Issue:June 2021
CLINICAL TRIALS - Statistical Challenges in Preserving Integrity of Ongoing Clinical Trials During the COVID-19 Pandemic
INTRODUCTION
The pharmaceutical industry is a patient-centric industry and is being impacted by the COVID-19 pandemic in ways not seen before. Implications range from a marked slow in recruitment rates due to enforced social distancing policies worldwide to a complete halt in drug development programs as pharmaceutical companies rationalize their investments in these uncertain times. It is unfeasible and often impossible to keep a trial running “as usual” for a variety of reasons, eg, site visits being replaced by remote ones. However, the consequences of this pandemic on ongoing clinical trials can be objectively assessed, and with the correct mitigation strategies put in place, study integrity can be preserved, optimizing use of the available resources for both patients and sponsors.
The following discusses some of the challenges posed to clinical trials from a statistical perspective, offering potential solutions to overcome them with the aim of maintaining scientific accuracy and regulatory compliance. The need for flexibility and how this can be achieved without affecting patients’ safety and study validity will be a key consideration explored. It is worth mentioning that the discussion needs to be tailored to the specific therapeutic area as the challenges faced and the solutions required will be different for each. For instance, studies for life-threatening diseases (eg, oncology) most likely can’t pause for both ethical and practical reasons, whereas studies on less serious illnesses might not suffer this problem.
SAMPLE SIZE: ARE WE GOOD OR NOT?
Getting the sample size right is the foundation that ensures a successful study whilst minimizing the number of patients undergoing potentially invasive study procedures and curtailing costs for the sponsor. In an ideal world, we might think of continuing as planned until normality is restored and procedures restart as before. However, shareholders and investors might disagree: the costs of running a study that doesn’t deliver study results in the originally agreed timelines can be overwhelming for major pharma companies and simply a killer for smaller biotech companies.
One potential solution is to investigate the impact on study power if recruitment was stopped and only patients currently recruited were allowed to continue and complete the study. Such an exercise is similar to what is often done while planning the study, to evaluate the impact of different assumptions (eg, comparator arm response level, variability, etc), with the difference that some new information obtained from external sources might have arisen that allow a more precise characterization of assumptions. If a new study, in which unblinded results had only been made available after the present study protocol was finalized, suggested that the response in the comparator arm was in fact different from what was originally assumed, the current sample size might still allow sufficient power to detect a clinically relevant effect. Whilst this situation is not a very common one, re-evaluating study power in a large number of scenarios (either via closed formulas or simulations) will provide the study team with a better understanding of what actions need to be put in place. However, if the only scenario to allow a sufficient power to be achieved requires a treatment effect twice as large as originally planned, it might be worth considering if the study can continue with the same characteristics prior to the pandemic.
This last example brings up an important item; the extent to which study design itself can be altered to respond to the currently evolving scenario. Let us assume that a study was planned to enrol 200 patients to demonstrate a difference in a continuous outcome between treatments ≥ 3. We further assume that the mean response was 10 in the treatment arm and 5 in the comparator arm with an 80% power (standard deviation = 5 and a one-sided test at a 2.5% level, drop-out rate assumed to be 0% for simplicity). If no further information on the potential treatment effect has arisen from external sources, it is clear that if, eg, only 150 patients have currently been recruited, it is not possible to halt recruitment now because this would leave us with only 68% power and increase the chances of the study being a failure.
In this situation, a viable option is to amend the study protocol to include an unblinded (and previously unplanned) interim analysis with the main purpose of estimating the current treatment effect and deriving measures of future study success (ie, conditional power or predictive power, depending on whether you root for frequentist or Bayesian statistics) given the current data. Using this information (yet considering all available patient-level data), the Data Monitoring Committee can make a better decision as to whether the study is still likely to succeed or not. The advantage of this approach is that only studies that are reasonably likely to deliver positive results, and for which no safety concerns arise, will continue, freeing up resources for other projects and minimizing unnecessary efforts on all sides.
It is relevant to point out that going down this route has implications on study design. If an unblinded interim is added, preservation of type I error rate needs to be maintained via, eg, alpha-spending functions that ultimately imply an increase in the overall sample size. Whilst this might seem counter-productive, considering the difficulties in achieving the planned, and lower, sample size, this ensures the efforts of recruiting additional patients are only done for promising compounds.
ESTIMANDS & MISSING DATA: IS COVID-19 AN INTERCURRENT EVENT?
Intercurrent events should be outlined in the protocol and the estimands defined to outline the approach to each anticipated intercurrent event. During the pandemic, it is expected that protocol amendments will document changes to the design and conduct of studies in order to adapt to travel restrictions, limited access to sites, subjects, and site staff suffering from COVID-19, etc. It therefore seems reasonable to review and adapt study estimands as well.
Protocols may be adapted to allow for a pause in treatment, an alternative treatment, remote visits, larger visit windows, and so on. Subjects may miss visits due to logistical reasons or having the virus. Each of these situations can be treated as an intercurrent event and, for each, the most appropriate strategy selected. The most suitable approach will depend on the details of the trial, the study treatment, and the indication, and will need to be agreed by the whole study team. Consequently, adaptations may be required to the planned analyses to ensure consistency with the estimands defined in the protocol.
COVID 19-related intercurrent events may potentially cause an increase in missing data. Updates to the approach to dealing with missing data may be required to ensure it is done appropriately and is consistent with the estimands.
Where only minimal changes to the trial conduct have been implemented due to the pandemic, it might be reasonable and acceptable not to treat events related to COVID-19 as intercurrent events. The practical impact would simply be a larger than previously anticipated amount of missing data, and this can be tackled by amending the missing data approach outlined in the Statistical Analysis Plan, or by justifying the reasons for no changes.
Trials are ideally designed with the intention of minimizing missing data so adaptations will be required as a reaction to the pandemic. By making some changes at the procedural level, such as making use of local labs or switching to standard-of-care or self-administration of the IP, data can be collected, whereas under the original protocol, these data would have been missing. These procedural changes will have some impact on the data collected, and analyses may need to be adjusted to take this into account. It is therefore critical that case report forms (CRFs) are amended to capture changes to trial procedures at each data collection, as well as reasons for treatment and/or study withdrawal. This information can then be considered in the statistical analyses so that it does not confound the treatment effect.
Let us consider the scenario in which there is an unacceptably large amount of missing data when determining the number of responders for the primary efficacy endpoint at the timepoint of interest. If the original analysis method was based on a generalized estimating equation (GEE), a weighted GEE could be considered instead, which would make use of the data from the preceding visits when computing estimates.
Sensitivity analyses may also be included to assess the impact of intercurrent events by utilizing techniques, such as multiple imputation under different outcome assignments.
IMPROVING DATA QUALITY THROUGH CENTRALIZED STATISTICAL MONITORING
It is likely that access to sites will be restricted for many months. This will necessitate the need for alternative mechanisms to provide monitoring and oversight activities. Therefore the need for centralized statistical monitoring is upon us, and this is highlighted by recent guidance from the FDA on conduct of trials during the pandemic: “If planned on-site monitoring visits are no longer possible, sponsors should consider optimizing use of central and remote monitoring programs to maintain oversight of clinical sites.”
While clinical research associates (CRAs) are no longer travelling to site, centralized statistical monitoring will be required to check for the usual data patterns and anomalies noted here:
- identify missing data, inconsistent data, data outliers, unexpected lack of variability and protocol deviations
- examine data trends, such as the range, consistency, and variability of data within and across sites
- evaluate systematic or significant errors in data collection and reporting at a site or across sites; or potential data manipulation or data integrity problems
- analyse site characteristics and performance metrics
- select sites and/or processes for targeted on-site monitoring
However, it is also important to examine areas not previously required, eg, traditional visits versus non-traditional visits, protocol defined endpoint data collection versus updated endpoint data collection. When analyzing at site or regional level, it may be more important to have some awareness or measure of how far the virus had progressed at time of reporting in that region, what measures were being taken, and how regional healthcare systems were coping. Patterns must be monitored as the trial progresses, but with an awareness that a full understanding of the situation, its impact, and what results may or may not be acceptable is still unfolding. Communication between departments is therefore key; signals must be raised on anomalies, and they must be opened up for multi-disciplined discussion across study teams.
One example of anomalous measures in sites that can be captured by centralized statistical monitoring is if a temperature measurement at a particular site differs from the others due to, eg, miscalibrated thermometers. Tools such as CluePoints and SAS JMP can pick up these differences by analyzing means and variances of temperature readings and comparing across sites. Without site visits, the need for this kind of analysis is increased as such differences are less likely to be picked up. And during these times, analyses should not only be carried out across different sites but by also comparing, for example, traditional patient visits to those that are carried out at a different time or place due to the pandemic.
DOCUMENTATION: TO AMEND OR NOT TO AMEND?
All of the aforementioned areas impacted by COVID-19 call for updates to existing study documentation. However, these updates can be made in different ways, and should be thought through carefully. To a certain extent, the rapidly evolving situation differs across regions and countries. As such, protocol amendments or updates to the Analysis Plans will have to account for this and factor in a certain level of uncertainty.
In addition to the items discussed in previous sections, other study features that should be considered for updating include the following:
Protocol Deviation Plan – many more patients will miss crucial visits, or will have to suspend treatment, and this in turn has an impact not only on the study estimands, but also on the definition of study populations as well as the list of protocol deviations. These are a critical part of a Clinical Study Report as they allow the assessment of the quality of study conduct and largely impact on regulatory decisions. Being able to identify what, in these new circumstances, is a deviation and what is not is key to ensuring the relevant information is collected, displayed, and analyzed. Descriptive comparisons of patterns before, during, and after the pandemic could be considered, both to measure the impact of mitigation strategies as well as to potentially identify under-reporting, ie, cases in which deviations occur but are not reported.
Analysis of Safety Data – in most studies, estimands are defined for efficacy assessment, with safety data often analyzed descriptively by looking at adverse event (AE) frequencies, lab parameter summaries, and trends over time in line plots. The nature of this pandemic, though, is likely to affect the level of AE reporting, resulting in an increase of mild-to-moderate events, such as pyrexia, sore throat, and other upper pharyngeal trait illnesses that in normal times could be dismissed as nuisance by patients and not reported. On the other hand, patients with undiagnosed COVID-19 might have further comorbidities that might never be related to the virus itself and would be incorrectly evaluated. Allowing, for example, stratified analysis of AE patterns might help to identify any relevant trend. Patient narratives will be key to ensure any such case is captured and properly discussed.
Data Management Plan – some additional built-in checks might need to be added, and the general schedule of data flows could benefit from an assessment of what the current situation could entail. For instance, the eCRF could be updated to collect relevant data on COVID-19 symptoms within a respiratory trial, or specific causes for treatment discontinuation.
It is important to stress that no matter how careful the assessment of the potential COVID-19 impact is, it is unlikely that all relevant impacted features will be identified straight away. Continuous monitoring will be required to capture every change in the current situation.
SUMMARY
The COVID-19 pandemic is the biggest challenge the world has faced in decades; it is impacting every aspect of daily life, and clinical trials are not exempt. The integrity and feasibility of ongoing studies is threatened as the outbreak continues globally.
The biopharma industry’s response to the COVID-19 crisis has been commendable, with new treatments and vaccines already in testing. This industry focus, however, along with the burden the pandemic is placing on hospitals and medical centres worldwide is highly disruptive to ongoing clinical trials. The impact over the coming months and years will be widespread and multifaceted, and although it is not possible yet to identify the full extent and severity, it is possible to examine core components of studies on an individual basis and identify the most affected areas. By putting mitigating strategies in place, it may be possible to salvage some of, if not all, a study’s potential.
REFERENCE
- https://www.fda.gov/news-events/ press-announcements/coronavirus-covid-19-update-fda-issues-guidance-conducting-clinical-trials
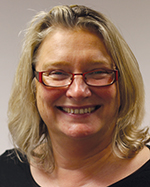
Karen Ooms is Executive Vice President and Head of Statistics at Quanticate, responsible for overseeing the Statistics department at Quanticate. She is a Chartered Fellow of the Royal Statistical Society and has a background in biostatistics spanning more than 25 years. Prior to joining Quanticate in 1999 (Statwood), she was a Senior Statistician at Unilever. She earned her MSc in Biometry from the University of Reading.
Total Page Views: 3279