Issue:April 2020
NATURAL LANGUAGE PROCESSING - How Life Sciences Companies Are Leveraging NLP From Molecule to Market
INTRODUCTION
Finding the right flavor of artificial intelligence to bring value to your organization is fraught with obstacles: what tool to use, what vendor to partner with, what application will most benefit from AI? One type of AI has already been bringing active value to pharma and healthcare organizations for a couple of decades – natural language processing (NLP) for text analytics. NLP text mining can be used to extract the key information from unstructured text, rapidly and effectively, to provide decision support from molecule to market. The following discusses some of the challenges facing pharma researchers and executives; the benefit NLP can bring; and some specific customer-use cases (covering patent landscaping, gene-disease associations, access to safety silos, and more).
CHALLENGES IN LIFE SCIENCE ORGANIZATIONS FOR ACCESS TO UNSTRUCTURED DATA
In the life sciences industry’s pipeline from drug discovery through development and into delivery, insight is needed at every stage, to answer questions, get through gates, or achieve milestones.
For example, early on in target discovery, researchers need to search the biomedical literature for specific genes involved in their therapeutic area of interest. Alternatively, they might want to search patent literature to understand the landscape around specific technologies, or to understand the competitive whitespace for certain disease targets. Or perhaps pharmaceutical executives want to find the optimal sites for a Phase 1 or Phase 3 clinical study; or might want to know more about patient-reported-outcomes for a particular product by searching Twitter, or voice of the customer feeds.
Answers to all these questions support business and healthcare decisions, and it is imperative that pharmaceutical companies employ the best possible view of data to generate insights. However, up to 80% of healthcare data is stored in an unstructured format, making it difficult to access and analyze, which often prevents scientists, researchers, and clinicians from leveraging the best possible information when making decisions.
To overcome the limitations of unstructured data, many of the leading life sciences companies have turned to natural language processing-based text mining. Among the key benefits of text mining with NLP is that, unlike standard keyword search that retrieves documents based on keywords that users must then read, NLP in essence reads the documents for users and identifies relevant facts and relationships. NLP extracts those facts and relationships in a structured format that enables review and fast analysis, connecting facts to synthesize knowledge and create actionable insights.
WHY NLP FOR TEXT MINING?
Historically, researchers have had little choice but to manually wade through free text, reading documents and creating summaries and analyses on their own. However, as the volume of healthcare data increases both in substance and format, this approach has become less viable. As a result, researchers need text analytics tools to make sense of this vast amount of information, and to uncover key facts and relationships that can provide answers to their questions.
NLP-based text analysis consists of several processes, including information retrieval, information extraction, lexical and semantic analysis, pattern recognition, tagging and annotation, and data mining techniques, such as association analysis and visualization. The overarching goal is, essentially, to turn text into data for analysis and insights, via application of NLP and analytical methods.
NLP enables a mapping from words in textual data and documents to meaning in a structured format for actionable decision support. NLP understands the grammar of a sentence and can identify nouns, verbs, the start and end of phrases and sentences, and more.
A key concept associated with text mining is ontologies, which are used to categorize similar things, group them together, and provide synonyms for concepts. For example, cancer, carcinoma, and neoplasm all refer to the same concept, so grouping this data together enables researchers to generate much more comprehensive data searches.
NLP-based text mining can be used across virtually any type of textual document, whether it’s scientific literature, patient literature, internal safety reports, drug labels, clinical trial data, social media, or electronic health records. By using NLP, researchers can transform their decision-making: from a document-centric view of finding documents and reading them, to a data-centric view of uncovering new insights from previously hidden relationships.
From molecule to market, life sciences companies have used NLP-based text mining to transform texts for decision support in multiple areas, including gene disease mapping, target selection, biomarker discovery or safety, right through to post-market activities, such as pharmacovigilance and competitive intelligence. The six use cases further on will give a flavor of these applications and the benefits NLP brings.
REAL-WORLD PHARMA USE CASES OF NLP
Pfizer: Effective Search for Patent Landscaping & Competitive Intelligence
Patent literature can provide a valuable competitive edge for pharmaceutical researchers by providing the first mention of critical data for novel drug targets, novel chemicals, compounds, and where competitors are working in specific disease areas. The problem is that patent literature documents are notoriously hard to search, often using obtuse and confusing language.
Pfizer observed that doing manual review and search of patents to find targets being researched by competitors in three therapeutic areas would require 50 full-time-equivalent days – a significant amount of manual effort. In response, Pfizer leveraged NLP text mining to build an automatic workflow to extract four main entities: target, indication, invention type, and organization. This data set is updated weekly across three of the major patent registries (WIPO, USPTO, EPO). The weekly data uploads go into a database that has a visual interface for business intelligence. The workflow improved recall tenfold over what Pfizer achieved with manual review, and the precision is equally strong.
Pfizer found that this integrated, automatic process significantly reduced the resources required to keep researchers and decision-makers up-to-date, and, also significantly increased the comprehensiveness of the data they were reviewing. Further, because the dashboards are so easy to interpret, the solution decreases the time to new insights, and broadens the value of patent data across the team.
Sanofi: Text Mining for HLA Allele Disease Associations
Sanofi has a significant focus on multiple sclerosis. In one project, Sanofi researchers sought to better understand what biomarkers might be useful in precision medicine research; so wanted to annotate the output of next-generation sequence (NGS) pipelines with the most up-to-date information from the literature. Particularly, they wanted to find any associations possible between HLA alleles and haplotypes and autoimmune diseases.
To address this, Sanofi employed NLP to mine literature sources to find HLA alleles and haplotypes and their relationships with diseases and drug sensitivity. They developed a suite of NLP queries that identified a wide range of HLA alleles, relationships, and diseases from abstracts or full-text articles. This strategy enabled the team to quickly find over 50 associations; both 22 associations already highlighted in a key review paper and an additional 33 disease- and drug-sensitivity associations from across the literature landscape that hadn’t been curated. NLP enabled the Sanofi team to standardize the information for integration into an internal knowledge base with a dashboard for broad use across the entire team. This provides Sanofi with a broader and more comprehensive knowledge base from which they can now confidently explore potential new biomarkers.
Merck: Preclinical Safety Data From Documentum Study Reports
Safety assessment during drug discovery through clinical development and into post-market surveillance and pharmacovigilance is essential. At all stages, project teams need the most comprehensive view of relevant data – ideally, both internal and external.
Pharma and biotech companies spend hundreds of thousands of dollars on preclinical safety studies, but the final reports often are stored in secure document repositories that are not easy to search, making it difficult for researchers to access the high-value information in these legacy documents. To capture key findings from safety assessment reports, Merck’s Safety Assessment and Laboratory Animals Resources (SALAR) group created an NLP workflow to extract key information from their safety report repository held in Documentum.
New reports are added to Documentum on a regular basis, and Merck’s NLP workflow analyzes them to pull out metadata on species, study duration, compounds, and other information. Notably, this workflow focuses on the interpreted results sections of these reports because it is the portion that includes expert conclusions on histopathology findings and adverse events, as distinct from any mentions of these in other sections (eg, methodology sections).
These new insights have enabled Merck to alleviate concerns in instances in which preclinical observations have been found not to be human-relevant, and also impacted on reducing late-stage failure. By better understanding the context of broad historical data, the company can better assess its current, active pipeline.
Eli Lilly: Mining ClinicalTrials.gov for Clinical Trial Intelligence
A number of life sciences companies employ NLP text mining to uncover information from clinical trials databases, such as ClinicalTrials.gov, TrialTrove, or Pharma Projects. Though these pipeline databases often store valuable information, it is difficult to query the unstructured text in those documents or use ontologies for better search and recall.
NLP helps researchers rapidly identify, extract, synthesize, and analyze relevant information such as clinical trial site, selection criteria, study characteristics, patient numbers, and characteristics that would not be possible using other approaches. Eli Lilly’s competitive intelligence clinical group needed to assess the landscape of Phase 1 and 2 clinical trials that were testing two or three drugs in combination for autoimmune diseases. Manually, they had found only seven trials, and had decided this approach required too much effort. However, with a relatively straightforward NLP query over ClinicalTrials.gov, Lilly was able to find an additional 300 trials very quickly. In addition, the NLP query extracted the drug names, specific autoimmune disease, the phase of the trial, and the sponsors; all normalized and structured for rapid effective review.
Novo Nordisk: Actionable Insights From Real-World Data
There’s a real buzz right now about real-world data (RWD). In pharma and healthcare, understanding the real-world impact of therapies on patients is critical. RWD can shed light on real-world clinical effectiveness and on safety profiles of products across a broad patient community; as well as to assess patient-reported outcomes and to understand product reputation management. However, many RWD sources contain unstructured text, which prevents easy analysis. Text analytics is essential to unlock the value from sources of RWD, such as social media, EHRs, clinical guidelines, and customer call transcripts.
Novo Nordisk wanted to identify healthcare market trends and detect patterns from three disparate RWD sources: call center feeds, medical information requests, and conversations with healthcare providers. The company was already analyzing this data, but via an inefficient and labor-intensive process in which vendors did manual extraction and scanning.
To solve the problem, Novo Nordisk built an NLP workflow to transform RWD from the three sources to drive a medical and patient dashboard, making medical and patient data actionable across its global workforce. Novo Nordisk hosts this information in an Amazon Web Services data lake, running NLP queries to pull out key topics and trends, and providing visual dashboards using Tableau.
The new workflow replaced the need for manual scanning, saving the company approximately two full-time employees per year. Novo Nordisk also reduced spend on external vendor report generation, has automated evidence-based insights generation, and significantly broadened access to these insights across its team.
Bristol-Myers Squibb: Text Mining EMRs for Patient Stratification of Heart Failure Risk
All pharmaceutical companies have a strong interest in understanding how different therapies and drugs are being used and applied. More specifically, Bristol-Myers Squibb (BMS) wanted to understand more about patient stratification for heart failure risk. Heart failure patients typically exhibit high levels of clinical heterogeneity, which is problematic for treatment and for risk stratification. BMS researches believed that if they could acquire a deeper understanding of the clinical characteristics of these patients, they could potentially understand how best to treat different patients or populations.
To that end, researchers obtained electronic health record and imaging data for about 900 patients, and used NLP to write queries, extract and normalize approximately 40 different variables around patient demographics, clinical outcomes, clinical phenotypes, and other variables such as ejection fraction and left ventricular mass.
With advanced statistical clustering, BMS researchers identified four classes of patients with discrete clinical and echocardiographic characteristics that showed significant differences in 1- and 2-year mortality and also 1-year hospitalizations. By better understanding how to stratify patient populations for heart failure, BMS has unlocked insights on that can potentially improve the design of clinical trials, identify unmet needs, and develop better therapeutics.
DRIVING COMMERCIAL EXCELLENCE THROUGH DATA TRANSFORMATION & ANALYTICS
At every stage of the drug development pipeline from molecule to market, data can provide the competitive advantage that determines the difference between success and failure. The problem for many pharmaceutical companies is that 80% of that data is unstructured and difficult to access and investigate for insights.
NLP-based text mining unlocks the hidden value in data sources as disparate as patents, scientific reports, patient literature, electronic health records, customer call transcripts, and social media. For life sciences companies, higher quality data means improved gene disease mapping, target selection, biomarker discovery, and competitive intelligence – boosting pharmaceutical innovation and enhancing commercial value.
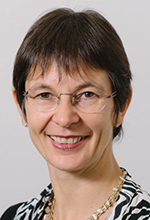
Dr. Jane Reed is Director, Life Sciences at Linguamatics, an IQVIA company. She is responsible for developing the strategic vision for Linguamatics’ growing product portfolio and business development in the life science domain. Dr. Reed has extensive experience in life sciences informatics, having worked for more than 15 years in vendor companies supplying data products, data integration and analysis, and consultancy to pharma and biotech, including roles at Instem, BioWisdom, Incyte, and Hexagen. She earned her PhD in Physiology from the University of Birmingham and her MA in Natural Sciences from the University of Cambridge.
Total Page Views: 9659