Issue:January/February 2019
CLINICAL ANALYTICS SOLUTIONS - Reducing Clinical Cost Budget Variations With State-of-the-Art Data Lifecycle Management Solutions
INTRODUCTION
Clinical trials are characterized by significant challenges, with respect to schedule delays and cost overruns. Some industry statistics are given below:
-More than 80% of clinical trials experience delays ranging on average from 1 to 6 months, costing companies upward of $35,000 per day, per trial.1
-A mere 10% of trials are completed on time.1
-Only 14% of clinical financial planners are highly confident in their budget forecasts.1
-The variance between forecasted and actual clinical trial costs for life science companies can be as high as 16%. Acceptable variance range is 5% to 10%.1
Data-driven decisions offer higher potential in controlling the schedule and cost drivers, thus enabling reduction in schedule and budget variance. This article explores an approach for how sponsor’s operational data, coupled with syndicated data and Real World Evidence (RWE) data, can enable predictive analytics on clinical cost drivers using a clinical big data and Machine Learning (ML)-enabled platform. The predictive clinical cost drivers can be used to create adaptive clinical financial budgets that include baseline spend, actual spend and projected expenses. This approach also provides details on automating the budgeting of the clinical trial financials based on trial assumptions and re-budgeting based on revised trial assumptions (as part of trial execution).
This article is composed of multiple sections. Section 1 pro- vides an overview of cost categories and introduces cost drivers, which are foundational for the forecasting approach. Section 2 introduces the forecasting approach on the cost drivers. Section 3 provides a high-level overview on the model’s functional and technical details. The final section (section 4) reviews overall solution components.
SECTION 1: DRILL DOWN ON CLINICAL TRIAL COSTS
Figure 1 indicates the cost ranges and average schedule for various trial phases across multiple therapeutic areas.
The key cost categories, with percentage ranges and relative variance, are provided in Table 1 (patient recruitment and retention, clinical procedure, site administration and site monitoring, and site management accounts for approximately 60% to 80% of clinical trial costs).
Forecasting of the costs associated with each category involves multiple levels of decomposition for each cost category against cost groups and cost line items. A typical sponsor budget can be decomposed into cost group/account group, and cost group can be further decomposed to cost line items. Cost line item is typically associated with one or more measure items. An approach to financial prediction is to develop a forecasted model, which provides a baseline forecasting model for each measure item based on the measure item’s predictor variables. Table 2 (a snapshot of a trial overall budget) provides examples of such a measure item with the corresponding predictor variable.
SECTION 2: CLINICAL TRIAL COST DRIVERS – FORECASTING APPROACH
Based on Table 2, a clinical forecasting approach can be created based on the following five steps:
Step 1 – Forecast all the predictor variables in each of the predictor variable groups. This will involve Machine Learning approaches.
Step 2 – Using the forecasted predictor variables and independent variables, calculate measure item. This calculation will be directly arithmetic in nature.
Step 3 – Using the negotiated cost for each measure item (in case of outsourced trials) or historical cost adjusted (in case of in-house trials), calculate cost for each individual cost line item. This can be aggregated for all the cost-like items in a cost category, and further aggregated to get the budget forecast.
Step 4 – Feed the model with the actual values for the predictor variables (as the trial progresses) to create projected values of predictor variable (for the remaining trial period).
Step 5 – Continuous learning of the model based on the variance between the actual values and baseline forecast and its updated projected forecast.
If all the cost line items are analyzed as per the Table 2, a list of predictor variables can be collated to build the forecasting model. Top list of predictor variables are typically associated with country details, site details, subject details, subject visit details, and trial month details. The forecasting approach using these predictor variables enables building a dynamic and continuous learning system that can be improved based on available study data. Multiple models are necessary based on the combination of therapeutic area/indication for higher levels of accuracy. A representation of some of the model input and predictor variable details are provided in Figures 2 and 3.
SECTION 3: MODEL FUNCTIONAL & TECHNICAL OVERVIEW
This section goes into functional and technical details of the adaptive forecasting model indicated previously.
Adaptive Forecasting Model (Functional Detail): Functional detail depends on the data sources and data processing of the data entities associated with the predictor variable. For example, in a case of predicting country approval data (a predictor variable in country detail predictor variable group), the key sources are the sponsor’s country milestones data and syndicated data source containing country milestones data (for similar TA/Indication). The key inputs are country milestones (Planned, Actual, Historical) from the sponsor and syndicated sources, and the output is country approval data (Forecasted/Projected). Some of the pre-processing steps include identifying prior milestones, forecast of the prior milestones, correlation of prior milestones, and forecast of the country approval data based on the correlation factors and prior milestones. Based on the actual data (after completion of prior milestones), the model will be re-forecasted for completed prior milestones to provide new projected country approval date.
In another example of predicting first patient enrolled date for a particular site (a predictor variable in subject detail group), the key sources are sponsor operational data, claims data, registry data, and syndicated data. The key entities are site enrollment detail (Planned, Historical), patient population (Historical/Current) and competing trials, which are extracted from claims, registry, and sponsor operational data. Some of the processing steps include identifying patient population based on claims data, co-relation of enrollment lead time (first patient) with factors such as site distance, number of trials/sites, site experience. An initial forecasting model can be developed to forecast country approval data using the aforementioned features and using the current population to forecast the country approval data. Similar to the previous example, the model will reforecast using actual details of prior milestones.
Adaptive Forecasting Model (Technical Detail): The technical approaches with respect to some of the models that can be used for clinical cost driver forecasting are in Table 3.
SECTION 4: SOLUTION OVERVIEW
Building a dynamic forecasting model for improved accuracy on clinical budgeting and costs involves data ingestion from multiple sources, data quality and harmonization, aggregation, and metrics generation. Saama’s Life Science Analytics Cloud (LSAC) for study planning enables protocol optimization, investigator site selection, and patient identification. This section gives an overview of solution components and features. Figure 4 and Table 4 depict some components and features to look for when evaluating such solutions.
A brief description of the aforementioned components are provided below.
Source Layer: The source layer is enabled by intelligent adapters. These adapters are enabled to pull in data and meta-data near real-time for standard EDC and CTMS industry products. It also uses adapters for pulling in clinical data (views) from leading CROs. The adapters include intelligent file watcher utility to pull third party files from drop zone and do metadata checks. The source layer contains the ability to configure file level checks and remediate file loading issues. The layer also supports configuration to support both incremental and full load of clinical operational data.
Data Quality: Data quality (DQ) is based on a library of data quality rules for management of structural and business integrity data quality checks. The data quality module enables self-service functionality to perform data profiling and to create new DQ rules. It also enables remediation of source data in case of data quality issues.
Data Harmonization: The data harmonization module enables users to set up harmonization rules for harmonizing the operational data from multiple sources. The harmonization rules establish the ranking of the source attributes to be matched in a common data model. Based on the source data and the ranking rules, source data gets harmonized into the common data model.
Common Data Model: The common data model (CDM) is made up of two sub-components. The first is a canonical model to standardize the integration layer. This model is a flat staging layer model based on clinical operational subject areas. It enables automated mappings from landing to canonical model. The second sub-component is the consolidated operational data store. This store consolidates all raw operational data in to a single common data model. It enables both standard CDM and supports sponsor-specific CDM extensions. It also supports data versioning and full process and data traceability (landing to CDM). The data access to the common data model is enabled through fine grained access control (column, row, value level access).
Metrics Rules Management: Based on industry standards for clinical operational metrics (MCC, Transcelerate), the metrics engine allows an out-of-the-box library and also allows users-defined metrics. The Metric library enables users to set their own metrics definition to create a custom metric in the analytics layer.
Metrics Engine: The metric rules are used to create metrics in the analytics layer from data from the common data model. The metric engine can be scheduled to execute on demand or on schedule to develop metrics data through incremental or full load of data from the common data model layer.
ML Algorithms: The solution allows machine learning training on historical data to predict KPIs on the current trials. For example, based on historical country approval milestones, a machine learning model to predict country approval date for a study can be developed. This model allows reviewing the model accuracy on a continuous basis, to retrain and to redeploy for improved accuracy.
Analytics Layer: The analytics layer is a consolidation of all conformed data into a single analysis dataset layer. It contains both operational KPI created through KPI library and predictive KPI created through machine learning libraries. It also supports storage of KPIs, which can have calculation variation depending on study hierarchy.
Visualization: The visualization includes canned reports, exploratory analysis reports, RBM reports and machine learning-based dashboards. The capabilities of threshold management, alerts, tasks and notification management are also part of this module. This module supports operational reports on key standard operational KPIs with interactive filters. It enables users for BYOR (bring your own reporting tool), and developed external reports can be enabled for access. Visualizations rendering to a user is based on the data access security model.
Foundational Features: The system allows both system workflows (e.g. data transformations) and business workflows (e.g. DQ issues or KPI breach). It abstracts the complexity of open source components through a self-service orchestration layer. All the changes to the data layer supports audit trail and data traceability across all layers.
The features of the solution also include a virtual assistant, which allows conversational experience on key intents (topics) for a scope of operational subject areas. It enables users to view graphs on demand (on known intents) to provide details on a conversation. It supports continuous training of the virtual assistant for accuracy improvement, with respect to responses from the virtual assistant. The virtual assistant is trained on the common data model. The roadmap includes a plan to support voice-based conversations in future.
REFERENCES
- http://www.appliedclinicaltrialsonline.com/closing-variance-gap-challenges-clinical-trial-budget-management-andforecasting
- https://www.centerpointclinicalservices.com/blogposts/driving-drive-drug-innovation-and-market-access-part-2-utilizing-technology-and-maximizing-resources/
- http://journals.sagepub.com/doi/abs/10.1177/1740774515625964?ssource=mfr&rss=1
To view this issue and all back issues online, please visit www.drug-dev.com.
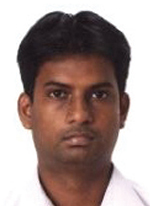
Srini Anandakumar is the Senior Director of Clinical Analytics Innovation at Saama. He is responsible for leading the solution development for next-generation clinical repositories based on Big Data and AI. He has more than a decade of experience building clinical analytics solutions for enabling both analytics and submission pathways. His experience includes product management and consulting in the clinical R&D space. His current passion is to explore the possibility of AI applications to bring in efficiencies in clinical development.
Total Page Views: 6917