Issue:March 2019
ARTIFICIAL INTELLIGENCE - Practical Applications of Artificial Intelligence (AI) for Drug Data Quality & Research
INTRODUCTION
It can be tempting to explore completely new business directions based on radical innovations from full-blown, large-scale AI applications. Over-ambitious AI efforts in drug discovery remain risky and have shown few game-changing benefits.1 However, by treating AI as one more tool within a broad data quality toolkit, and by focusing narrowly on specific research and business bottlenecks, it is possible to efficiently bring real practical benefits to research and business.
CAN AI SOLVE PRACTICAL CHALLENGES IN DRUG RESEARCH?
There has been a lot of hype recently around AI, with a few advanced software applications making news in drug discovery and precision medicine.2,3 It is possible that AI will eventually deliver the ambitious, transformative precision medicine innovations that have been promised by the likes of IBM’s Watson, and Google’s DeepMind, but at high cost and risk. Many of the most ambitious AI applications that promise to transform the way we do drug discovery and delivery do represent a risk. Indeed, there have been notable failures in the recent past, and likely there will be more ambitious failures in the near future.1
Can AI make a difference for drug discovery efforts, or will the industry waste time and money on another hype cycle? There are many benefits that can be achieved from AI today at low cost and with virtually no risk. By combining traditional data quality and analysis capabilities with AI applications, drug discovery research can benefit through reduced time and cost to achieve practical goals. AI has the potential to make a positive difference in drug discovery, development, and delivery.
The following will briefly discuss main trends in AI for drug discovery before describing some basic data quality applications that can be useful in drug research.
OVERCOMING BASIC BLOCKERS
AI has struggled to hit home runs that completely transform the healthcare industry, for example, by creating virtual doctors that can diagnose and prescribe.4 However, less ambitious solutions to bottlenecks that can block research – such as data identification, extraction, quality, harmonization, and integration – are seeing real benefits.
Drug discovery research goals can benefit through improved data quality at reduced time and cost. By focusing AI on narrower but challenging and time-consuming bottlenecks, researchers have been able to reduce time and cost to identify, extract, harmonize, and integrate drug-related data. AI-enabled technologies combined with traditional data quality processes are finally moving drug discovery and precision medicine past data quality issues that have plagued biotechnology and pharmaceutical industries for decades.
COMBINING TRADITIONAL & ADVANCED TECHNOLOGIES
General AI methods include machine reasoning (MR) and machine learning (ML). First, MR builds on newer (NoSQL, Graph, and Semantic) AI-enabling database technologies to provide expert systems. MR applies semantically meaningful data models or “ontologies,” used for deductive reasoning, entailment, and decision support, even with incomplete datasets. ML applies various statistical analyses for training and learning using example datasets with features (eg, variables) and outcomes (eg, results). Next, ML applies supervised and unsupervised algorithms to analyze these training datasets, to identify features that are, or seem to be, related to outcomes for inductive hypothesis generation and to facilitate pattern identification for decision support.
Traditional methods for improving data quality in drug discovery include rules-based data quality assessment and transformation, normalization of drug terms to standardized lists or “lexicons” via scored string matching, and finally, statistical analyses of data quality. Combining the two approaches, however, creates a new and modern way of solving drug development problems. The key is to apply these technologies in focused and well-defined applications, resulting in a smarter kind of data tool.
MAKING AI PRACTICAL VIA SPECIFIC APPLICATIONS
Ideally, AI can be applied to enhance narrowly defined applications, including drug terminology normalization, enrichment of existing drug data with published information, and master data integration and management. Perhaps most importantly, core technologies that can deliver these capabilities, even when applying AI under the hood, can be delivered to the industry in convenient and accessible ways.
Example applications include cloudbased transformation APIs (specific data quality and enrichment applications); drug content resources (look-ups and knowledge hubs); and master data management (environments, workflows, databases for data extraction, harmonization, linking, search, and management). These resources make use of traditional and advanced technologies to reduce time and cost required to achieve comprehensive, clean, harmonized, standards-compliant drug data that is effectively linked to the life science data required to meet scientific and business goals today.
Let’s take a look at a few example applications of AI that have shown immediate practical benefits for drug discovery and development.
COMBINING MR WITH TRADITIONAL METHODS FOR IMPROVED DATA QUALITY AT REDUCED COST
Data quality has presented endless challenges in drug research and discovery, development and delivery. These challenges can be overcome efficiently by bringing the best of the old and new to solve practical data quality problems.
Basic issues like diverse standards for chemistry terminology can cause research and business bottlenecks. For example, in the US, the FDA requires one set of preferred terminology for submissions (such as those based on National Drug Code or NDC), but different drug discovery research also requires attention to diverse standards and data sources, including RxNorm, ChEBI, and ChEMBL, to ensure that data definitions and terms are most useful for different purposes. Traditional methods, such as standardized lexicons with synonyms and preferred terms, can often be applied to easily transform data from one standard lexicon to another.
However, cleaning up and standardizing drug terminology using traditional methods is only the first step. AI, such as MR, can be applied to solve data quality issues including:
-Identifying data, to extract useful content from documents or dirty databases
-Assigning data, to correct classes and relationships for data integration
-Enriching data, to fill in gaps with new information
How can AI fill in gaps on incomplete datasets? Ontologies from MR can be applied to create entailments, or processes that make new assertions about data based on reasoning. Let’s look at a simple example.
A researcher has a drug database with compounds and associated biological activities and mechanisms. However, there is no drug-drug interaction information.
With the database modeled in Figure 1, researchers can’t ask if these two drugs are contraindicated. Adding an ontology and MR capabilities to the environment can bring broad benefits to research.
Specifically, adding an ontology with semantic assertions makes reasoning possible (Figure 2). The database “knows” that Selegeline is an MAOI and Phenylephrine is an AR Agonist. The ontology for MR knows that MAOIs and AR Agonists are contraindicated. By bringing ontology-enabled MR to the database, we can infer that Selegeline is contraindicated with Phenylephrine. With reasoning and entailment, we can add that information to create a new, richer database (Figure 3).
It is increasingly easy for knowledge engineers and other advanced data scientists to access and apply traditional lexicons along with advanced semantic ontologies and MR to, for example, curate and integrate internal research with public content. However, these aren’t tasks for drug researchers.
Benefits for research can be delivered simply. For example, end users can plug into APIs or use web-based look-ups that apply AI quietly in the background to transform inconsistent lists of drug terms and metadata into comprehensive datasets with standardized terminology and rich information.
Uploading a messy list of drug terms and receiving validated, standardized, and enriched results within seconds can be very useful. For example, uploaded “dirty” terms can be automatically mapped to the FDA’s preferred terms, with additional data added according to interest, such as NDC codes, proprietary and generic names, FDA Product IDs, labeler names, routes, dosages, and associated biological mechanisms. Combining traditional list-based standardization with MR to identify and ensure correct matches and to enrich existing data reduces time and cost to achieve clean, rich, usefully connected data.
Check and verify that you are using preferred, correct terms for your drug or list of drugs in order to harmonize your data and comply with terminology standards. Validate millions of pharmaceutical names, variants, dosages, and spellings against a pharmacopeia to save time, clear confusion, and mitigate errors.
COMBINING ML & MR FOR IMPROVED RESEARCH
Traditional and semantic MR technologies have made it possible for vendors to integrate information from hundreds of public data sources, about hundreds of thousands of drugs and potential new drugs, including content from massive databases and peer-reviewed journal resources.5
Comprehensive reports from clean, internally integrated data and from integrated public data can provide deep information about your company’s drugs, competitors’ drugs, concomitantly prescribed drugs, contraindicated drugs, as well as relationships between drugs, genes, proteins, and diseases. But how can AI impact your basic research? By combining ML with MR, it becomes possible to gain a deeper understanding of data:
-Harness the power of ML to identify related variables and uncover patterns in data
-Reduce data dimensionality to identify and focus on the information that makes a difference
-Segment and consolidate information to classify drug general usage or bioequivalence at prescription
-Harness the power of combined general AI by applying MR to transform correlated data from ML into explanatory, causally meaningful information
ML is a more familiar form of AI. While MR requires knowledge and expertise to create and apply general ontologies to specific data challenges, MR requires clean, integrated data, particularly variable features and target outcomes. ML commonly requires training, usually in the form of acceptance or rejection of analytical results, but also by providing guidance regarding important features for analysis. ML teaches machines to identify and respond to patterns. Many traditional analytical methods are applied within ML algorithms, including Support Vector Machines, Bayesian, clustering analysis, and many others.
Common goals for ML are reducing data to key dimensions, and segmenting or clustering those dimensions according to the outcomes with which they are associated.
A classic problem with ML is separating causal signal from spurious correlations caused by statistical overfitting. ML algorithms often give a lot of statistically significant results that are eventually determined not to have any causal relationship to target outcomes.
Importantly, by combining ML with MR, it is possible to guide (supervise or train) ML analysis with existing MR ontologies. It is also possible to contextualize and test ML results. MR-enabled ontologies can detect and correct false patterns and generate new hypotheses. If new data that emerges from an ML analysis contradicts relationships defined by expert ontologies, either the new data is spurious, or a substantial new hypothesis is called for. If targeted research on causality bears out, the MR-enabling ontology may need to be updated. It is also possible to test ML results using more or less traditional methods by uploading to existing integrated databases or knowledge bases, to determine if the ML results align with what is currently understood about a particular molecule and biological mechanism.
CONCLUSIONS – EFFECTIVE GENERAL AI COMBINES TRADITIONAL, MR & ML METHODS
MR can be thought of as knowledge-based data intelligence, building on expert knowledge and flexible semantics. Most forms of MR require ontologies that describe expected data qualities and relationships in an area of interest. Computing with ontologies enables reasoning based on logic and assertion. In MR, computers are able to apply and identify patterns and create entailments and hypotheses.
Particularly when combined with traditional data quality methods, MR makes it possible to curate, enrich, and integrate large, messy, even incomplete datasets at lower time and cost. The examples provided in this article show how MR can help fill in the gaps in sparse datasets. MR can also combine with ML to make new discoveries possible with less wasted time and higher confidence.
ML can be thought of as analysis and supervision, or training-based data intelligence. ML commonly requires lots of clean data, including features and outcomes. ML also commonly requires training, based on training data (in which important features and outcomes are known) and supervised acceptance or rejection of results to guide the algorithm. ML teaches computers to identify potentially important variables and application of analytical methods, including Support Vector Machines, Bayesian, regression, visualization, decision trees/rules, random forests and many others.
ML is often more exploratory and potentially risky compared to MR. This article reviewed an example for application of ML that clusters potential drug compounds according to their performance on a multi-variable retrospective analysis of candidate compounds and target outcomes. Risks in using ML can be reduced substantially by combining ML with MR and traditional methods that help confirm or correct spurious results that are common to ML.
FOR SUCCESS WITH AI – AVOID OVERHYPED MOON SHOTS, ADDRESS PRACTICAL CHALLENGES
This short article has briefly reviewed the two main types of AI that are currently solving real-world problems in drug discovery. The possibilities are immense – by combining traditional data quality processes with advanced AI methods, computer science has only just begun solving basic time-consuming challenges that have plagued the industry for years. Specifically, blending traditional methods with advanced AI capabilities, such as ML and MR, can make it more cost-efficient to overcome basic research and business blockers, with higher quality results.
Scientists can avoid risk and ensure practical success by focusing more narrowly on specific applications that can solve tedious and costly problems, rather than by shooting for the moon with overambitious AI applications that impact core business models. AI can solve practical challenges in drug research – not only benefitting patients but also creating a new competitive landscape for drug developers.
REFERENCES
- Matthew Herper. MD Anderson Benches IBM Watson In Setback For Artificial Intelligence In Medicine. Forbes. Feb 19, 2017.
- Simon Smith. 29 Pharma Companies Using Artificial Intelligence in Drug Discovery. BenchSci. November 5, 2018.
- Sam Shead. DeepMind Is Handing DeepMind Health Over To Google. Forbes. Nov 13, 2018.
- Dom Galeon. IBM’s Watson AI Recommends Same Treatment as Doctors in 99% of Cancer Cases From quiz show champion to a weapon against cancer. Futurism. October 28, 2016.
- Florian Bauer, Martin Kaltenböck. Linked Open Data: The Essentials A Quick Start Guide for Decision Makers. Edition mono/monochrom, Vienna, Austria. 2018. ISBN: 978-3-902796-05-9.
To view this issue and all back issues online, please visit www.drug-dev.com.
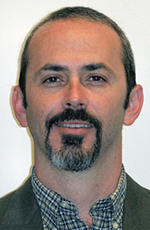
Robert Stanley is Senior Director, Customer Projects at Melissa Informatics, where he leads the company’s slate of customer projects, blending deep data quality expertise with his robust background as founder of IO Informatics. He helps clients harness the entire data lifecycle for business, pharmaceutical, and clinical data insight and discovery. Connect with Bob via email at robert.stanley@melissa.com.
Total Page Views: 8358