Issue:June 2022
CLINICAL TRIALS SOLUTIONS - Cracking Down on the Rising Costs of Drug Development: How Pinpointing the Complexity of Individual Patients Can Improve Success Rates
DRUG DEVELOPMENT IN THE PAST 2 DECADES: AN EVOLUTION OR A REVOLUTION?
Drug development has changed significantly throughout the past 2 decades. Nearly 20 years ago, in 2004, the FDA was launching the Critical Path Initiative with the objective of more efficiently transitioning from discovery to NDA. At the same time, personalized medicine was in its infancy, and the pharma industry was already focusing their efforts on reducing the time and cost of clinical trials. The ambitions were lofty, challenging, and occasionally at odds with each other. Twenty years later, the cost of bringing a new treatment to the market has increased from $800 million to about $2.6 billion, and development timelines remain unchanged.1 Does it mean that our industry has failed to achieve a major transformation and that this quest is hopeless? This conclusion would be too simplistic and quite frankly untrue.
In truth, we have made great leaps forward in areas from drug discovery to personalized medicine. New types of therapies ranging from small and large molecules to stem cells, drug-device combination products, gene therapies, and novel delivery technologies have emerged. Personalized medicine has moved to the forefront with more generalized use of biomarkers, surrogate markers, genomics, epigenomics, proteomics, metabolomics, and imaging. Beyond this, new trial designs have also evolved (for example, Bayesian adaptive clinical trial design). Drug development has indeed progressed substantially, and personalized medicine has made a great step forward. Innovation has addressed some of the most critical needs for treatment, as evidenced by the consistent and sustained decline in cancer death rates, and all would agree that any patient is better treated now than 20 years ago.2
CLINICAL TRIAL COMPLEXITY
Yet, with all this progress, we, as an industry, are still working diligently to reduce clinical development cost and timelines and to push the boundaries of treatment optimization. With new drug types and personalized medicine comes increased complexity while demonstrating optimal benefit versus risk remains a challenge. While some disease and patients have found better cures, the overall probability of technical in most therapeutic areas remains low (Figure 1).3 The major drivers of drug development cost, timeline, and failure persist, with the inability to demonstrate adequate efficacy or safety as the largest and most difficult obstacles.4
Our main challenges include the following:
- High number of patients required to demonstrate efficacy, driving long recruitment timelines and high trial costs
- Increased data per trial patient (increased number of procedures or outcomes) needed to characterize patient response to treatment
- Complexity and cost of the study procedures themselves (imaging, biomarkers, etc)
- Operational complexity to achieve trial goals [geographies, number of sites; suboptimal patient recruitment/enrollment (due to rigid inclusion criteria, drop out, non-compliance, etc)]
Considering only external costs, the median cost of a clinical trial patient varies between $10k and $30k, depending on the therapeutic area.5 Let’s explore these sources of inefficiency in more detail.
ONGOING INITIATIVES TARGETING TRIAL CONDUCT EFFICIENCY
To address these remaining obstacles, operational optimization strategies have been launched and broadly implemented for several years, including quality by design, blockchain, and process optimization with lean and six sigma approaches. With these methods, efficiency gains result from operational rationalization and improvement, as well as generating data of better quality. For example, the Clinical Trials Transformation Initiative, CCTI has made progress with Transforming Trials 2030. Among recent efforts, they are interested in facilitating the development of recommendations for the conduct of decentralized clinical trials, an essential step forward in the conduct of clinical research.
Historically, the pharmaceutical industry makes incremental improvements over time, yet the unexpected disruption caused by Covid-19 is motivating companies to make more radical transitions to reach their corporate goals. Initiatives like Modernizing Clinical Trial Conduct from Transcelerate will use data and experience issued from solutions implemented during Covid-19 and evaluate them across the board. In all cases, the industry will benefit from having all stakeholders, including regulators, simultaneously focus on the patient voice and patient-centric approaches.
COULD PATIENT-CENTRIC APPROACHES IMPROVE EFFICACY EVALUATION?
Inability to demonstrate efficacy of experimental therapeutics is a major source of late-stage clinical trial and program failure, and significant efforts have been invested in the identification of factors that can improve “assay sensitivity” in indications with high failure rates like pain and depression.4,6 One option is to focus on data variability – or the “noise” in clinical trial data resulting in part from interpersonal differences in response to treatment. Approaches that reduce data variability would have a direct positive impact on the treatment effect size, resulting in ripple effects on the trial costs (fewer patients needed) and duration (less time needed to recruit patients). This approach has the added ethical benefit of exposing fewer patients to experimental drugs during clinical trials while improving success rates and effectively accelerating patient access to innovative treatments.
Reducing interpersonal data variability requires an understanding of the characteristics that differ between individual patients that relate to or explain their variable treatment response. Traditionally, clinical trials collect a wide range of biological, physical, or anatomic data – ranging from vital signs to the investigator’s interpretation of patient disease status and/or improvement. We would, however, assert that patients are people and should be viewed holistically as having a unique personality, motivations, and beliefs. Acknowledging the industry is an era of patient centricity, understanding patient personality as a key component and an influencer of data variability would complete the full picture of patient data and only improve efficacy evaluation.
Understanding and reducing clinical data variability resulting from interpersonal differences would enable the field to understand the efficacy and potential of new therapies more quickly and effectively. Placebo response, for example, is one of the most significant sources of data variability in clinical trials, and it may account for a significant portion of the observed treatment effect (Figure 2).7 Considering the contribution of the placebo response to clinical trial failures – and the fact that it has continued to increase in the past several decades despite the best efforts of scientists and physicians – the time is ripe to employ novel solutions. The placebo effect is a true psycho-social-biological phenomenon that is intrinsic to each patient and is influenced by the patient’s individual personality, expectations, and beliefs – among other factors. This large diversity and quantity of data (like traits of personality) requires the use of new advanced data analytic methods. AI and ML can pinpoint the relevant variables and their relative importance and may simplify this complex information into a single patient characteristic: an individual score relating to each patient’s placebo responsiveness. Integrating this information into clinical data analysis can yield substantial rewards: increased assay sensitivity, increased study power, improved success rates, and decreased sample size.
The financial impact of reducing clinical data variability can be equally impactful, relating to both decreased overall clinical development costs and earlier market launch. A Phase 3 patient cost may be as much as $40,000 per patient; thus, reducing sample size of a Phase 3, 1000 patient study by 30% could save about $12 million in direct costs and 3 months of recruitment time.5 Considering that every month of a Phase 3 trial cost an average of $671,000, reducing timelines by 3 months would save about an additional $2 million.8 Beyond this, an additional 3 months of marketing under patent protection may represent between $75 to $210 million in sales (depending on the drug). Similarly, reducing data variability may avoid or reduce the risk of an inconclusive trial – which avoids a minimum of 2 years of delay, clinical study and manufacturing costs, additional patients exposed to the study drug, and ultimately delayed sales. To better understand this, let’s consider a case study employing these methods.
CASE STUDY: A PATIENT-CENTRIC APPROACH TO REDUCING THE IMPACT OF PLACEBO RESPONSE ON CLINICAL DATA ANALYSIS
We have used predictive algorithms based on machine learning to understand the spectrum of placebo responsiveness in a clinical trial patient population at baseline based on patient psychology, expectation, and other factors (eg, age, demographics, baseline disease intensity). This modeling approach is intended to address the inherent interpersonal differences in placebo response as a source of noise in the data with minimal trial burden and absolutely no added study risk. Including placebo responsiveness as a baseline covariate – as typically used by clinical trial statisticians to account for factors that differ between patients – can safely and significantly reduce data variability and improve study power.9,10
These machine learning-based models can be calibrated specifically for each disease and then used to calculate a single score for each patient in a given study. When used in the statistical analysis, the reduction in data variability improves the ability to detect true treatment efficacy. Currently, models have been constructed in multiple diseases with more than 10 clinical studies completed. Model performance has been consistent in chronic pain, Parkinson’s disease, and ophthalmology (dry eye disease), with additional studies ongoing in areas like psychiatry, auto-immune disease, and neurology.11,12 In general, it has been demonstrated to explain between 25%-35% of data variability related to the placebo response across endpoints and indications, regardless of route of drug administration and study design.
This ~30% reduction in placebo response-related data variability in indications evaluated to date can yield tremendous gains in study power and reduced enrollment. To illustrate this concept, one can consider a clinical trial with N=100 patients that is powered to 80% (Figure 3). Reduction in variance by 30% translates into increasing study power from 80% to 92% – meaning the risk of trial failure due to false negative results is significantly decreased. Looking at this another way, the trial now has an equivalent power to a trial that included 43% more patients. Conversely, this same study now only requires 70 patients to achieve a power of 80%. Over time, use of such a covariate could result in reduced sample size in clinical trials, which quickly translates to reductions in clinical trial costs and timelines, and quicker delivery of drugs to market.
SUMMARY
The pharmaceutical industry sorely needs new approaches to improve efficiency of drug development to shorten timelines and reduce costs. Patients are complex beings with highly variable biological – and psychological – makeups, yet only biological characteristics have traditionally been considered when analyzing clinical trial data. Taking a more holistic, patient-centric approach by considering patients’ individual psychology, perceptions, and beliefs provides drug developers the opportunity to quantify these interpersonal differences between patients and address this source of variability in data analysis and interpretation. In the example of the placebo response, new approaches powered by machine learning have been shown to successfully reduce data variability by 30% or more, which translates into increased success rates and decreased enrollment. These novel methods can provide substantial savings while improving market access of novel therapeutics.
REFERENCES
- DiMasi JA, Grabowski HG, Hansen RW. Innovation in the pharmaceutical industry: New estimates of R&D costs. Journal of health economics. 2016;47:20-33. doi:10.1016/J.JHEALECO.2016.01.012.
- Siegel RL, Miller KD, Jemal A. Cancer statistics, 2020. CA: A Cancer Journal for Clinicians. 2020;70(1):7-30. doi:10.3322/CAAC.21590.
- Clinical Development Success Rates and Contributing Factors 2011–2020. https://pharmaintelligence.informa.com/~/media/informa-shop-window/pharma/2021/files/reports/2021-clinical-development-success-rates-2011-2020-v17.pdf.
- Fogel DB. Factors associated with clinical trials that fail and opportunities for improving the likelihood of success: A review. Contemporary Clinical Trials Communications. 2018;11:156. doi:10.1016/ J.CONCTC.2018.08.001.
- How Much Does a Clinical Trial Cost? – Sofpromed. Accessed January 9, 2022. https://www.sofpromed.com/how-much-does-a-clinical-trial-cost.
- Harrison RK. Phase II and phase III failures: 2013-2015. Nature Reviews Drug Discovery. 2016;15(12):817-818. doi:10.1038/NRD.2016.184.
- Hafliðadóttir SH, Juhl CB, Nielsen SM, et al. Placebo response and effect in randomized clinical trials: meta-research with focus on contextual effects. Trials 2021 22:1. 2021;22(1):1-15. doi:10.1186/S13063-021-05454-8.
- Martin L, Hutchens M, Hawkins C, Radnov A. How much do clinical trials cost? Nature Reviews Drug Discovery. 2017;16(6):381-382. doi:10.1038/NRD.2017.70.
- Fda, Cder, Goldie, Scott. Adjusting for Covariates in Randomized Clinical Trials for Drugs and Biologics with Continuous Outcomes Guidance for Industry. Accessed March 8, 2020. https://www.fda.gov/Drugs/GuidanceComplianceRegulatoryInformation/Guidances/default.htm.
- CHMP. Committee for Medicinal Products for Human Use (CHMP) Guideline on Adjustment for Baseline Covariates in Clinical Trials.; 2015. Accessed March 8, 2020. www.ema.europa.eu/contact.
- Branders S, Dananberg J, Clermont F, et al. Predicting the placebo response in OA to improve the precision of the treatment effect estimation. Osteoarthritis and Cartilage. 2021;29:S18-S19. doi:10.1016/J.JOCA.2021.05.032.
- Branders S, Rascol O, Garraux G, et al. Modeling of the Placebo Response in Parkinson’s Disease. In: Proceedings of the International Parkinson and Movement Disorders Society MDS Virtual Congress. ; 2021:369.
- Häuser W, Bartram-Wunn E, Bartram C, Reinecke H, Tölle T. Systematic review: Placebo response in drug trials of fibromyalgia syndrome and painful peripheral diabetic neuropathy – Magnitude and patient-related predictors. Pain. 2011;152(8):1709-1717. doi:10.1016/j.pain.2011.01.050.
- Rief W, Nestoriuc Y, Weiss S, Welzel E, Barsky AJ, Hofmann SG. Meta-analysis of the placebo response in antidepressant trials. Journal of Affective Disorders. 2009;118(1-3):1-8. doi:10.1016/j.jad.2009.01.029.
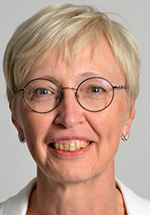
Dr. Dominique Demolle serves as Chief Executive Officer of Cognivia (formerly Tools4Patient) since its inception in 2013. She earned her PhD in Biochemistry from the University of Brussels. She joined the Clinical Research Group of GD Searle and then Eli Lilly. She has held positions with increasing leadership responsibilities at Eli Lilly and Company with the Lilly Indianapolis Clinical Research Unit in the US and the European Operational Staff management and ultimately became the Associate Director of Global Early Phase Operations. In 2007 she co-founded and successfully developed a Consulting Clinical Research Organization, including partnerships with pharma and biotech before she left to set up Cognivia with previous colleagues.
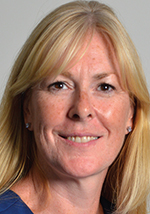
Dr. Erica Smith joined Cognivia (formerly Tools4Patient) as VP of Business Development in 2018 and assumed the role of Chief Business Officer in December, 2021. She earned her PhD in Biomedical Engineering from The University of Michigan in Ann Arbor, MI. She began her career in the pharmaceutical industry at Genetics Institute/Wyeth Research in Cambridge, MA, and Pfizer in Groton, CT. She then worked for several CROs, developing a strong track record of sales leadership, strategic planning, developing and executing corporate growth strategies, and marketing.
Total Page Views: 5945