Issue:March 2023
AUTOMATED SOLUTIONS - Automation & Shared Knowledge Pave the Way Into the Future
INTRODUCTION
Automation is a vital component to fuel the labs of tomorrow and to ensure drug development continues at the rapid pace seen in response to the COVID-19 pandemic. By no means is automation a novel concept for most research labs, but its swift advancement and expansion into new fields – such as synthetic biology – have shown us that we are only witnessing the start of what is possible. Together with open access data, which allows scientists around the globe to benefit from each other’s findings, it paints a bright picture of a future that is full of exciting new possibilities.
Laboratories all over the world have been shaken by the COVID-19 pandemic. This global event forced them to step up to the challenge, finding ways to handle unprecedented sample volumes quickly and efficiently for both research and diagnostics. This put automation in the spotlight, not only as a convenient tool, but a necessity, as obtaining accurate results with such speed would not have been possible if every sample was handled manually.
Alongside the need for rapid diagnostic testing, it was crucial to develop a vaccine as fast as possible, to curb rampaging infection rates, and help the world recover both medically and economically. Laboratories came together, sharing their discoveries through open access (OA) data portals to ensure breakthroughs would not only benefit one organization or country, but the entire world. This shines light on another important point – how much more we can accomplish by sharing our knowledge, instead of guarding it.
Synthetic biology has also played a major role in winning ground against the pandemic, allowing the creation of a candidate vaccine a mere 66 days after the viral genome was released.1 This vaccine was created using synthetic genes, an approach that is not only useful for developing vaccines, but might also be helpful in combatting cancer, making it a powerful tool for drug discovery.
SYNTHETIC IS THE NEW NATURAL
Synthetic biology is based on metabolic engineering, but takes this concept a step further to encompass non-metabolic applications, with the aim of creating new biological building blocks and systems, or improving on those found in nature. In contrast to metabolic engineering, this discipline uses a systematic approach based on generalizable methods, making synthesis and sequencing of DNA more accessible and less costly.
One of the principles of synthetic biology is the “design-build-test-learn” (DBTL) cycle, which helps achieve a design that fulfills certain requirements through multiple iterations, learning by doing.2 The first step is designing a biological system that is expected to be able to perform the task. This is followed by building that design using DNA parts, and integrating them into a microbial chassis. When this is done, the system can be tested – using a variety of assays – to see if it is indeed suitable for the desired application. During this phase, a lot of data is collected through production- and omics-profiling. This is then used during the learn phase to influence the next design, as it is unlikely that the optimal system, demonstrating the right properties, is obtained the first time. Multiple iterations are usually required, and so the learning phase relies on the ability to predict the biological systems behavior in response to a design change. Machine learning can be of great help here, statistically linking an input to an output, to predict the result for completely new scenarios.
MAKING THE COMPLEX EASY
Synthetic biology opens up many new possibilities, and its structured nature makes it easier to move forward toward new discoveries. However, although the principles are straightforward, the synthetic biology workflows are generally complex, and rely heavily on automation to achieve rapid and reproducible results. Without it, this new and exciting discipline would not be able to progress at a sufficient rate.
Higher and higher levels of automation can be seen in many labs all over the world, from handheld electronic pipettes that can aspirate and dispense several channels simultaneously, to fully automatic liquid handling workstations powered by intelligent software that can follow the most complex protocols. Many laboratories that perform high throughput screening or clinical and analytical testing – as well as large-scale biorepositories – simply would not exist without this technology.
In addition, automation all but removes the human variability factor, increasing reproducibility and ensuring productivity through staff absences, labor issues, and a variety of other challenges.
THE 3D PUZZLE
3D cellular models are becoming increasingly popular in drug discovery, providing more physiologically relevant results than 2D cell cultures or animal models. These microenvironments can more accurately mimic the complex immune response of human tissues, which is of great importance, helping to avoid costly late-stage failures of drugs in clinical trials. Grown using a variety of approaches, 3D cell culture workflows are another example of research benefiting from automation.3 Automated solutions are required both for consistent growth of 3D cell cultures, and to support cell imaging and real-time cytometry assays for drug discovery because manually examining cells under a microscope is both labor intensive and time consuming. Automated culture maintenance and imaging improves reproducibility and throughput, as well as removing the risk of missing a key cellular event when leaving the lab – an important consideration for any cell-based study.
COLLECTIVE KNOWLEDGE
Many biological studies produce a tremendous amount of data, with thousands of genetic sequences produced daily. If not reused, this data will go to waste, together with all the possible insights that it could have provided.4 Considering the entire human genomic sequence only requires 1 GB of storage space, this is truly a shame. Fortunately, it is becoming increasingly common for researchers to upload their data, providing open access to anyone who is interested. If shared in an effective and comprehensive way, this data can greatly increase the impact of the original experiments, making the most of something that took significant funding and research time to produce. By sharing sequencing data globally, initiatives such as the Darwin Tree of Life and the 100,000 Genomes projects are made possible.5 The former is a tribute to biodiversity, aiming to sequence the genomes of 70,000 species of eukaryotic organisms found in the UK, while the latter project uses data from patients affected by a rare disease or cancer, with the goal of advancing diagnosis and personalized treatment. Furthermore, giving open access to data also provides other benefits, such as increased credibility; if the research data is made available and possible to reproduce, it becomes more believable.
However, for others to make use of data, it needs to be organized and documented properly. This type of careful cataloging of results is equally beneficial to groups that do not plan to upload their data because it promotes traceability and repeatability. There are many software platforms that work well with automated workflows to offer scientists a convenient way to plan experiments and manage results, as well as receive feedback on the outcomes. For example, the Synthace Life Sciences R&D Cloud allows scientists to automate experimentation and share insights. Berlin-based Labforward is another company offering increased lab connectivity, enabling scientists to effectively connect their devices to make research data more manageable and easily accessible. On the same note, a company in San Francisco called Benchling had developed a platform that helps standardize and centralize R&D data, accelerating and improving research while working seamlessly with third-party hardware. The software company Titian offers similar services, driving digitalization of research and advancing management and traceability in every step of the sample lifecycle. Many of these advances are being made possible through the work of the SiLA Consortium, a non-profit industry body working to develop free and open system communication and data standards, providing researchers with an opportunity to connect, interface with their instruments, and merge data across the laboratories. These are only a few examples and, as more and more scientists grasp the benefits of laboratory digitalization, an even greater choice of solutions will become available.
SUMMARY
Automation is a great way to catapult laboratories into the future, speeding up sample preparation and establishing high throughput versions of complex workflows while minimizing the risk of cross contamination, eliminating human errors and saving time and resources. Automated solutions are particularly important to fields such as synthetic biology, allowing the development of a more structured approach. This has enabled synthetic biology to become a powerful tool in drug discovery, replacing the hit-and-miss strategies commonly employed in many laboratories with the design-build-test-learn principle. This relatively new field is empowered by powerful machine learning software, which can make predictions based on large data sets that are beyond the capabilities of the human mind to quickly and easily comprehend. Driving science forward in such a structured manner helps speed up new discoveries and reduce the number of failed experiments.
Learning from our own mistakes can be of great help, but learning from the mistakes of others performing similar research in parallel is a far more powerful tool, as many laboratories around the world are currently discovering. There are several software platforms that have been developed especially for this purpose, helping scientists to document, store and share their data with others, as well as streamlining workflows through connectivity between programs and hardware. With so many tools available, digitalizing and immortalizing your research has never been easier, bringing about the laboratory of the future, which is not only fully digitalized, but connected to research centers around the globe, letting everyone reap the benefits of hard-won knowledge.
REFERENCES
- Synthetic biology speeds vaccine development, 28 September, 2020 https://www.nature.com/articles/d42859-020-00025-4.
- A machine learning Automated Recommendation Tool for synthetic biology, Nature Communications, September 15, 2020 https://www.nature.com/articles/s41467-020-18008-4.
- Don’t miss a beat with live cell imaging, Tecan Journal, 2021 https://www.tecan.com/tecan-journal/dont-miss-a-beat-with-live-cell-imaging.
- Sharing biological data: why, when, and how, FEBS Letters, 11 April, 2021 https://febs.onlinelibrary.wiley.com/doi/10.1002/1873-3468.14067.
- Open access data benefits millions of scientists around the world and is essential for a rapid response to the COVID-19 pandemic, EMBL Communication, 20 October, 2020 https://www.embl.org/news/science/open-data-sharing-accelerates-covid-19-research/.
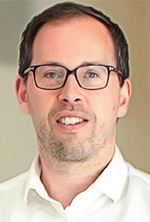
Luca Valeggia is the Senior Vice President of Laboratory Automation and interim General Manager of the Genomics Reagents business at Tecan. Over the past decade, he has focused on driving innovation in lab automation and digitalization. He played a pivotal role in defining Tecan’s product portfolio, and in commercializing some of the most successful lab automation solutions on the market. His passion for advancing research and scaling healthcare innovation has contributed to Tecan’s growth strategy and leading position in the life sciences sector. He is a strong advocate of collaborative research, striving to leverage the potential of automation in new applications, from specialty immunodiagnostics to 3D cell culture and synthetic biology. He is also a driving force behind Tecan’s digitalization strategy. He earned Masters degrees in Molecular Biology from the University of Basel, and Advanced Studies in Management, Technology and Economics from ETH Zurich, both in Switzerland.
Total Page Views: 2454