Issue:January/February 2023
PATIENTS-ON-A-CHIP - Why Artificial Intelligence Will Be the Tipping Point to Remove the Faulty Reliance on Animal Testing in Drug Discovery
INTRODUCTION
Advanced Bio-AI platforms that integrate artificial intelligence and machine learning with patients-on-a-chip, real-time nano-sensing, and stem cell genomic diversity technologies are the future of drug discovery and development.
The inability to predict which drugs will work safely in the human body has been a stumbling block since the advent of pharmacology. The reliance on inaccurate animal testing models to try to determine human efficacy creates massive time, cost, and safety challenges for drug development efforts. Animal testing is so ineffective in predicting clinical safety and efficacy that it is almost always wrong.
Industry leaders agree the current drug development process is flawed and are eager to help navigate the next era of pharmaceutical industry breakthroughs – using AI-powered patient-on-a-chip technology to improve predictions of clinical safety and reduce drug development cost while accelerating timelines. With regulatory changes to remove animal testing mandates underway, the path forward for AI-based technologies to disrupt decades of reliance on faulty testing models is becoming clearer.
FAULTY MODELS & HIGH FAILURE RATES
The inability to predict which drug candidates will work safely and efficaciously in the human body prior to expensive clinical trials continues to create massive challenges for drug development. On average, discovering and developing new drugs costs more than $2.6 billion over 12 to 15 years.1,2 Worse yet, an analysis of the drug development cost structure found the average out-of-pocket success-cost to develop a drug currently averages $200 million, while the out-of-pocket failure-cost runs a staggering $1 billion.3 In other words, for each successful drug developed, there is a massive, five-fold cost from failed attempts.
To date, animal testing models have served as the foundation for clinical prediction prior to clinical trials. Unfortunately, animal testing is an extremely poor predictor of clinical safety and efficacy. In fact, an astounding 89% of drug candidates that successfully pass animal testing fail in clinical trials.4-6
So why are clinical failure rates so astronomically high? According to Nobel Laureate Aaron Ciechanover, MD, DSc, “One of the main problems in drug development is the model that we are using – the mouse. We are not mice, so what works in animal-based trials is not a proper indicator of what will work for people.”
Thankfully, awareness of the limitations of animal experimentation and the increasing pressure for alternatives has led to momentous regulatory developments. The FDA Modernization Act removes an 84-year-old statute mandating reliance on animal studies and replaces them with more modern, effective approaches.7 In addition, the European Parliament recently passed a landmark resolution to phase out animal testing altogether.7
TECHNOLOGY BREAKTHROUGHS STILL MISS THE MARK
Artificial intelligence (AI) and machine learning (ML) approaches have accelerated and improved the drug discovery process by delivering qualitative, well-characterized drug candidates, but they still have not been able to solve the clinical prediction challenge.8-10 While these companies have shown measurable savings and impact, most use ML to discover new targets, invent new molecules, find drug candidates that have better molecule-target fit, repurpose existing drugs, or improve our understanding of the mechanism of action of drug candidates to better anticipate and avoid off-target side-effects.
“Our drug discovery process is broken, and technology darlings across biotech, AI, ML, and big data have not been able to overcome the colossal clinical trial failure rate,” added Dr. Kobi Richter, Founder and Chief Technology Officer of Medinol.
Existing ML platforms and organ-on-chip devices cannot offer reliable, high-throughput prediction for the clinical safety of drug candidates. Initially, AI-pharma companies relied primarily on existing data, whether it be publicly available data or data collected by other pharma and health organizations. Most AI-pharma companies today generate their own proprietary data, which they use to train the AI. However, current AI and ML models remain unable to determine if drug candidates are clinically safe and efficacious as each identified new molecule or target must still be tested to assess its actual effect in the human body.
Complicating matters further, not all biological data is created equal. Traditional organ-on-chip approaches that try to detect drug safety are modeled after antiquated animal studies: a single experiment that generates end-point data and is analyzed manually. AI that relies on traditional data points results in the same end point (mice-based trials) as previous (and woefully failing) drug development approaches, just faster. After traditional in vitro lab assays (including traditional 2D tissue cultures and other in vitro assays), they then still move into animal models, which are overwhelmingly (remember that 89% mentioned earlier) wrong when it comes to predicting if a drug candidate is safe and efficacious in the human body.
Luckily, there has been significant progress in the evolution of sophisticated 3D miniaturized organs – from the first organoid models 15 years ago, to organ-on-a-chip devices in the past decade, to robust validation as the technologies have matured in the past 2.5 years. However, miniaturized organ technologies face similar barriers as the AI/ML breakthroughs previously noted.
As the organ-on-a-chip field has evolved, its models have delivered higher levels of clinical predictiveness. Let’s start with a quick look back at the evolution of organ-on-a-chip technologies. The first breakthrough, 2D models, poorly predict clinical safety – testing a molecule on a 2D model does not predict toxicity or pharmacokinetics and pharmacodynamics (PK/PD) in the human body.7-9,11 Organoids, the most basic form of miniaturized organs or tissues, proved to offer better clinical predictiveness than 2D mono-cell, cell-line biology models.1,7 However, organ-on-chip models added fluid replacement, mechanical ques such as microfluidic “shear-flow” and/or mechanical deformation, and, therefore, can offer much more sophisticated organ mimicking for specific organs. Organ-on-chip models have proven to offer better clinical predictiveness and data fidelity than organoid models.6,7,12 A prime example, changes in stress-induced, elevated liver enzymes levels are absent in simple liver organoids but present in liver-on-chip devices in which the miniaturized liver is subjected to mechanical cues.13,14
However, despite continued advances, organoid and organ-on-chip devices are notoriously unscalable. Achieving intra-organoid variability remains elusive, and the expense and operational demands of multi-organ-on-chip technology puts it out of reach for mass research. Recent studies by the Wyss Institute used multiple interconnected organ-on-chips to demonstrate an unprecedented ability to discern drug toxicity and PK/PD, but each “patient-on-interconnected-chips” required a complex, expensive, cubic-foot size device – making it practically impossible to scale to run thousands of such experiments.8,15 These devices are extremely expensive and require intensive manual labor by a skilled scientists – making it impossible to achieve the end goal of running thousands, and ultimately millions, of clinical prediction experiments reliably and inexpensively.
Currently, there is an enormous disconnect between AI and organ-on-a-chip modalities. While AI pharma companies rely on 2D biology, which is extremely poor predictor of drug safety, organ-on-chip devices ignore the power of AI/ML and rely mainly on manual data. While innovators continue to make significant strides in other stages of the drug discovery and development process – from determining a drug candidate’s mechanism of action or identifying new drug targets, to designing new molecules and their validation – the clinical prediction challenge remains unsolved and has become recognized as a primary focus for the industry.
According to Prof. Robert Langer, Co-founder of Moderna and former Chair of the FDA’s Science Board, “We are at the tipping point of the modernization of drug discovery. Predicting clinical safety is of huge value to pharmaceutical companies and the health of society at large.”
PATIENTS-ON-A-CHIP – THE NEXT ERA OF DRUG DISCOVERY
The true tipping point will come from the seamless integration of maturing innovations, including AI, patient-on-a-chip models, stem-cell science, and nano-sensing. To effectively predict human efficacy and safety, we need a completely new path that combines advanced technologies into one platform and removes the current faulty, time-intensive reliance on mice from the equation.
Patient-on-a-chip models, also referred to as multi-organ-on-chip models, will play a central role. Patient-on-a-chip models are now being created in which multiple miniaturized organs are interconnected by a blood-like circulation system. These models have demonstrated superior recapitulation of drug systemic effect and unprecedented PK/PD assessment in synthetic in vitro systems.2,6,8 Offering a remarkable breakthrough, Donald Ingberg’s work at Wyss Institute demonstrated how interconnecting several miniaturized organs produces unprecedented, accurate predictions of PK/PD for several toxic drugs that were missed by animal model testing in some cases.16 While this example linked multiple organ-chips with external pipes and pumps rather than relying on one, multi-organ chip, some systems are being developed with multiple organs on the same chip. But while multiple-interconnected-organs-on-chip systems offer a much higher level of data safety predictiveness, these systems were previously low-throughput and not easily scalable.8,11
Unlocking the full power of patient-on-a-chip technology requires an integrated approach. Other elements will be key to improving data safety predictiveness and accurately simulating a real human body reaction to drugs through patient-on-a-chip models, such as: real-time nano-sensing, high-throughput multi-OoC, and genomic diversity. Using next-generation, high-throughput patient-on-a-chip technology that leverages multiple, interconnected, miniaturized organs-on-a-chip to test thousands of known drugs, BioAI platforms can recapitulate their systemic effect and metabolism in the human body. As an example, each drug tested must first be metabolized by a miniaturized human liver, its metabolites then must interact with a miniaturized human blood-brain-barrier, and finally select chemicals would interact with a miniaturized human brain.
The platform must be able to run thousands, and ultimately millions, of experiments accurately and inexpensively. Miniaturization and advanced microfluidics will be key in addressing this challenge as making a significantly smaller scale patient-on-a-chip will reduce media volume, eliminate external pumps and tubes, reduce costs, and improve measurement sensitivity. Nano-sensing will also provide real-time measurements of metabolites and other biomarkers in the miniaturized tissues, creating time-series data for each of the metabolites monitored (such as oxygen, glucose, lactate) by documenting the response of various miniaturized organs to each administered drug. However, the nano-sensing measurements must be low-cost, highly sensitive, and create zero disturbance to accurately train the ML engine. Integrating nano-sensing technology with precision robotics and architecture miniaturization now make it possible to address these challenges – allowing high-throughput “dip-in” nano-sensing into the chamber housing the miniaturized organ to take multiple measurements over time without extracting any samples or affecting the concentration of metabolites in the well. Capturing microscopy images, such as fluorescent laser confocal microscopy, can monitor intracellular co-localization, in situ hybridization and changes in cellular morphology. Such nano-sensing capabilities increase the potential for more powerful organ-on-chip platforms, that harness real-time monitoring data, rather than relying on limited end-point data.
Stem-Cell Genomic Diversity to test known drugs on thousands of genomically diverse patients-on-a-chip creates a clinical-trial-on-a-chip by capturing the spectrum of diverse patient responses to a drug. Recent advances in stem cell automation technologies make this possible by rapidly creating hundreds of iPSCs relatively inexpensively from simple blood samples and then biologically programing each iPSC into different types of miniaturized organs (liver, brain, etc) on a chip.17 This allows the ML platform to learn not only to predict if a drug is generally safe, but also for whom is it safe – offering tremendous personalized-medicine ramifications in optimizing clinical trials, repurposing existing drugs and salvaging failed drugs.
When machine learning is at the center of an integrated Bio-AI prediction approach, it becomes fundamentally different from traditional biology and organ-on-chip approaches. The ML determines if a drug candidate will work safely in the human body. While patients-on-chip, nano-sensing, and stem cell genomic diversity all generate massive amounts of highly predictive drug safety data, the Bio-AI prediction approach does not rely on these components to directly detect the safety of a drug. Rather, the massive data they generate is used to train the ML, and based on that training, the ML can then predict drug safety – making it significantly different from traditional organ-on-chip approaches in both the data it uses and in how it uses this data. True Bio-AI platforms rely on thousands of experiments that generate massive, real-time time-series data, which is then used to train the ML. This approach harnesses the tremendous power of AI to predict drug safety.
Leading innovation in this field, Quris has developed a proprietary high-throughput patient-on-chip platform that integrates real-time nano-sensing to test thousands of known drugs, both safe and toxic, and then train the ML on the data generated, to better predict drug safety. With the scientific leadership of industry visionaries, including Nobel Laureate Prof. Aaron Ciechanover and Prof. Robert S. Langer, Quris has developed and proven a radically novel approach to drug development. The Quris BioAI platform (with 18 granted and pending patents) is the first AI clinical-prediction platform that simulates clinical trials and a real human body’s reaction to drugs by leveraging a patented patient-on-chip system through the use of stem-cell-derived tissue and AI to effectively predict drug toxicity. Our exclusive collaboration with the New York Stem Cell Foundation, a world leader in stem-cell automation, will allow us to rapidly run experiments on miniaturized organs derived from hundreds of proprietary, genomically diverse stem-cell lines that train our Bio-AI platform to discern personalized drug safety.17
Technology advances have finally aligned to allow a massive transformation in drug development speed, safety, and cost. The next decade will bring an explosion of drug innovation as regulations and the industry take note.
REFERENCES
- DiMasi JA, Grabowski HG. Economics of New Oncology Drug Development. J Clin Oncol 2007;25:209–16. https://doi.org/10.1200/JCO.2006.09.0803.
- DiMasi JA, Feldman L, Seckler A, Wilson A. Trends in Risks Associated With New Drug Development: Success Rates for Investigational Drugs. Clin Pharmacol Ther 2010;87:272–7. https://doi.org/10.1038/clpt.2009.295.
- The cost of opportunity – Gupta Strategists – Gupta Strategists – EN n.d. https://gupta-strategists.nl/en/research/the-cost-of-opportunity (accessed August 8, 2022).
- Wouters OJ, McKee M, Luyten J. Estimated Research and Development Investment Needed to Bring a New Medicine to Market, 2009-2018. JAMA 2020;323:844. https://doi.org/10.1001/jama.2020.1166.
- Mullard A. 2021 FDA approvals. Nat Rev Drug Discov 2022;21:83–8. https://doi.org/10.1038/d41573-022-00001-9.
- Brown DG, Wobst HJ, Kapoor A, Kenna LA, Southall N. Clinical development times for innovative drugs. Nat Rev Drug Discov 2021. https://doi.org/10.1038/d41573-021-00190-9.
- Low LA, Mummery C, Berridge BR, Austin CP, Tagle DA. Organs-on-chips: into the next decade. Nat Rev Drug Discov 2021;20:345–61. https://doi.org/10.1038/s41573-020-0079-3.
- Herland A, Maoz BM, Das D, Somayaji MR, Prantil-Baun R, Novak R, et al. Quantitative prediction of human pharmacokinetic responses to drugs via fluidically coupled vascularized organ chips. Nat Biomed Eng 2020;4:421–36. https://doi.org/10.1038/s41551-019-0498-9.
- Kimura H, Sakai Y, Fujii T. Organ/body-on-a-chip based on microfluidic technology for drug discovery. Drug Metab Pharmacokinet 2018;33:43–8. https://doi.org/10.1016/j.dmpk.2017.11.003.
- Bailey J, Thew M, Balls M. An Analysis of the Use of Animal Models in Predicting Human Toxicology and Drug Safety. Altern to Lab Anim 2014;42:181–99. https://doi.org/10.1177/026119291404200306.
- Cecen B, Karavasili C, Nazir M, Bhusal A, Dogan E, Shahriyari F, et al. Multi-Organs-on-Chips for Testing Small-Molecule Drugs: Challenges and Perspectives. Pharmaceutics 2021;13:1657. https://doi.org/10.3390/pharmaceutics13101657.
- Cook D, Brown D, Alexander R, March R, Morgan P, Satterthwaite G, et al. Lessons learned from the fate of AstraZeneca’s drug pipeline: a five-dimensional framework. Nat Rev Drug Discov 2014;13:419–31. https://doi.org/10.1038/nrd4309.
- Vernetti LA, Senutovitch N, Boltz R, DeBiasio R, Ying Shun T, Gough A, et al. A human liver microphysiology platform for investigating physiology, drug safety, and disease models. Exp Biol Med 2016;241:101–14. https://doi.org/10.1177/1535370215592121.
- Li X, George SM, Vernetti L, Gough AH, Taylor DL. A glass-based, continuously zonated and vascularized human liver acinus microphysiological system (vLAMPS) designed for experimental modeling of diseases and ADME/TOX. Lab Chip 2018;18:2614–31. https://doi.org/10.1039/C8LC00418H.
- Novak R, Ingram M, Marquez S, Das D, Delahanty A, Herland A, et al. Robotic fluidic coupling and interrogation of multiple vascularized organ chips. Nat Biomed Eng 2020 44 2020;4:407–20. https://doi.org/10.1038/s41551-019-0497-x.
- Brown DG, Wobst HJ. A Decade of FDA-Approved Drugs (2010–2019): Trends and Future Directions. J Med Chem 2021;64:2312–38. https://doi.org/10.1021/acs.jmedchem.0c01516.
- Paull D, Sevilla A, Zhou H, Hahn AK, Kim H, Napolitano C, et al. Automated, high-throughput derivation, characterization and differentiation of induced pluripotent stem cells. Nat Methods 2015;12:885–92. https://doi.org/10.1038/NMETH.3507.
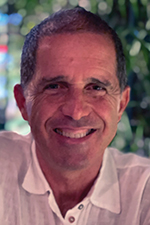
Dr. Isaac Bentwich is the Founder and CEO of Quris. Prior to Quris, he founded and led three bio-AI technology companies, each of which led revolutions in medicine, genomics, agriculture, and conservation. He is a physician and entrepreneur with a passion for leading interdisciplinary teams of scientists and technologists to tackle impactful challenges in the intersection between machine learning and life sciences; and to leverage and commercialize the resulting solutions. One of the companies he founded was Rosetta Genomics (NASDAQ:ROSG), which analyzed the human genome. He led the team at Rosetta Genomics in the discovery of hundreds of novel genes, more so than all the universities in the world combined, and delivered novel cancer diagnostics based on these genes. Its subsidiary, Rosetta Green (TASE:RSTG), was acquired by Monsanto for $35M. Under his leadership, team members at Rosetta went on to lead artificial intelligence at IBM, Google, and Microsoft. Now, at Quris, he and his team are using a similar bio-AI approach to disrupt the drug development process.
Total Page Views: 2902