optimization based on biological models.
PRECLINICAL DEVELOPMENT
Following the work completed in the cloud, the carefully screened hit list is ready for further preclinical development. Because you’ve eliminated many candidates that have a high probability of failure, this step should go much more efficiently. While drug designers still have to complete all of the customary preclinical development steps, they’re being applied to a much more qualified list of candidates, resulting in a much higher success rate, more rapid progress, at lower cost, and on novel and hard-to-drug targets. Cloud Pharmaceuticals partners with contract research organizations (CROs) and risk-sharing partners to perform many of these steps.
The power of the cloud is that it yields novel molecules for hard targets and offers improved results that lower costs and speed time to market. Further, collaborative drug discovery and development can be securely managed among multiple partners via the cloud, which is what Cloud Pharmaceuticals does. The company integrates the efforts of its partner network to carry out a great deal of the drug discovery, design, and development efficiently, in addition to using the cloud for computational power in design work.
DE NOVO DESIGN IN THE CLOUD
The significance of the approach detailed here is that the hit list and early lead identification steps are all performed in the cloud, until synthesis. Further, the composition of matter patents that result are more powerful because they include many compounds in the same nexus from a common process. The “hits” produced are easier for the medicinal chemist to turn into good “leads” because good drug-like properties are already built in.
In fact, Cloud Pharmaceuticals is currently working with an emerging life sciences data center in Iceland, where it expects to preemptively design inhibitor libraries for the entire druggable genome and known mutations thereof, based on data reported by the Human Proteome Project, a multi-year project expected to be disruptive to HTS.
This process has been applied to many single-target projects ranging from kinases to allosteric proteins, GPCRs, and other receptor proteins. The company is working with a long list of targets, including Aurora-A, BACE1, betaCR, DHFR, eiF4E, HDAC8, hsp90, JAK3, MetAP2, Metnase, PKCe, PKR, PLA2, ROCK2, and Serpins – to name a few. Quantum Molecular Design can be applied to any of these targets and begin with x-ray structure or with homology.
REFERENCES
1. Mullin R. Cost to develop new pharmaceutical drug now exceeds $2.5B. Scientific American. 2014. Website visited: http://www.scientificamerican.com/article/cost-to-develop-new-pharmaceutical-drugnow-exceeds-2-5b/.
2. Morgan S, Grootendorst P, Lexchin J, Cunningham C, Greyson D. The cost of drug development: a systematic review. Health Policy. 2011;100(1):4-17. Doi:10.1016/j.healthpol.2010.12.002. Epub 2011 Jan 21. http://www.ncbi.nlm.nih.gov/pubmed/21256615.
3. Sekharan S, Wei JN, Batista VS. The active site of melanopsin: the biological clock photoreceptor. JACS. 2012;134(48):19536-19539. Website visited: http://dx.doi.org/10.1021/ja308763b.
4. Wang M, Hu X, Beratan DN, Yang W. Designing molecules by optimizing potentials. JACS. 2006;128(10):3228-3232.
5. Keinan S, Hu XQ, Beratan DN, Yang W. Designing molecules with optimal properties using the linear combination of atomic potentials approach in an AM1 semiempirical framework.” J Phys Chem. 2007;A 111:176-181.
6. Keinan S, Therien MJ, Beratan DN, Yang W. Molecular design of porphyrin-based nonlinear optical materials. J Phys Chem. 2008;112(47):12203–12207. Website visited: http://dx.doi.org/10.1021/jp806351d.
7. Keinan S, Paquette WD, Skoko JJ, Beratan DN, Yang W, Shinde S, et al. Computational design, synthesis and biological evaluation of para-quinone-based inhibitors for redox regulation of the dual-specificity phosphatase Cdc25B. Org Bimolec Chem. 2008;6(18):3256-3263.
8. Menikarachchi LC, Gascón JA., QM/MM approaches in medicinal chemistry research. Curr Top Med Chem. 2010;10:46-54. ISSN (Print): 1568-0266.
9. Senn HM, Thiel W. QM/MM methods for biological systems. Top Curr Chem. 2007;268:173–290. Doi: 10.1007/128_2006_084.
10. Thompson JE. JAK protein kinase inhibitors. Drug News Perspect. 2005;18(5):305-310.
To view this issue and all back issues online, please visit www.drug-dev.com.
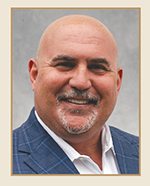
Ed Addison is the CEO of Cloud Pharmaceuticals, a therapeutics company focused on cloud-based drug design and development he co-founded in 2009. Mr. Addison is a serial entrepreneur who has founded three previous ventures, two of which successfully merged with public companies in deals worth $55 million. He has a unique and strong blend of in-depth business and technical experience in biotechnology and in information technology.
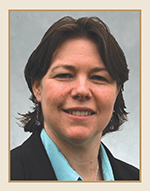
Dr. Shahar Keinan is the Chief Scientific Officer and co-founder of Cloud Pharmaceuticals. She has over 20 years of extensive experience in the field of computational and theoretical chemistry. Dr. Keinan earned her PhD in Theoretical Chemistry from The Hebrew University of Jerusalem and has numerous papers and presentations in the fields of in silico drug design and discovery, as well as molecular materials design and computational methods development to her credit.
Total Page Views: 5391