Issue:April 2020
CLINICAL STUDY REPORTING - Assessing the Value of Interim Analyses in Clinical Trials
INTRODUCTION
Phase 3 trials are the most extensive and rigorous types of scientific investigations of a new intervention, with the objective to compare the efficacy and safety of the new intervention to the standard-of-care. In these randomized trials, the sample size needs to be sufficiently large in order to make the proper assessments.
Data needed for sample size calculations usually comes from studies on similar drugs, devices or compounds, small-scale studies, and historical data. With these estimates coming from vastly different sources, estimates are apt to be imprecise. Thus, interim analyses provide an opportunity to update these values during the study or uncover issues that necessitate stopping the study early.
The following will review how and when an interim analysis would be valuable and how, with examples and outcomes, it can be applied in a clinical trial setting.
WHAT IS AN INTERIM ANALYSIS AND WHY IS IT IMPORTANT?
An interim analysis compares randomized arms at any time point before the end of a Phase 3 trial and usually occurs before recruitment is complete. It is especially appealing to the regulatory agencies and the sponsor, allowing for decisions and changes to be made in the middle of the study. The analysis provides several options and opportunities for the trial, for example:
-An opportunity to re-estimate the sample size
-An opportunity to modify the trial design
-An option to stop the trial for efficacy or futility
-An option to continue the trial as originally planned.
As Phase 3 trials are generally cost-intensive, it makes sense to insert an interim analysis in the planning protocols of the trial, particularly as a Phase 3 trial is needed to gain FDA or EMA approval.
Depending on the disease prevalence and the required number of subjects for the study, a traditional Phase 3 may take a couple of years to recruit and a couple of years to follow the patients. By the time the last subject enters the study and has his or her last visit, it could be years between the first patient in and the end of the study. It’s a long time to go without any real knowledge about the efficacy or safety of an intervention.
WHEN TO CONDUCT AN INTERIM ANALYSIS
The timing, frequency, and methods for the analysis should always be specified in the trial protocol before the study starts. There may be a single analysis or more at different points of time depending on the number of subjects and the drug or device being tested. The analysis needs to be timed when there are enough subjects enrolled in the trial to “see something” (ie, the less data you have, the harder it is to see signals). On the other hand, you don’t want to conduct an analysis so far into the study that there’s little value. For example, if 90% of the subjects have already been enrolled and you do an interim analysis and find you should drop one treatment arm or stop the study early, the benefits of the interim analysis, in terms of cost savings or the safety of the subjects, are limited. Generally, depending on the size and scope of the trial, midway through is a good point to conduct the analysis. Additionally, there may need to be an adjustment of alpha to preserve the overall Type I error rate of the study.
STATISTICAL APPROACHES WITH THE INTERIM ANALYSIS
When a study is conducted, it is necessary to determine the number of people that need to be enrolled to show a statistically significant treatment benefit, if one actually exists. So, the method for doing a sample size calculation means there needs to be previous knowledge of treatment responses and how impactful the treatment is expected to be.
These values are typically found in previous Phase 2 studies or literature from a comparable drug in the same family. But those estimates are not always accurate. Sometimes a study will be conducted thinking there might be, for instance, a 10-point benefit in cholesterol reduction; however, there was only a 7-point benefit. But even though the 7-point benefit might be clinically relevant, because the study wasn’t large enough, it isn’t statistically significant and therefore the drug wouldn’t pass regulatory approvals.
One way to use the interim analysis is to re-estimate the sample size to make sure the assumptions made in the sample size estimate are holding. Another is to stop the study because it’s doing so well the results will be statistically significant or stop the study because it’s doing poorly. There might be a modification in the study design, ie, if there are three arms in the study with a low dose of the drug or high dose of the drug or standard of care, the analysis might show that the effect is only pronounced in the high-dose group, but not the low-dose group. Therefore, enrollment would stop in the low-dose group. Or it might show that everything is going as planned and the study would continue as is.
When a statistical analysis is conducted, a p-value is calculated, which is the probability that results seen are due to chance alone. If, for example, the p-value is 0.04, that means 4% of the time there would be as big of a difference observed in the two treatment arms just by chance alone, even if both arms were the same. And so that would result in rejecting the null hypothesis of no difference between the arms.
That is known as a false positive, incorrectly rejecting the null hypothesis and concluding a difference in treatments, which is also called a Type I error. Every time the data are analyzed, there’s a 5% chance of making a Type I error, if alpha=0.05. As the number of analyses increase, the chance of making a Type I error also increases. As a result, an alpha adjustment is often required.
APPROACHES FOR ALPHA ADJUSTMENT
An alpha adjustment is needed to preserve the overall Type I error rate. Not surprisingly, researchers have established different methods to account for multiple analyses and ways to adjust the alpha. There isn’t any one consensus but there are a few that are commonly used.
- Pocock
– Same alpha for interim and final analysis
– 2 analyses, a = 0.0294 - Haybittle-Peto
– Very strict alpha adjustment at interim, no adjustment at final
– 2 analyses, a = 0.002 at interim and a = 0.05 at final - O’Brien-Fleming
– Strict alpha adjustment at interim, small adjustment at final
– 2 analyses, a = 0.0054 at interim and a = 0.0492 at final
OUTCOMES OF DIFFERENT METHODOLOGIES – EXAMPLES
The application of different methodologies can make a significant difference in the outcome of a study as outlined by the following contrived example (although similar situations have been experienced in real-life studies). Let’s say we are designing a pivotal Phase 3 clinical trial to compare a new treatment to standard-of-care. The outcome is “treatment success.” Based on the results of an earlier Phase 2 study, the expected percent of treatment success is 34% in the experimental group and 20% in the control group. The sample size calculation yields that 414 subjects are needed (207 in each arm) to achieve 90% power at alpha=0.05. Once the study is conducted, the results are as follows:
Treatment Arm
- 59 successes at end of study (28.5%)
- 30/104 (28.8%) at interim
Control Arm
- 41 successes at the end of the study (19.8%)
- 20/104 (19.2%) at interim
Scenario No. 1 – No Interim Analysis
Scenario No. 2 – 1 Interim Analysis – Midway
WHAT’S THE CONCLUSION
At the end of the study, the p-value was 0.041, which was less than 0.05, so without an interim analysis, the results would suggest a statistically significant treatment difference. However, conducting the interim analysis necessitated an adjustment of the alpha at the end of the study and depending on which method was chosen, there were different conclusion. If Pocock was used, the null hypothesis would not have been rejected. However, if O’Brien-Fleming was used, the null hypothesis would have been rejected. Clearly the methodology chosen had an important impact on this study example.
How to decide which methodology to use is not an easy choice for pharma or medical device companies. Different methods have different assumptions and scenarios. If, for example, a new drug is viewed as extremely promising, and the study has the potential to meet the strict alpha threshold at the interim analysis, O’Brien-Fleming might be favored over Pocock, in the hopes of stopping the study for benefit at the interim analysis, saving time and money.
IS AN INTERIM ANALYSIS ALWAYS BENEFICIAL?
With the time and investment involved in clinical trials, particularly if the drug or device has advanced to the Phase 3 level, it’s incumbent on all involved, from the sponsor as well as partners like the CRO, to carefully decide whether an interim analysis is appropriate in the specific setting. If so, all details about the timing, frequency, and method should be specified in the trial protocol before the study starts.
To view this issue and all back issues online, please visit www.drug-dev.com.
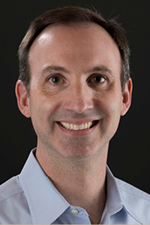
Dr. Paul C. Stark is the Director of Biostatistics for PHASTAR, Inc. and the head of the Cambridge, MA, office. He has more than 20 years of experience designing and analyzing data from clinical trials, surveys, observational studies, and large datasets. Dr. Stark earned his undergraduate degree from Cornell University and his MS and Doctoral degrees from Harvard University. He has served as the Director of Biodata Sciences at Clinlogix and the Director of Biostatistics and Epidemiology at New England Research Institutes. Before working at NERI, he was a Professor and the Director of Statistics at Tufts University for almost a decade and is still an Adjunct Professor. He has authored or coauthored more than 70 articles in peer-reviewed journals, focusing on statistics, cardiology, nephrology, oral-health research, and oncology.
Total Page Views: 3459