Issue:January/February 2023
CLINICAL TRIALS - Historical Controls in Rare Disease Drug Development: Using RWE to Overcome Key Challenges
INTRODUCTION
Rare disease drug development poses unique challenges that can be overcome by using real world evidence (RWE). Small populations, pediatric patients, and the desire from patients and their caregivers to receive active therapy can make the conduct of randomized trials with placebo control untenable.
As an alternative, regulatory agencies have allowed the use of RWE to estimate a historical control (HC) compared to patients enrolled in an uncontrolled study. Additionally, healthcare payers understand HCs can provide value by describing potential treatment benefit relative to untreated patients in their populations. The following will explore the advantages of using HCs, as well as several considerations for their effective use, including scientific methodology and regulatory guidance.
BENEFITS OF HISTORICAL CONTROLS
HCs can offer several advantages over concurrently collected control groups. For example, they can be created from multiple sources of disease natural history information. Disease natural history describes the demographic, genetic, environmental, and other variables (eg, treatment modalities, concomitant medications) that relate to the disease’s development and outcomes over the course of disease. Natural history information also describes the current standard of care or emergent care, which may alter disease morbidity and mortality. Sources include ongoing prospective studies, such as patient registries, retrospective data from medical charts, control populations from previous trials, and expert opinion.
Another benefit is fewer patients are required in clinical trials using HCs, thereby reducing time to trial completion and speeding drug approval. However, the HC group needs to be very similar to the treated group in all respects, including outcome assessment, disease severity, duration of illness, prior treatments, and other aspects of the current standard of care that could affect the measurement and timing of treatment outcomes. To address these limitations, researchers may use selection techniques that consider independent risk factors that may bias the assessment of the key treatment outcomes in order to match control subjects to the patients enrolled in the active treatment arm.
HC information has been successfully used to gain drug approval — for example, Brineura (cerliponase alfa) was approved to slow the loss of ability to walk or crawl in symptomatic pediatric patients three years of age and older with CLN2 disease.1 Historical information on CLN2 patients was obtained from the DEM-CHILD database. As three domains of the rating scale used as primary outcome were measured differently between the database and the clinical study, the primary efficacy endpoint was limited to a single domain of this motor scale. The differences in the patient populations were addressed by matching controls to specific patient characteristics (specifically, baseline motor score, baseline age, genotype, gender, and age of first symptom) in the active treatment population. The FDA concluded the improvement in the active treatment group was not affected by the original differences identified between the active and HC population and approved the drug in April 2017.
SCIENTIFIC METHODOLOGY
Two different methodologies are used in the application of HC information in clinical development. The first, Bayesian statistical methodology, uses estimates from historical data to generate a prior probability distribution representing expected event rates for the outcome of interest in a trial. This is then combined with the observed data from the clinical trial to create an estimate, known as the posterior distribution, which is essentially a weighted average of the prior and the observed data from the clinical study. The application of Bayesian methodology has been recognized by the FDA as useful in early phase clinical trials involving pediatric populations.2 Applications include more realistic estimates of adverse event rates in small populations; drawing statistical strength from adult data to make decisions about device performance in pediatrics; and shortening trials through use of adaptive designs and predictive probability of trial success before all patients finish the trial.
The second, frequentist methodology, involves the direct comparison of patient-level information from historical data to patients in the clinical study. This provides an approach using similar statistical methods to those used in a randomized clinical trial. The lack of randomization is likely to introduce bias due to differences between the historical and trial population. The differences may be reduced by several different strategies, including restriction of the patient population entering the HC; matching of the HC to increase similarity with patients in the active arm; and statistical methods to adjust for observed differences. Propensity scores, which are the probability of treatment assignment conditional on observed baseline characteristics, have been used to adjust for multiple patient factors in non-randomized studies.3 The propensity score allows for the design and analysis of a non-randomized study so that two or more groups of patients with similar characteristics are compared in the assessment of treatment effect.
The decision to take a Bayesian or frequentist approach to using historical information is generally influenced by the availability of subject-level information to compare with the active trial arm and the preference of regulatory agencies in a specific disease area or application. International regulatory agencies have normally favored direct comparison of patient-level data rather than estimated rate approaches when an HC is completely substituted for a concurrent control arm in a Phase 3 or registration study.
REGULATORY & PAYER GUIDANCE
Regulatory agencies in the US and Europe have been exploring the use of disease natural history information in drug development. Recently, the FDA published draft guidance outlining the different sources and applications of natural history information, including identification of patient populations, trial planning, and the use of HC information to establish drug safety and efficacy.4 The EMA has also published their own guidance for the use of historical controls as part of its guidance on the conduct of trials for small populations.5
Healthcare payers also use clinical trial evidence to assess the effectiveness of treatments in making reimbursement decisions. These assessments often need to involve comparisons against multiple alternative therapies that would be impractical in the context of clinical drug development. The use of HCs can provide a comparative population not studied in the clinical trials. Some formal health technology assessment (HTA) guidance on the use of external controls and RWE is available from the UK’s National Institute for Health and Care Excellence (NICE).6,7
A recent analysis of international HTA submissions from 2011-2019 found out of 433 submissions that involved the use of single arm trials, 52% contained some type of supplementary dataset that can be designated as HCs — most often from prior clinical trials, real world data, or both.8 Submissions that contained external controls based on RWD had the most positive recommendations (59%) followed by submissions with prior trial ECs (49%). This suggests the widespread incorporation and acceptability of HCs in drug reimbursement decisions.
SUMMARY
RWE from rare diseases is available from a wide variety of sources for use as an HC in rare disease drug development. HCs are used in drug approvals, HTAs, and in describing the impact of new therapies in different clinical settings for rare diseases. As a result, the utility of HCs based on RWE should be evaluated for potential applications to increase speed of product approval, reimbursement, and clinical practice adoption.
REFERENCES
- FDA Summary Review Report for Brineura https://www.accessdata.fda.gov/drugsatfda_docs/nda/2017/761052Orig1s000SumR.pdf.
- Challenges in Pediatric Study Design and Analysis, and Some Potential Solutions. Thompson, LA. FDA presentation https://events-support.com/Documents/Peds_Workshop_2012_v3.pdf.
- Austin PC. An Introduction to Propensity Score Methods for Reducing the Effects of Confounding in Observational Studies. Multivariate Behav Res. 2011 May; 46(3): 399–424. doi: 10.1080/00273171.2011.568786.
- FDA Rare Diseases: Natural History Studies for Drug Development Guidance for Industry https://www.fda.gov/media/122425/download.
- EMA Guideline On Clinical Trials In Small Populations. https://www.ema.europa.eu/en/documents/scientific-guideline/guideline-clinical-trials-small-populations_en.pdf.
- D.M. Phillippo, A.E. Ades, S. Dias, S. Palmer, K.R. Abrams, N.J. Welton NICE DSU technical support document 18: methods for population-adjusted indirect comparisons in submissions to NICE. http://nicedsu.org.uk/wp-content/uploads/2017/05/Population-adjustment-TSD-FINAL.pdf.
- NICE decision support unit Observational data TSD. http://nicedsu.org.uk/technical-support-documents/observational-data-tsd/.
- Patel D. et al. Use of External Comparators for Health Technology Assessment Submissions Based on Single-Arm Trials. https://doi.org/10.1016/j.jval.2021.01.015.
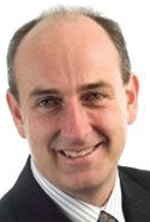
Dr. William C. Maier, MD, PhD, Vice President, Rare Disease, Drug Development Sciences at ICON. He has over 25 years of experience in drug development and commercialization at pharmaceutical companies in Europe, Canada, the US and Asia. At ICON he works with pharmaceutical companies throughout the world to provide regulatory, strategic, and scientific guidance on medical treatment development and commercialization. He is a member of the EMEA’s European Network of Centres for Pharmacoepidemiology and Pharmacovigilance (www.encepp.eu). In addition, he is a frequent speaker at medical conferences, has had academic appointments in the UK (Dundee) and the US (North Carolina) and is a member of the Royal Society of Medicine in the UK.
Total Page Views: 3150