Issue:April 2024
DRUG DISCOVERY - Addressing Challenges in Drug Discovery: DEL Screening Applications
INTRODUCTION
DNA-encoded library (DEL) screening has emerged as a well-established technique in early stage drug discovery. Many large pharmaceutical companies (and a handful of service providers) operate DEL platforms, and the number of published DEL successes is growing year over year.
One reason for DEL’s growing popularity is the fact it is well-suited for solving key problems in contemporary drug discovery. The following will explore DEL’s application in three areas of drug discovery that have received much attention throughout the past 5 years: Induced Proximity, Covalent Drug Discovery, and Machine Learning.
INDUCED PROXIMITY
The use of small molecules to induce the proximity of protein pairs represents a revolutionary new modality in drug discovery. This approach allows researchers to harness cellular machinery to modulate protein (or RNA) functions, effectively mitigating disease. The first example of induced proximity to gain attention is Targeted Protein Degradation (TPD).1 In TPD, the goal is to induce proximity between a disease-causative protein (often referred to as a Protein of Interest or POI) and an E3 ligase. By doing so, the POI is drawn into the E3’s associated ubiquitinylation machinery and thereby labeled for proteasomal destruction. As such, TPD does more than just inhibit disease-associated POIs; it eliminates them altogether.
To induce proximity between these proteins with a small molecule, the molecule must have binding affinity for both proteins. This is commonly achieved by creating so-called “chimerical” compounds, in which binding moieties for both the E3 and the POI are connected by a chemical linker. This modular approach simplifies the design of TPD agents. Binders for E3s are documented in the literature, centering the main workflow on discovering POI binders, linking them with known E3 ligands, and optimizing for degradation efficiency and ADME. It is within this workflow that DEL really shines.
At its heart, DEL screening is a binding experiment. Compounds displaying affinity for the POI are isolated from the bulk library and identified through DNA sequencing. Because TPD requires only affinity (not functional effects like inhibition), DEL hits can be seamlessly integrated into a chimeric molecule and assessed for degradation ability. An efficient workflow can be implemented wherein DEL hits are re-synthesized off-DNA with a reactive linker in place of the DNA attachment. These hits can then be coupled with commercially available E3 ligands featuring complementary reactive handles. This facilitates swift array synthesis, enabling the generation of tens or even hundreds of candidate degraders that explore diverse combinations of E3 ligands, POI binders, and linkers. These candidate degraders can be directly tested for their protein knockdown effects.
A publication describing this workflow appeared in 2020 (Figure 1).2 Ligands for the oncology target estrogen receptor α (Erα) were discovered by DEL screening, resynthesized with reactive linkers, and linked to E3 ligands. It was quickly determined that the E3 VHL was the optimal partner in this system, and the first tested compound was shown to degrade Erα with a potency of 38 nM.
Induced proximity approaches extend beyond just TPD. Newer modalities, such as AUTACs, RIBOTACs, DUBTACs, and many others rely on different cellular mechanisms, such as autophagy or RNA degradation. These approaches expand the toolkit of induced proximity through targeting RNA, extending the lifetime of proteins, or degrading proteins by non-E3-dependent mechanisms. But in all cases, high-quality, linkable ligands will be required, making DEL a powerful enabling technology as these modalities mature.
A new frontier in induced proximity is the emergence of molecular glues, non-chimerical compounds that can induce protein interactions. Glues could have significant advantages over the modalities previously discussed because they should have better developability properties than chimerical compounds (eg, lower molecular weight, fewer rotatable bonds, improved metabolic stability). The search for novel glues is daunting; rather than finding the proverbial needle in the haystack, glue discovery is like searching a mountain of haystacks. Fortunately, the size and scope of DEL libraries indicate that the direct discovery of glues should be possible. DEL glue selection proof-of-concept experiments are ongoing at several labs and reports are on the horizon.
COVALENT DRUG DISCOVERY
Another emerging (or re-emerging) modality that DEL will accelerate is covalent drug discovery. These drugs form covalent associations with their biological targets — typically proteins via their reactive amino acid side chains (eg, cysteine). The bonds are typically irreversible, meaning the drug compound becomes permanently bound to the target. This approach has obvious advantages in terms of potency, duration of action, and pharmacokinetics. But despite these advantages, covalent drug discovery attracted little attention for many years. This reluctance was based on the perception that covalent drugs had a higher risk of off-target activity and toxicity than conventional drugs (it should be noted that aspirin is a covalent drug).
Covalent drug discovery has generated renewed interest in recent years. For example, in the early 2010s, several companies brought cysteine-targeted covalent kinase inhibitors to market. Additionally, chemoproteomics researchers showed that the reactivity of protein side chains could vary widely based on the local environment, supporting the possibility of selective targeting. Lastly, new reactive groups were introduced that went beyond cysteine-targeting, making it possible to target other amino acids, such as serine and lysine.
With this renewed interest, the question then arose: how to best discover covalent drugs? Screening large collections of reactive compounds appears to be impractical because of the likelihood of limited shelf-stability (compared to non-reactive compounds, as typically utilized in high-throughput screening). Two approaches that bypass this problem, however, have shown success. The first is the re-purposing of non-covalent compounds. In this process, researchers take a pre- existing non-covalent ligand, and using structural information about nearby side chains in the binding site, append it with a reactive group to target a specific side chain. The BTK inhibitor ibrutinib is an example of this approach.3 The second method is to screen small numbers of covalent fragments and elaborate the hits into suitably potent drug candidates. This process was exemplified by the discovery of KRas G12C inhibitors, all based on a small reactive fragment (discovered by professor Shokat at University of California San Francisco).4
DEL screening represents a new and powerful approach to covalent drug discovery. Encoded libraries containing reactive groups can be prepared just like conventional DELs. Installation of the reactive groups may be conducted immediately prior to screening to minimize storage time and possible decomposition. These reactive library members are not fragments; rather, they are fully elaborated lead-like or drug-like compounds. In the screening experiment, the library is exposed to the desired protein target, and only those compounds that are permanently attached to the protein (and survive extensive washing) are sequenced and analyzed. Because DEL analysis is based on the differential enrichment of hit compounds over other library members, reactivity-based promiscuity is addressed immediately in the screening experiment. Thus, we can see that DEL directly addresses a major challenge faced in covalent drug discovery.
One published example of covalent DEL screening illustrates these advantages.5 The kinase BTK was screened using DELs that contained several different reactive groups. The resulting hits were structurally unrelated to any known non-covalent BTK inhibitors, although they did share a substructure with the DEL-derived BTK ligand reported earlier by the same group (Figure 2). Furthermore, the compounds were exquisitely selective, showing once again that covalent drugs need not be promiscuous.
MACHINE LEARNING
The use of machine learning (ML) in drug discovery has attracted considerable interest throughout the past 5 years. ML holds the promise of taming the incredible volume and diversity of data produced by drug discovery research, yielding novel insights. Medicinal chemistry has been described as the ultimate multiparameter optimization problem, in which numerous factors like potency, stability, permeability, toxicity, solubility, and others must be honed simultaneously. ML is well-suited to addressing the multiparameter problem, thereby accelerating the discovery of new drug candidates.
There is a paradox, however, in the embrace of ML in drug discovery. The highest-value, most novel drug targets are those that have the least amount of historical data, especially chemical data. New targets emerge in the literature, indicated by proteomic or genomic data as being involved in a particular disease state. Throughout the ensuing years, as hit generation and lead optimization campaigns progress, a chemical data resource for the target gradually accumulates. This means that for the highest value targets, it can take years to decades to amass sufficient chemical data to allow ML to add value.
DEL short-circuits this problem. The DEL screening experiment relies on DNA sequencing to characterize the compound structures bound to the target. Because modern techniques can generate millions of individual sequences for a modest cost, DEL can produce millions of chemical data points very quickly. This quantity of chemical data surpasses that which can be generated by any other screening modality, and it is sufficient in scope and depth to bring ML to bear. Essentially, DEL screening rapidly generates a quantity of data that would take other modalities years to produce. Thus, even unprecedented targets can be swiftly enabled with a DEL screening was used to generate such a model, and one of the first known DCAF1 ligand was found in a commercial virtual library (Figure 3) data resource.
Recent peer-reviewed publications validate this proposition. In a proof-of-concept experiment reported in 2020, X-Chem and Google collaborated to apply ML to DEL datasets across a small set of pharmaceutically relevant targets.5 The resulting ML models were adept at predicting new binders from untested virtual libraries. More recently, this method has moved beyond proof-of-concept and demonstrated successful hit generation for targets for which no binders had been previously reported. In early 2023, Li and colleagues at the University of Toronto and the Structural Genomics Consortium (SGC) demonstrated the DEL and ML workflow in action, focusing on the novel target DCAF1.6 At the time of publication, no ligands for DCAF1 had been reported. Consequently, there was no corpus of historical data to rely on for ML model training. DEL screening was used to generate such a model, and one of the first known DCAF1 ligand was found in a commercial virtual library (Figure 3).
A second example of this workflow was recently disclosed by workers from the same institution. In this case, the target was WDR91, another novel target for which no ligands were known.7 Once again, the DEL and ML workflow was able to generate the first known ligands for WDR91. Following from these successes, X-Chem and the SGC recently announced a collaboration aimed at bringing this technique to scale, using DEL and ML to find probes for the drug targets of the future.8
As this methodology continues to develop, it is likely to move beyond early adopters in academia and begin to find purchase in biotech and pharma. An important question remains about the breadth of applicability and the critical mass of libraries and screening data needed to build effective models. We eagerly anticipate the development of this field in the coming years as we tackle the difficult targets of the future.
SUMMARY
As always, new opportunities and challenges abound in drug discovery. New modalities, such as induced proximity, promise to unlock new vistas in how we attack disease. Innovations breathe new life into older techniques like covalent drug discovery. And advances in computational power allow ML to illuminate hidden patterns in vast data repositories. In all three cases, the power of DNA-encoded library screening will drive and enable these advancements to create the drugs of the future, fulfilling the unmet medical needs of patients around the world.
REFERENCES
- Békés, M., Langley, D.R., & Crews, C.M. PROTAC targeted protein degraders: the past is prologue. Nat Rev Drug Discov 21, 181–200 (2022) https://doi.org/10.1038/s41573-021-00371-6
- Disch, J.S., et al. J. Med. Chem. Bispecific Estrogen Receptor α Degraders Incorporating Novel Binders Identified Using DNA-Encoded Chemical Library Screening. 2021, 64, 8, 5049–5066 https://doi.org/10.1021/acs.jmedchem.1c00127
- Ibrutinib (2023, September 12) In Wikipedia. https://en.wikipedia.org/wiki/Ibrutinib
- Cully, M. Undruggable? Inconceivable. Nature Briefing (2020). https://www.nature.com/articles/d42859-020-00080-x
- McCloskey, K., et al. J. Med. Chem. Machine Learning on DNA-Encoded Libraries: A New Paradigm for Hit Finding. 2020, 63, 16, 8857–8866 https://doi.org/10.1021/acs.jmedchem.0c00452
- Li, A.S.M., et al. J. Med. Chem. Discovery of Nanomolar DCAF1 Small Molecule Ligands, 2023, 66, 7, 5041–5060 https://doi.org/10.1021/acs.jmedchem.2c02132
- Ahmad, S., et. al. J. Med. Chem. 2023, 66,23, 1605116061. Discovery of a First-in Class-Small-Molecule Ligand for WDR91 Using DNA-Encoded Chemical Library Selection Followed by Machine Learning. https://doi.org/10.1021/acs.jmedchem.3c01471
- X-Chem and Structural Genomics Consortium Enter Into Collaboration to Unlock the Human Proteome and Promote Open Science (2023, September 28). Press Release. X-Chem. https://www.x-chemrx.com/about/news/x-chem-and-structural-genomics-consortium-enter-into-collaboration-to-unlock-the-human-proteome-and-promote-open-science/.
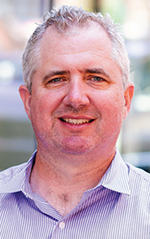
Dr. Matt Clark is a world-recognized innovator and leader in the DNA-encoded library (DEL) field. He was part of X-Chem’s founding team, served as VP of chemistry, SVP of research and CEO prior to his appointment to the CSO position. Under his scientific leadership, the company developed from a niche chemical discovery platform to a world-leading drug discovery engine serving the biopharma industry. Before joining X-Chem, he was director of chemistry at GlaxoSmithKline, where he led the group responsible for design and synthesis of early-iteration DELs. He began his professional career at Praecis Pharmaceuticals, where he played a key role in the early development and implementation of technologies that would become the basis for DEL. He is a thought leader in the DEL space, with numerous patents and key DEL publications to his name. He earned his B.S. in biochemistry from the University of California, San Diego, his PhD in Chemistry from Cornell University and conducted post-doctoral studies at the Massachusetts Institute of Technology.
Total Page Views: 3270