Issue:January/February 2024
ARTIFICIAL INTELLIGENCE PLATFORM - Advancing Precision Medicine With bfLEAP™: Next Generation AI for Drug Development
INTRODUCTION
In the ever-evolving landscape of drug development, the pursuit of precision medicine has gained tremendous momentum. This approach aims to revolutionize healthcare by tailoring treatments to individuals based on their unique genetic makeup, lifestyle, and environmental factors.
BullFrog AI (NASDAQ: BFRG) is a digital technology company that is leveraging the potential of artificial intelligence (AI) and machine learning (ML) to advance this field and improve success rates in drug development. AI and ML have emerged as game-changers in the drug discovery and development industry, enabling computers to analyze vast amounts of complex data, identify patterns, and extract valuable insights for drug developers and clinicians. These technologies hold promise for revolutionizing patient care and advancing more effective therapies to market faster. Why is this so important?
- Getting a drug to market can take close to a decade, with a cost of $0.8 to $2.8 billion, while patients are desperately waiting for treatments that often don’t come.1
- Almost 90% of drugs fail at some point along the way.2
BullFrog AI is focused on changing this narrative using bfLEAP™, an AI platform exclusively licensed from the prestigious Johns Hopkins Applied Physics Laboratory (APL) for biological and chemical pharmaceutical therapeutics applications. bfLEAP is a robust platform that can help to rapidly detect anomalies and uncover hidden associations within patient data, making it a powerful tool with the potential to help researchers predict drug responses and identify patient subgroups.
With an advanced technical architecture, bfLEAP solves numerous scalability and flexibility challenges, facilitating the comprehensive analysis of diverse and complex data sets. Using unsupervised ML algorithms and proprietary clustering techniques, the platform identifies potentially meaningful and understandable information, paving the way for the creation of personalized treatments, optimized clinical trials, disease progression predictions, and drug target identification.
The following explores the technical architecture and capabilities of bfLEAP, delving into its ML algorithms, proprietary clustering techniques, and visually understandable outputs. Additionally, some of the potential use cases for the platform in drug development and discuss future developments that hold tremendous promise are examined.
TECHNICAL ARCHITECTURE – HIGH-THROUGHPUT, DATA-AGNOSTIC PROCESSING POWER
The true strength of bfLEAP lies in its highly efficient architecture. The platform is capable of handling either small, homogeneous data sets or large, complex data sets with equal ease. By overcoming the scalability and flexibility challenges that are commonly faced by researchers and clinicians, bfLEAP can provide a path to more efficient and comprehensive data analysis.
One of the standout features of bfLEAP is its ability to handle incomplete data sets, an all-too-common occurrence in real-world clinical settings. Whether it comes from patient dropouts, missed appointments, technical glitches, human error, or other causes, researchers are frequently faced with data gaps upon study completion. By effectively incorporating and interpreting incomplete information, this platform ensures that valuable insights are preserved.
Data agnosticism is another key attribute of bfLEAP, allowing it to integrate, analyze, and interpret heterogeneous data sources. Whether inputs consist of medical records, demographics, genomic data, or real-world evidence, the platform’s ability to integrate and process disparate data sets is a valuable benefit to users. This versatility enables a more comprehensive analysis, unlocking new possibilities for understanding even the subtlest relationships.
bfLEAP excels through its powerful high-throughput processing capabilities, fueled by the TinkerPop™ API for parallel computing. This enables the rapid analysis and interpretation of data, facilitating the accelerated identification of meaningful information and potentially enabling a more efficient drug development process. This will provide researchers and clinicians actionable insights on an optimized timescale, allowing them to make data-driven decisions quickly.
UNSUPERVISED ML & PROPRIETARY CLUSTERING ALGORITHMS
The bfLEAP platform takes full advantage of the power of unsupervised ML algorithms to discover hidden patterns and relationships. Unlike supervised learning methods that rely on labeled data and predefined assumptions, its unsupervised ML approach allows bfLEAP to uncover patterns organically – without human intervention. This approach ensures important insights are not overlooked due to false or limiting assumptions.
One of the significant advantages of bfLEAP is its proprietary suite of clustering algorithms, all of which are designed to uncover unknown associations between key entities, regardless of data type or use case. This versatility and flexibility allow bfLEAP to open doors in drug development that may not have previously been known to exist.
With a total of over 200 analytic utilities and algorithms at its disposal, the bfLEAP platform provides a comprehensive toolkit for data analysis and clustering. These algorithms have been carefully selected and fine-tuned over time to deliver optimal performance with maximum efficiency.
Another important feature of the platform is its anomaly detection algorithm, a random subspace mixture model (RSMM). In a rigorous benchmarking study that analyzed 12 open-source data sets, this algorithm outperformed the top 10 currently used algorithms for anomaly detection.3
EXPLAINABLE AI
Transparency and interpretability are essential qualities in AI platforms, though they are often lacking. This is particularly critical in domains such as drug development, in which the details of complex relationships – like drug interactions – are essential to comprehend.
bfLEAP addresses this issue through its layered processes and explainable AI approach. The platform provides critical context to the data outputs, allowing researchers and clinicians to understand the steps involved in generating insights. This purposeful transparency is designed to not only facilitate comprehension, but to also enable sponsors to validate and trust their results.
A key component of bfLEAP’s “explainable AI” approach is its graph analytics output. This visual representation of relationships and correlations within the data greatly simplifies interpretation. Through the display of nodes and edges that users can explore and interact with, weighted relationships between various factors are visually illustrated, facilitating an intuitive understanding of the data. This important software feature enhances sponsor engagement, as it presents complex information in an easily digestible format.
Yet another advantage of bfLEAP’s explainable AI approach lies in its minimal custom coding requirements. Apart from the initial data cleaning and ingestion process, the platform requires few adjustments, ensuring shorter lead times and consistent output interpretation. This ease of use and interpretability maximizes efficiency and allows the platform’s full capabilities to be leveraged without the need for extensive programming expertise.
POTENTIAL USE CASES IN DRUG DEVELOPMENT
The versatility of the bfLEAP platform offers numerous potentially impactful use cases within the field of drug discovery and development. The following lists some specific ways in which this platform can help provide meaningful impact in the clinical research field:
Identifying Patient Subgroups to Better Predict Drug Response: By analyzing patient data, bfLEAP can assist in the identification of distinct subgroups based on factors such as genetic information, biomarkers, demographic information, or a variety of other patient factors and characteristics. This can help researchers and clinicians predict drug response within specific patient subgroups, potentially facilitating personalized treatment approaches and improved patient outcomes.
Informing Better Inclusion & Exclusion Criteria for Clinical Trials: Designing effective clinical trials requires the inclusion of relevant patient populations while excluding confounding factors. bfLEAP can aid researchers in identifying significant patient characteristics, helping them to optimize inclusion and exclusion criteria. This helps ensure clinical trials are highly targeted, efficient, and yield robust results.
Predicting Disease Progression: By thoroughly analyzing longitudinal patient data, the bfLEAP platform can help researchers predict disease progression patterns. With this knowledge in hand, clinicians may be able to intervene at appropriate stages more effectively, facilitating early detection, proactive treatment, and improved disease management for patients. Further, they may be able to use this knowledge to better design the subsequent phases of their program.
Identifying Pathways for Drug Application: bfLEAP analyzes genomic data and gene expression data to uncover associations between networks of genes and proteins. By identifying these novel connections, the platform may help researchers discover important new targets and disease biomarkers. This enables the development of transformative therapies for unmet areas of patient need.
Drug Target Identification: By leveraging its comprehensive data analytics capabilities, the bfLEAP platform can assist researchers in identifying potential drug targets. By exploring intricate relationships and patterns within the data, the platform may help to expedite the drug discovery process and facilitate the development of highly targeted precision medicines.
Drug Repurposing and Rescue: The ability to recognize previously undetected patterns also creates the potential to revisit the efficacy of failed drug candidates or repurpose existing drugs for new indications.
FUTURE DEVELOPMENTS FOR bfLEAP
As the landscape of drug development evolves, BullFrog AI remains committed to advancing bfLEAP and addressing this industry’s growing needs. The platform’s architecture is also designed to accommodate future developments, allowing for the seamless addition of bolt-ons to enhance its already impressive capabilities. One key example is the implementation of natural language processing (NLP) and GraphQL integration, which can be leveraged to mine the existing literature and further expand scientific knowledge.
This platform is continuously being refined and enhanced. Recent updates have streamlined the data input process, making this process faster and more efficient. This enhancement benefits sponsors who often operate on tight timelines.
Overall, by prioritizing collaboration and innovation with leading organizations and eminent scientists, BullFrog AI strives to ensure bfLEAP will remain at the forefront of AI-driven drug development for years to come. Continued technological advancements will mean even greater sophistication and more powerful capabilities that will enable researchers and clinicians to unlock new frontiers in the pursuit of advanced therapies.
With an unwavering commitment to innovation and collaboration, BullFrog AI is well positioned to help shape the future of drug development, revolutionize patient care, and transform the landscape of precision medicine.
REFERENCES
- Wouters, O.J., McKee, M., & Luyten, J. (2020). Estimated research and development investment needed to bring a new medicine to market, 2009-2018. Jama, 323(9), 844-853.
- Sun, D., Gao, W., Hu, H., & Zhou, S. (2022). Why 90% of clinical drug development fails and how to improve it? Acta Pharmaceutica Sinica B, 12(7), 3049-3062.
- Savkli, C., & Schwartz, C. (2021). Random Subspace Mixture Models for Interpretable Anomaly Detection. arXiv preprint arXiv:2108.06283.
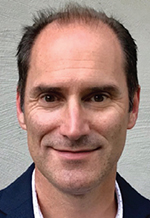
Dr. Thomas Hazel, BullFrog AI VP of Drug Development, has over 20 years of industry experience in R&D and business development. He most recently served as senior VP of R&D at Seneca Biopharma, overseeing the development of the company’s stem cell-based therapeutics platform. He has been granted 9 US patents and multiple foreign patents in stem cells and regenerative medicine. He earned his PhD in Genetics at the University of Illinois College of Medicine.
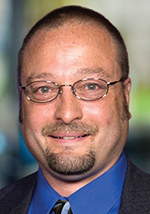
JT Koffenberger, BullFrog AI Chief Information Officer, has over 30 years of experience leveraging IT services for business. His range of expertise includes providing better security through custom application development and automating cumbersome business practices. He is Founder of Delmarva Group, LLC and Director of IT Architecture for Day and Zimmerman.
Total Page Views: 2250