Issue:January/February 2024
CLINICAL TRIALS - The Power of AI in Overcoming Patient Diversity Challenges
INTRODUCTION
Clinical trials continue to be a major stumbling block in the drug discovery and development life cycle. A stumbling block so overwhelming, current pharmaceutical practices do not even provide a 50% shot of success. In fact, clinical trial success rates do not even top 10%; there is an abysmal 90% failure rate for novel drugs in clinical trials, meaning only 1 out of every 10 drug candidates. There is no question the novel drugs that manage to work their way through to market make a critical impact, but those life-changing – and often life-saving – drugs are too few and far between.
The following explores why patient diversity is such a challenge for clinical trials, where that pain is often felt the most, some of the regulatory, technological, and industry changes already underway to solve the clinical trial gap, and where artificial intelligence (AI) changes the future of patient diversity.
THE ROOT ISSUE
Clinical trials serve as the primary pre-market evaluation step for novel drugs that have made it through the time- and cost-intensive preclinical phase. Unfortunately, the traditional drug development process puts novel drug candidates at a significant disadvantage before they even make it to clinical trials. Still overwhelmingly tied to the faulty animal/mouse model, the data used to advance novel drug candidates into the clinical trial phase relies on animal studies.
In the words of Nobel Laureate Aaron Ciechanover, MD, DSc, “One of the main problems in drug development is the model that we are using – the mouse. We are not mice, so what works in animal-based trials is not a proper indicator of what will work for people.”
Yet despite the incredible failure rate consistently demonstrating its limitations, animal models have reigned as the preclinical kings for 80+ years due to animal testing requirements mandated by outdated regulations. Even predictive models based on mice that show promise at predicting lethal doses of drugs struggle to effectively screen for likely side effects. Some studies have even compared the variations by animal species for predicting compounds’ metabolic behavior in humans. There are simply too many critical differences in the organ systems as well as the composition, expression, and catalytic activities of drug-metabolizing enzymes to effectively extrapolate data from animal models to humans.
THE PRIMARY PATIENT DIVERSITY PAIN POINT
For the drug candidates that make their way to trial, patient diversity is the primary pain point. And much like the limitations of relying on animal models in the preclinical phase, the diversity dilemma has been a decades-long issue. Early clinical trials often relied solely on white male participants, and the problem is not going away. Worse yet, this is a global issue; an in-depth study of trials across 29 countries between 1997 and 2014 found that participants were almost 90% white. Yet, racial/ethnic minorities are 1.5 to 2 times more likely than whites to suffer from major chronic diseases.
The dearth of diversity creates major gaps in the resulting clinical trial data as those trials typically fail to properly represent the individuals most affected by the specific disease or condition the drug was intended to treat. The groups who likely stood the best chance to benefit from the drug, whether by a combination of age, biological sex, race, sexual orientation, or other risk factors like medical history or environmental conditions, were woefully underrepresented in many trials. If a trial underrepresents certain groups by design or mere oversight, the resulting knowledge gap severely limits the ability to pinpoint optimal treatments, fully understand potential risks, and properly determine safe use cases.
Disappointingly, the disconnect between trial participants and future patient demographics still runs rampant today. Recent studies continue to confirm that white participants are overrepresented in all types of clinical trials, and the lack of diversity extends to sex, age, etc. as well (Figure 1). Despite decades of advancements in our understanding of the need for patient diversity in trials, advocacy efforts, and regulatory changes to try to make trial data more representative of the long-term treatment demographic, most clinical trials still fail to meet patient diversity demands.
According to Raeka Aiyar, PhD, of The NYSCF Research Institute, “Many minoritized populations have been excluded from research. And as a result, we have a biased understanding of disease and treatments that do not work for everyone who needs them. A black woman is 22% more likely to die from heart disease than a white woman, and 71% more likely to die from cervical cancer,” she continued. “Before COVID, less than 5% of NIH funded respiratory research reported inclusion of racial or ethnic minorities.”
When researchers actively try to recruit a more diverse trial set, they still often find members of minority groups – be it by age, race, ethnicity, or gender – are more reluctant to participate. Often it is driven by a lack of awareness in underinsured populations. Access, resource, and visibility limitations aside, fears of discrimination, unethical treatment, exploitation, job loss, or financial ramifications also contribute to inadequate participation. Regardless of the cause(s) of the continued lack of diversity in clinical trials, the ramifications are far-reaching and life-threatening as some treatments can be rendered ineffective or deadly when that demographic group is not included in trial data. Biological differences can change how patients will respond to certain drugs or therapies – making a treatment more or less effective, or even more or less toxic, for one group than another.
The absence of diverse research impacts patients and treatments in many ways; asthma is one easy-to-understand example. “In the Bronx, we talk about ‘ABCD’ being the priorities in terms of healthcare: asthma, blood pressure, cancer, and diabetes,” noted John Greally, PhD, DMed, Montefiore Hospital, Albert Einstein College of Medicine, in the same NYSCF webcast. “The treatment of asthma using the standard bronchodilators that relax the muscles of the airway and prevent spasm work much better in some ancestry than others. The non-responsiveness to albuterol, which is our first line treatment, is about 47% of people who identify as African American and 67% of Puerto Rican kids. It’s about 20% for people who are Northern European white. Unless we study [diverse groups], we’re not going to understand where we could be doing harm with these sorts of medications.”
Perhaps a silver lining for the future of drug research and development, the pandemic confirmed the need for clinical trial diversity and health equity, making it a medical and media priority. In response to the continued lack of diversity, the FDA issued draft guidance for the industry, focused on developing plans to enroll more participants from underrepresented racial and ethnic populations into US clinical trials. Adding to the regulatory push, the omnibus spending bill was enacted (Public Law 117-328) requiring diversity action plans for the clinical trials used by the FDA to decide whether drugs are safe and effective. The National Institutes of Health even recently released its Minority Health and Health Disparities Strategic Plan 2021-2025, which focuses on improving diversity and inclusion in NIH-funded research through updated clinical trial policies and guidelines. Trial diversity has come to the forefront of the clinical approval process.
While the pandemic disproportionately affected racial and ethnic minority groups, Black or African American, American Indian or Alaska Native, Hispanic or Latino, and older adults were underrepresented in early U.S.-based vaccine clinical trials. However, due to the intense public scrutiny and shockwaves of change the pandemic created throughout the medical research community, clinical trial sponsors eventually saw an increase in diversity among participants as a result of the pandemic. As researchers adopted a decentralized model that allowed for remote assessments and community-based recruitment, it eliminated the need for trial participants to be within close proximity to the testing center, which is a primary barrier for many minority groups.
As evident during the height of the pandemic, fully embracing recent advances in the pharmaceutical arena can push life-saving treatments into circulation faster. However, patients should not need to wait for the next global health crisis. Plagued by low patient access pools for trials and regulatory roadblocks, rare diseases certainly don’t elicit the same urgency for review and approvals as pandemic treatments and vaccines.
When considering the patient diversity challenge in clinical trials, rare disease trials top the list. If finding strong, diverse trial patient pools for common health conditions like asthma, diabetes, or cholesterol concerns is tough, imagine the hardship of a diverse trial set that properly accounts for child/minority group representation for rare diseases.
According to the National Organization for Rare Disorders (NORD), an estimated 350 million people worldwide have a rare disease. That includes 25-30 million Americans – half of whom are children. Taking an average of 5 years to reach an accurate diagnosis, many patients are then left with no options or hope as only 10% of rare diseases even have an FDA-approved treatment. Rare diseases generally receive inadequate funding and have even longer development and approval timelines, so life-saving treatments are either seriously delayed or never reach the market due to the extremely high cost and trial constraints. NORD’s Rare Disease Video provides additional information, including the often overlooked fact that ALL pediatric cancers are considered rare diseases.
At the start of 2023, we marked the 40th anniversary of the Orphan Drug Act, which drove a push to develop and approve more than 550 drugs and biological treatments for 1,100+ rare disease indications since its inception, and the fifth anniversary of the Memorandum of Understanding Between the US Department of Health and Human Services, FDA, and NORD. However, much like how outdated regulations drove the continued reliance on animal-testing models, rare diseases cannot be left behind in the next era of drug development innovation. As our scientific knowledge evolves, so must our laws and our practices.
Unfortunately, federal mandates continue to threaten rare-disease breakthroughs, and minority groups face added treatment access barriers, according to Jenifer Ngo Waldrop, Executive Director of the Rare Disease Diversity Coalition. “What little federal funding goes toward rare-disease research often flows to conditions that primarily affect white Americans. For example, compare cystic fibrosis (CF), a lung disease with an outsized impact on white Americans, with sickle cell disease (SCD), which disproportionately affects Black Americans. The overall number of Americans with SCD is three times higher than the number with CF. Yet, a study found that government funding between 2008 and 2017 was nearly $2,000 higher per person for CF,” Waldrop stated.
Looking at Figure 2, the lack of patient diversity in rare disease trials is stark – compounding the challenge for minority groups suffering from rare diseases. It is time to reshape rare disease drug discovery and development by embracing new technologies and answering the call of patient-driven advocacy to accelerate future drug development.
WAYS AI CAN FILL THE DIVERSITY GAPS
Since the FDA Modernization Act 2.0 was signed into law, a shift in the drug discovery and development model has begun. As the FDA no longer requires animal testing on drugs in development, the pharma industry is beginning to embrace valid non-animal drug testing methods and technologies. Furthermore, the House and Senate recently pushed for broader FDA integration of non-animal approaches and requested periodic reports on the progress of the removal of any remaining barriers to integrating non-animal methods.
The current technology transition will correct the root issue of the faulty animal-testing model and extend well beyond, into all aspects of trial data. Advances in artificial intelligence (AI) are primed to rapidly disrupt the entire drug development process by ensuring more expansive, diverse, and accurate trial data earlier in the process – helping to get new, safe drugs to patients sooner.
So how exactly can AI help overcome patient diversity challenges and speed up the introduction of safe drugs? Let’s focus on four primary elements – trial site selection, patient recruitment and retention, stem cell diversity, and patient-on-chip platforms.
Site Selection
Clinical trial site selection has always been challenging, but global crises like the pandemic and war in Ukraine created new obstacles. The need to still ensure trial participants accurately represent a diverse global population despite unpredictable setbacks infinitely complicates the process.
As manual search and curation of sources are painfully slow and inefficient, Natural Language Processing (NLP) can quickly integrate advanced searches, systematically analyze potential sites, and condense the selection timeline. To remove discriminatory biases, AI can help identify sites that will provide access to diverse, relevant patient populations if properly trained using a mix of clinical trial metadata, medical and pharmacy claims data, and patient group membership data. Creating the proper cohort composition often depends on the site selected; cognitive computing has been effectively used to match trial cohort sites at the Mayo Clinic. To remove discriminatory biases, AI can also be taught to consider which sites minimize enrollment and participation barriers.
Another option when site selection challenges seem insurmountable, especially with rare diseases, is to consider decentralized clinical trials (DCTs). DCTs often invite more diversity as they allow more flexibility and reduce transportation barriers as some components of the trial – be it drug delivery, imaging, electronic surveys, and remote patient monitoring (RPM) – take place remotely rather than at a primary trial site. AI could improve the patient experience in DCTs through reinforcement learning, computer vision, and AI models designed for temporal data to improve user interfaces and engagement as, despite the added flexibility, DCTs place more responsibility on participants and shortcomings in digital health user interfaces can put retention at risk.
Patient Recruitment & Retention
Once a site is selected, AI can ease core clinical trial design headaches – from patient recruitment to patient monitoring and retention. Recruitment is a costly, time-intensive exercise, but doing it correctly is well worth the investment, as under-enrolled or unfinished trials create a ripple effect of additional costs and lost opportunity.
Unfortunately, 86% of trials do not meet enrollment timelines, almost one-third of Phase 3 trials fail because of slow enrollment and 40% of patients become non-adherent 150 days into a clinical trial. AI, in combination with wearables, sensors, and/or video monitoring solutions, can alleviate some of the tedious, patient-driven self-monitoring tasks like disease diaries and logging while also collecting more reliable data. In the years ahead, generative AI tools will become part of the clinical trial toolkit to automate the patient identification/eligibility process, personalize recruitment messaging, and track patient care and progress – ensuring diversity along the way.
Stem Cell Diversity
The science behind stem cell development and usage has significantly evolved. The ability to create miniaturized organs called organoids, representing a specific patient profile, is rapidly becoming more common, reliable, and affordable. Due to the massive cost reduction in developing human-induced pluripotent stem cells (iPSC), thousands of patient-specific stem cells and organoids can be created with ease, while disease models based on human iPSCs have also advanced.
But what does this mean for patient diversity and where does AI fit in? Stem cells, or more specifically the organoids or full patient-on-a-chip organ systems developed from the stem cells, hold the key to unlocking clinical trial diversity.
“Increasing the diversity of stem cell biobanks presents a key opportunity to ensure disease research captures the full diversity of humanity and benefits all communities in need,” Dr. Greally told the NYSCF. “What we can and should do in terms of research is to try to bring the same amount of genomic insights to all populations so that we can provide the same care to everybody, no matter their ancestry.”
Patient-on-a-Chip Platforms
Patient-on-a-Chip platforms combine the power of AI and stem cell science. Integrating machine learning with patient-on-a-chip technology, interconnected miniaturized versions of human organs created from stem cells, provides a more accurate model of how a specific drug will react in the human body. An advanced patient-on-a-chip platform can test known drugs on thousands of genomically diverse patients-on-a-chip. To optimally train the machine learning, the known drugs (clinically safe ones and unsafe ones) must be tested on hundreds or thousands of different individual patients-on-a-chip.
Advanced Bio-AI platforms can solve the core clinical prediction challenge – predicting, before expensive clinical trials, which drug candidates will work safely in the human body. Whether to determine drug toxicity or treatment viability for a specific patient in a personalized medicine approach or to build an entire clinical prediction platform on specific, yet diverse, patient profiles, this is arguably the most powerful application of AI in terms of clinical trial viability and patient diversity. These clinical trials-on-a-chip capture the full spectrum of drug responses, so the data generated can be used to train an AI model to not only accurately predict if a drug is safe, but also for whom. AI-powered patient-on-a-chip platforms create access to a diverse trial participant pool without the geographic or social constraints that may limit conventional clinical trial models. To start, this is most helpful in the preclinical trial phase as it weeds out the drug candidates that won’t work while providing a more diverse data set to confidently move certain candidates forward into the clinical phase.
THE FUTURE OF AI & PATIENT DIVERSITY
AI has the ability to support patient diversity efforts. Its application at various stages of the drug discovery and development life cycle will provide tremendous ongoing benefits to stakeholders throughout the drug ecosystem. However, the most important benefit is to potential patients. While applicable to all ailments, rare disease patients will benefit most from AI’s ability to better predict clinical safety and efficacy without animal trials from a diverse data set, significantly accelerating new drugs’ path to market.
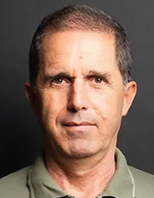
Dr. Isaac Bentwitch is the Founder and CEO of Quris-AI. Prior to Quris-AI, he founded and led three bio-AI technology companies, each of which led revolutions in medicine, genomics, agriculture, and conservation. He is a physician and entrepreneur with a passion for leading interdisciplinary teams of scientists and technologists to tackle impactful challenges in the intersection between machine-learning and life-sciences; and to leverage and commercialize the resulting solutions. One of the companies he founded was Rosetta Genomics, which analyzed the human genome. He led the team at Rosetta Genomics in the discovery of hundreds of novel genes, more so than all the universities in the world combined, and delivered novel cancer diagnostics based on these genes. Its subsidiary, Rosetta Green, was acquired by Monsanto for $35M. Under his leadership, team members at Rosetta went on to lead artificial intelligence at IBM, Google, and Microsoft. Now, at Quris-AI, he and his team are using a similar bio-AI approach to disrupt the drug development process.
Total Page Views: 2748