Issue:November/December 2023
CLOUD COMPUTING - Revolutionizing Antibody Discovery: The Role of Cloud Computing
INTRODUCTION
Antibody therapies are an important class of drugs that have exhibited outstanding efficacy and safety in the treatment of many major diseases, including cancers, auto-immune, hematological, and infectious diseases, including COVID-19. Considerable progress has been made in the global research and development of antibody therapies in the past decade.
The antibody market is also the fastest growing class of therapeutics, with 9 of the top 20 best-selling drugs, and is projected to grow from $178.50 billion in 2021 to $451.89 billion in 2028 at a CAGR of 14.1%.1,2 According to the Umabs-DB, 162 antibody therapies have been approved by at least one regulatory agency in the world, including 122 approvals in the US, followed by 114 in Europe, 82 in Japan, and 73 in China. While the US and Europe have led the way in antibody drug discovery for decades, Japan and China have made significant strides in the past decade.3
Traditional methods of antibody discovery using random colony screening and low-throughput Sanger sequencing are inefficient and incomplete. Due to the inherent limited sampling in this approach, understanding the complete binding diversity is nearly impossible at a reasonable cost, which makes it difficult for many small biotech companies to compete. Further, such traditional approaches only capture a fraction of the diversity, lowering the potential to find antibodies with desired specificity or biophysical properties.
The advent of next-generation sequencing (NGS) and high-throughput computing have revolutionized antibody discovery, making it faster, more efficient, and cost-effective. Cloud computing has further improved this process by reducing overhead costs as well as significantly simplifying the process. The following discusses the role of cloud computing in antibody discovery, its benefits, and its potential applications in the field of biotechnology.
ANTIBODY DISCOVERY
Antibodies are essential tools in diagnostics, therapeutics, and research. Antibody discovery, most often using in vitro (phage or yeast) or in vivo (hybridoma) technologies, is a crucial step to identify leads that bind specifically to target proteins.
The massively parallel sequencing technology known as NGS includes several high-throughput approaches to DNA sequencing and provides extremely high throughput, scalability, and speed. NGS parallelization of sequencing reactions generates hundreds of megabases to gigabases of nucleotide sequence reads in a single instrument run. This has enabled a significant increase in available sequence data and fundamentally changed genome sequencing approaches in the biomedical sciences.4
Applying NGS to antibody discovery pipelines allows for more comprehensive coverage of output populations at substantially reduced costs. Further, advances in high-throughput computing and computational tools have simplified the handling of the large amount of data generated. This not only allows the expansion of diversity from any given selection campaign, but also reduces biases, such as favoring only the most abundant clones. The underlying statistics collected from NGS outputs enrich datasets, providing information that significantly improves ranking criteria for potential leads. Most importantly, the greater number of unique antibodies identified allows the use of unsupervised clustering to obtain diverse clones. This improves screening efficiency by eliminating redundancy arising from screening antibodies in the same cluster with similar binding properties.
The advent of NGS, high-throughput computing, and computational tools have elevated the need for cloud computing, before which companies had to rely upon the expertise of highly technical staff supported by computational overhead, making it challenging for organizations with fewer financial resources. Cloud computing involves the use of remote servers to store, manage, and process data, democratizing access to computational tools and computing power/compute resources across a broad spectrum of companies from small to larger biotech.
THE ROLE OF CLOUD COMPUTING IN ANTIBODY DISCOVERY
The emergence of cloud computing has revolutionized the field of biotechnology. In the case of antibody discovery, cloud computing enables the storage and analysis of large volume of data, particularly DNA sequencing data, leveraging sophisticated algorithms residing on remote servers, rather than on-premise. This allows screening of target antibody populations, derived from large antibody library selections or animal immunization, by rapidly performing complex calculations on massive amounts of data, making the process more efficient and cost-effective.
BENEFITS OF USING CLOUD COMPUTING IN ANTIBODY DISCOVERY
Other benefits to cloud computing are that it helps overcome any data sharing limitations, and empowers teams with easy access to software and computational resources. Data sharing, in turn, promotes collaboration and enables researchers to identify, visualize, and prioritize leads across distinct discovery campaigns through a cloud interface in real time.
Finally, as an increasing number of machine learning solutions are applied to the antibody space, the need to scale computational power poses a serious challenge. Before cloud computing, users were restricted to in-house hardware, requiring frequent updates and significant support from overhead informatic technical staff. Cloud computing reduces overhead and staffing needs by providing scalable access to computational power. Cloud-based antibody discovery platforms provide the flexibility to scale up or down depending on the needs of the project. Companies can easily access the computing resources they need to run their discovery programs, without having to invest in expensive hardware and infrastructure.
CLOUD COMPUTING BEST PRACTICES FOR ANTIBODY DISCOVERY
A key element of cloud computing best practices for antibody discovery includes benchmark datasets and validation studies. Benchmark datasets are compiled to compare the performance of a model against an industry standard, while validation studies test the performance of a particular tool against empirical performance metrics. This in turn allows for automating the workflows with tested parameters and simplifies the overall pipeline by uploading the sequencing data and inputting the desired number of leads. This creates a “plug and play” to run the calculation on the cloud. The best cloud computing platforms also provide adequate technical support and training, secure and reliable data management, and compatibility for various computation tools.
CASE STUDY: SUCCESSFUL APPLICATIONS OF CLOUD COMPUTING IN ANTIBODY DISCOVERY
Selection campaigns from the Specifica Generation 3 antibody platform were conducted against three SARS-CoV-2 targets. Unique NGS sequences identified by the AbXtract module in the cloud-native Orion® Antibody Discovery Suite were compared to random colony screening for the same selection outputs. Antibody sequences were clustered by unsupervised machine learning, and genes corresponding to representative cluster antibodies were synthesized, expressed as IgG and experimentally tested for epitope binning by surface plasmon resonance (SPR).
As expected, this study showed that antibodies within clusters recognized identical epitopes, while antibodies recognizing distinct epitopes belonged to distinct clusters. This study validated Specifica’s bioinformatics pipeline and clustering method to prioritize leads for experiments.
THE FUTURE PROMISE OF ANTIBODY DISCOVERY WITH CLOUD COMPUTING
The future of antibody discovery with cloud computing is promising, particularly by enabling the efficient deployment of artificial intelligence (AI) to this field at large scales. AI is composed of a wide variety of subfields, whereby machine learning, particularly deep learning (DL) and natural language processing (NLP), show great promise within the antibody discovery space.
Many recent advances in DL, particularly through the increasing use of transformers, are enhancing NLP capabilities within the antibody discovery space. Some active areas of AI in the antibody discovery space include: 1) de novo antibody design, 2) developing AI-enhanced library formats, 3) improving the probability of success of lead candidates from in-vitro discovery campaigns, 4) paratope and epitope prediction, 5) structural homology modeling and docking, and 6) identifying and ranking developability issues in antibodies.5-10
While many of these applications continue to show real improvements in antibody discovery, it is unclear whether de novo design, which holds the greatest potential to disrupt the field, will match the hype. For instance, a recent study on de novo design employed generative AI to produce a wide array of leads to a known binder, but still required construction of a library followed by the screening of leads.11 While the employment of AI for library construction is novel here, it begs the question of whether that is an improvement to existing capabilities or shows true cost savings over some of the latest discovery technologies.12
While we hold high hopes for de novo antibody lead generation, it will be important for these technologies to show clear improvements in time, cost, diversity, and developability over existing state-of-the-art display technologies.12 One limitation of the de novo effort may be the available datasets used to train AI models, which may fall short of comprehensively capturing the latent patterns within protein-protein interaction networks, understanding the biophysics driving these interactions, or the sequence or structural properties implicated in developability. Of course, this is not necessarily a limitation in AI, but high-throughput experimental technologies, cross-institutional data silos, and/or in silico modeling (homology models and docking), which may be required to enable these AI models to fulfill its promise, particularly in de novo design.
To overcome this limitation for effective AI training, not only large amounts of data but appropriate processing and storage requirements will be required. Cloud computing offers immediate solutions to these hurdles by allowing ready access to a vast computing network, which enables researchers to scale their needs to process these large datasets at the push of the button. Furthermore, cloud infrastructure allows for the testing of a large array of AI models and the fine-tuning of hyperparameters in a massively parallel fashion, enabling more automated selection of models and hyperparameters suited to a given task. Streamlining of these processes is a major advantage of cloud computing, ultimately facilitating better decisions on lead prioritization and reducing costs.
SUMMARY – THE IMPACT OF CLOUD COMPUTING ON THE FUTURE OF ANTIBODY DISCOVERY
Cloud computing has transformed the field of antibody discovery, making the process faster, more efficient, and cost-effective. The use of cloud computing tools and algorithms has enabled the rapid screening of large antibody libraries, leading to the identification of high-affinity antibodies with high specificity. Overall, antibody discovery is a complex process and leveraging cloud-based platforms provides a centralized location for researchers to store, share, and analyze data, facilitating collaboration and development of new antibody discovery methods.
REFERENCES
- Mullard A. FDA approves 100th monoclonal antibody product. Nat Rev Drug Discov. 2021;20(7):491-495. doi: 10.1038/d41573-021-00079-7.
- Monoclonal antibody therapy market size, share, & COVID-19 impact analysis, 2021-2028. Fortune Business Insights. August 2021. Available at: https://www.fortunebusinessinsights.com/monoclonal-antibody-therapy-market-102734.
- Antibody Therapy Database; 2023 UmabsDB. Available at: https://umabs.com/info/2c9f89307fe47598017fe98385610018.
- Tucker T, Marra M, Friedman JM (August 2009). Massively parallel sequencing: the next big thing in genetic medicine. Am J Hum Genet. 2009;85(2):142-154. doi: 10.1016/j.ajhg.2009.06.022.
- Hie, B.L., et al. Efficient evolution of human antibodies from general protein language models. Nat Biotechnol (2023). https://doi.org/10.1038/s41587-023-01763-2.
- Shuai, R.W., Ruffolo, J.A., Gray, J.J. Generative language modeling for antibody design. BioRxIV (2022). https://www.biorxiv.org/content/10.1101/2021.12.13.472419v2.
- Saksena, S.D., et al. Computational counterselection identifies nonspecific therapeutic biologic candidates. https://doi.org/10.1016/j.crmeth.2022.100254.
- Leem, J. et al. Deciphering the language of antibodies using self-supervised learning. Patterns (2022). https://doi.org/10.1016/j.patter.2022.100513.
- Brennan Abanades et al. ImmuneBuilder: Deep-Learning models for predicting the structures of immune proteins. BioRxIV (2022). 10.1101/2022.11.04.514231v1,
- Ameya Harmalkar et al. Toward generalizable prediction of antibody thermostability using machine learning on sequence and structure features, mAbs (2023). 10.1080/19420862.2022.2163584.
- Shanehsazzadeh, A et al. Unlocking de novo antibody design with generative artificial intelligence. BioRxIV (2023). https://doi.org/10.1101/2023.01.08.523187.
- Teixeira, A. et al. Drug-like antibodies with high affinity, diversity and developability directly from next generation antibody libraries. mAbs (2021). 10.1080/19420862.2021.1980942.
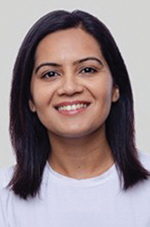
Dr. Suhani Nagpal is an Application Scientist at OpenEye, Cadence Molecular Sciences in Boston, MA. Leveraging her technical expertise, she provides scientific support for OpenEye’s applications and cloud-based software solutions in both antibody and small molecule drug discovery areas and works on services projects with customers. Prior to joining OpenEye, she earned her PhD in Bioengineering with a focus in computational biophysics at the University of California-Merced, where she explored the conformational rheostat mechanism in protein folding and binding and engineered a protein-based conformational transducer for biosensing.
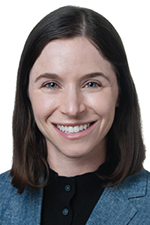
Dr. Laura Spector is a Bioinformatics Scientist at Specifica, a Q2 Solutions Company in Santa Fe, NM, where she interfaces between the Discovery and Bioinformatics teams to conduct next-generation sequencing and data analysis for antibody discovery campaigns. In developing new AbXtract workflows for OpenEye’s Orion Antibody Discovery Suite and validating them using in-house data, she contributes to tools that accelerate the identification of clonotypes of interest. Prior to joining Specifica, she earned her PhD in Genetics at Stanford University, where she studied genomic factors influencing a virus-mediated gene therapy platform.
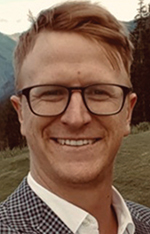
Dr. M. Frank Erasmus is Head of Bioinformatics at Specifica, a Q2 Solutions Company in Santa Fe, NM. He has more than 15 years of experience in antibody therapeutics, with several patents and publications contributing to the advancement of the field.
Total Page Views: 3518