Issue:May 2020
RISK-BASED MONITORING - Making the Move to RBM: Improving Patient Safety & Data Quality With Real-Time Insights
INTRODUCTION
In an era in which the time- and resource-intensive business model of developing blockbuster drugs has been supplanted by a push to move drug candidates through the pipeline with greater speed, accuracy, and quality, risk-based monitoring (RBM) and real-time data analytics are becoming a must-have for clinical trials. Taking a risk-based monitoring approach can help enhance the safety, quality, and efficiency of clinical studies, while supporting regulatory compliance.
WHAT IS RBM?
In traditional monitoring, clinical research associates (CRAs) check every data point reported by an investigator against source records with the goal of achieving 100% source data verification (SDV). However, studies have shown that SDV is not necessarily synonymous with data quality.
RBM represents a paradigm shift from traditional monitoring methods. RBM utilizes a combination of monitoring strategies, including a greater reliance on centralized monitoring and statistical assessments to guide site monitoring visits and a focus on advanced technical capabilities. The goal of RBM is to bring together the relevant metrics and data necessary to increase efficiency, safety, and quality and to make data-driven decisions. Targeted monitoring replaces pre-scheduled site visits with data-triggered ones, concentrating on sites with a higher workload and a greater need for support and monitoring. Centralized remote monitoring and risk-based SDV helps reduce the number of data points CRAs must verify against source data on site, reducing workload and time.
TRENDS BEHIND THE RISE OF RBM
RBM has been hotly debated since the US FDA published its industry guidance Oversight of Clinical Investigations – A Risk-Based Approach to Monitoring in 2014. However, adoption has been slow and industry has been uncertain about implementation. In recent years, the trend toward RBM has been accelerating, fueled by a number of key factors.
Changes in the Regulatory Environment
RBM is becoming the regulatory standard for clinical monitoring. With the 2016 updates to the ICH Guidelines for Good Clinical Practice, sponsors are now tasked with a greater responsibility for trial oversight, including the use of a formal approach to quality management that integrates technology and leverages real-time information to drive a more structured approach to risk.1 In April 2019, the FDA published the draft guidance A Risk-Based Approach to Monitoring of Clinical Investigations: Questions and Answers, providing additional direction to help industry operationalize and implement risk-based monitoring.2
Shifts in Development Priorities
Throughout the past few years, changes in policy and a greater emphasis on developing new therapies for rare diseases have changed the clinical trial landscape, as an increasing number of studies are being launched with fewer participants. According to a study from the 2019 National Organization for Rare Diseases (NORD) and Orphan Products Breakthrough Summit, 34 of the novel drug approvals in 2018 were for rare or orphan diseases, representing a historic high since the Orphan Drug Act was passed in 1984.3 The small patient populations and low data volume in rare disease trials underscore the importance of making every data point count.
Drive Toward Digitization
Industry-wide emphasis on modernizing and improving the efficiency of clinical trials is driving digitization of the entire development process, from recruitment and data collection to adherence and data analysis. In January 2019, then-FDA Commissioner Dr. Scott Gottlieb indicated that modernization of the clinical trial process would necessitate the pairing of real-world data with advances in machine learning to “help expand the sources of evidence [used] to make more reliable treatment decisions.”4
Exponential Increases in Data Volume
The volume, velocity, and variety of data generated by clinical trials is increasing exponentially. Despite advances in technology, time spent collecting, cleaning, and organizing data sets still accounts for 50% to 80% of data scientist time, leaving little time for data analysis.5 Automated solutions can help address this pain point.
Demand for Data Quality
Outside of investigator fees, clinical monitoring is the biggest driver of clinical costs, accounting for over 60% of labor costs and approximately 30% of total expenses.6 However, research has shown that expenditures related to source data verification do not necessarily correlate with improved data quality.7
BENEFITS OF IMPLEMENTING AN RBM TECHNOLOGY
RBM offers a responsive, real-time approach to clinical trial monitoring and risk management. Beyond providing a way to view and visualize risks, a comprehensive RBM solution provides insight into what the data mean in the context of the study and offers actionable steps to make optimal use of that data for in-trial decision making. Comprehensive implementation of RBM has the potential to bring a number of clear, measurable benefits.
From a compliance standpoint, regulators are seeking more rigorous assurances of patient safety and data quality. Selecting a robust RBM platform with assurance, traceability, and a built-in audit trail helps drug developers stay compliant with current and emerging regulatory requirements.
More advanced RBM software may utilize machine learning to alert decision-makers about potential risks that were not previously identified during trial set-up. These technologies analyze prospective data collected during the trial on an ongoing basis to detect patterns and anomalies, automating the time-intensive process of data analysis.
RBM technology can track trend information and make actionable data readily available to sponsors and CROs so they can take corrective action, which can improve both patient safety and data quality. In addition, by determining when it makes sense for monitors to perform site visits based on study-specific risk thresholds, RBM analytics can help reduce unnecessary on-site monitoring and focus resources on sites that require more support.
Finally, RBM can help shorten the timelines for evaluating study data. Data cleaning at the end of a study can be time-consuming, typically requiring three months or more. With RBM technology, data are evaluated on an ongoing basis throughout the course of a clinical trial, which helps minimize the time from study end to the reporting of study results.
SELECTING AN RBM TECHNOLOGY
A comprehensive RBM system approaches risk in all of its layers (operational, safety, and quality), from initial risk identification and assessment all the way through to ongoing risk review.
Risk Identification & Assessment
The system should allow users to identify and log critical study-related data and processes. It should also let users evaluate and characterize risk both at the start and throughout the course of the study using a built-in risk register.
Risk Control & Mitigation
For each of the risks identified, the RBM technology should have the ability to control and mitigate the risk through a variety of automated or manual risk management strategies. For example, the software should be able to issue alerts when risks approach pre-defined thresholds and when safety or data quality is at risk. By remotely monitoring for signals that might require an in-person visit to a trial site, sponsors and CROs can be more judicious about how and when they deploy their staff to sites. Consequently, on-site visits can be scheduled only when the RBM technology signals that such visits are needed, rather than scheduling site visits at regular intervals.
More advanced RBM software may utilize machine learning to alert decision-makers about potential risks not pre-identified during trial set-up. Rather than relying on historical data, which can be misleading and/or out-of-date, a robust RBM system should be able to analyze the prospective data collected during the trial in an ongoing manner to find patterns and anomalies. Furthermore, all of the information in the system should be fully traceable through audit trails.
Risk Communication & Action
Risk detection is only one part of the risk management continuum. The system should also allow for streamlined, centralized review of the risks detected by offering statistical models, intuitive data visualization, and the ability to drill down into the data. The software should also let users act on and close out risks through built-in workflows and ticketing functionality. This functionality should allow for targeted actions to follow-up, close, and prevent such signals in the future.
Risk Review & Updating
Finally, the RBM software must accommodate regular and ongoing risk review and modification, enabling sponsors and CROs to monitor the effectiveness of risk management activities and ensure that these activities remain effective in the context of emerging knowledge and experience. As such, the Risk Assessment and Risk Mitigation plan undergo periodic changes that may include additions, modifications, and deletions of items.
MAKING THE TRANSITION TO RBM
RBM technology can help sponsors and CROs implement comprehensive monitoring strategies from an integrated platform with automated workflows and advanced analytic capabilities. Navigating the transition to RBM can be complex, especially as RBM software solutions become more sophisticated. To help make the transition more seamless, below are strategies for addressing some of the key challenges associated with adopting an RBM approach.
Overcoming Resistance to Change
Drug developers may feel cautious about or even resistant to transitioning to RBM. This resistance may be due, in part, to uncertainty about how the FDA will respond to the use of these newer techniques. However, sponsors and CROs should also consider how regulatory agencies might respond if RBM modifications are not made. For companies that are cautious about making the shift to RBM, it may be useful to evaluate the factors underlying that hesitation and to determine how to address it.
Even for companies that are ready to transition to RBM, the change can be daunting. The move toward an RBM approach requires change management related to people, process, and technology. Commitment at the executive level, along with comprehensive staff training and a clear understanding of each team member’s roles and responsibilities, helps ensure that RBM is incorporated into the fabric of both the company and the clinical trial.
Clarifying Uncertainty About Implementation
As clinical trials become more complex, RBM technologies have also become more sophisticated. Unlocking the full value of RBM technology requires proactive planning, thoughtful implementation, and ongoing strategic execution following deployment. The process of implementation involves requirements gathering, planning, and testing, and should cover five key areas:
- Project Scope – The scope should be aligned with the needs of the end user(s) and should include clear definitions of roles and responsibilities. Sponsors may want to consider starting with a pilot of the technology, so they have an opportunity to familiarize themselves with the implementation process, test the system, and validate internal processes on a small scale.
- SOP/Plan Impact – This entails reviewing existing procedures and plans and updating them or creating new processes, if needed. This is also a good time to document the internal escalation process for handling issues identified by the system.
- Communication – This describes how the sponsor will communicate with both the technology vendor and the end user(s).
- User Acceptance Testing (UAT) Plan – This is an area many sponsors overlook. UAT should be performed at both the system level and the study-specific level.
- Oversight Plan – This involves outlining success and value metrics, and how these metrics will be communicated to all stakeholders.
Training Algorithms for Machine Learning-Based RBM Systems
In some RBM technologies, machine learning is being used to support data processing, analytics, visualization, and decision-making. The use of machine learning creates an opportunity for researchers to process, analyze, and visualize more data than ever before, but it is not intended to replace human resources. Machine learning-based RBM technologies are only as effective as their algorithms, and their outputs still need to be interpreted and contextualized by trained trial management staff. In short, machine learning is a complement to, rather than a substitute for, human judgment. Machine learning algorithms “learn” from their users by observing their interaction with the results and gathering feedback. This will further help improve the algorithm’s accuracy and effectiveness. This is especially important for clinical trials on rare diseases, where historical data is lacking or limited.
Sponsors should keep in mind that the accuracy and efficacy of machine learning algorithms are dependent on the quality of the data. In computer science, “garbage in, garbage out” describes the concept that flawed input data produces nonsense output. As such, machine learning algorithms will generate signals based on any valid raw data format, regardless of what those data are. In the context of a clinical trial, a false positive or a false negative could have significant downstream impact on patient safety and study integrity. Consequently, it is important to never act on a result without questioning it first. Algorithms are designed to facilitate decision-making, not to dictate action, so their outputs should always be validated by researchers.
KEY TAKEAWAY
When implemented and managed appropriately, RBM technologies are powerful tools for monitoring risk and improving both patient safety and data quality. Comprehensive RBM technologies approach risk from all angles, alerting users when risks approach predefined thresholds and when safety or data quality is at risk of compromise. In addition to making on-site monitoring more efficient, RBM software provides ongoing oversight of patients, sites, and the study as a whole to facilitate informed decision-making. By combining risk-based approaches with advancements in technology, RBM helps sponsors implement comprehensive monitoring strategies and focus resources towards the monitoring practices that have the greatest impact on the quality of both patient safety and clinical trial data.
REFERENCES
- International Council for Harmonisation of Technical Requirements for Pharmaceuticals for Human Use. Guideline for Good Clinical Practice E6 (R2), November 9, 2016. Available at http://www.ich.org/fileadmin/Public_Web_Site/ICH_Products/Guidelines/Efficacy/E6/E6_R2__Step_4.pdf.
- U.S. Food and Drug Administration. A Risk-Based Approach to Monitoring of Clinical Investigations Questions and Answers, March 2019. https://www.fda.gov/media/121479/download. Accessed October 24, 2019.
- MD Magazine. Approvals for Rare Diseases Reached Historic High in 2018. MD Magazine website. Published October 24, 2019. https://www.mdmag.com/conference-coverage/nord-2019/approvals-rare-diseases-reached-historic-high-2018. Accessed October 24, 2019.
- Health IT Analytics. FDA: Real-World Data, Machine Learning Critical for Clinical Trials. Health IT Analytics website. https://healthitanalytics.com/news/fda-real-world-data-machine-learning-critical-for-clinical-trials.
- IBM. Breaking the 80/20 rule: How data catalogs transform data scientists’ productivity. IBM website. https://www.ibm.com/cloud/blog/ibm-data-catalog-data-scientistsproductivity. Accessed November 22, 2019.
- Ray S. Clinical Teams Should Re-Think Risk-Based Monitoring Costs to Improve Their Bottom Line. Cutting Edge Information, 2013. Available at https://www.cuttingedgeinfo.com/2013/risk-basedmonitoring-costs/.
- Medidata. eCRF Data Correction Rates Still a Surprise to Many. Medidata website. https://www.medidata.com/en/blog/ecrf-datacorrection-rates-still-a-surprise-to-many/. Accessed October 24, 2019.
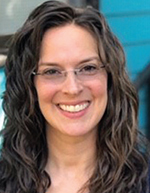
Crystal Stone is Risk-Based Monitoring Director, Customer Engagement at Remarque Systems. After a 12-year career in the medical device industry, she transitioned to the software industry to support Quality Risk Management/Risk-Based Monitoring. Her role includes management and oversight of customer engagement as well as consultant and subject matter expert to small and large pharma, medical device, and CROs, which has given her broad exposure to how companies are implementing risk management/RBM.
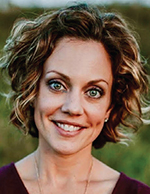
Amanda Coogan is Risk-Based Monitoring Senior Product Manager at Remarque Systems. She worked for 12 years in university hospitals and medical device firms supporting domestic and international studies across a range of disease states, then transitioned to the software industry. There she serves as consultant, subject matter expert, and project manager to small and large pharma, medical device, and CRO customers supporting the implementation of Quality Risk Management/Risk-Based Monitoring. In addition to working with customers, she provides RBQM and clinical trial operation expertise to support software product development.
Total Page Views: 4468