Issue:May 2022
ARTIFICIAL INTELLIGENCE - Modernizing Your Clinical Development Safety Practices With Artificial Intelligence
INTRODUCTION
Digital transformation has been shaking up the pharmaceutical industry for the past decade as the value of technology becomes apparent, but also increasingly necessary for business continuity in the face of rapidly growing health data.1 In fact, artificial intelligence (AI) is believed to be the most disruptive technology to the pharmaceutical industry in 2021.2
Though still relatively nascent in parts of the pharmaceutical industry, AI and machine learning (ML) have already been explored for numerous use cases across the continuum of drug development, delivery, and post-market activities with some success. Among these, leveraging AI/ML for safety processes in clinical research as well as post-market pharmacovigilance (PV) hold tremendous value for organizations to process and extract more insight from clinical trials preemptively, improving the safety of new products for patients.
AI IN POST-MARKET PHARMACOVIGILANCE
AI/ML as well as natural language processing (NLP) have an inherent value to post-market pharmacovigilance. In a post-market environment, pharmaceutical companies are in a position of knowing a lot about their product but not a lot about the individual patients receiving it. Once a product is on the market, the patient population receiving it is infinitely larger than in a clinical trial setting, and data about them and their reported experiences is increasingly disparate. For instance, they may switch doctors, which can fragment their medical history. Additionally, they could be reporting their experiences to their doctors, to a call center, or even on forums and social media. Nevertheless, it’s the responsibility of the pharmaceutical company to capture and create as holistic of a picture as possible to identify potential adverse events. At the rate data is both growing in volume and becoming more disparate, this is simply not a problem that companies can hire their way out of.
NLP supports post-market pharmacovigilance by converting information from text-based sources, such as doctor’s notes in electronic health records, call center transcriptions, and social media, to a structured format that can be read by a computer. From there, AI/ML can fulfill its most essential purpose: analyzing massive amounts of captured health data to identify significant data points. For safety teams, applying AI/ML helps them to spot potential adverse events to review and subsequently supports the automation of reporting.
BRINGING AI TO THE CLINICAL RESEARCH PROCESS
The value of AI/ML for safety in clinical development may be less inherently obvious to pharmaceutical companies exploring use cases compared to a post-market environment. Due to the necessarily stringent requirements for qualified patients in randomized clinical trials (RCTs), there are a very small number of patient cases to observe. What’s more, in a clinical research setting, medical professionals have the luxury of knowing a lot about the drug in question as well as the individual patients. However, every case in an RCT is disproportionately more important than in a real world, post-market setting. Researchers have an imperative to uncover every possible safety outcome in clinical development to streamline the approval process and mitigate safety challenges when the drug is approved and available to patients.
Simply put, the use of these technologies in clinical research is fundamentally the same as in post-market activities. The key difference is a focus on analyzing depth of patient information versus breadth, looking at one case at a time. Reducing the opportunity for human error in data capture and analysis will help researchers to identify potential safety concerns more holistically and deliver safer treatments to patients, making the jobs of safety teams easier in the more reactionary post-market environment.
CLINICAL RESEARCH USE CASES THAT BENEFIT FROM AI FOR SAFETY
Applying AI/ML to clinical trial data analysis will have a tremendous amount of value for virtually any type of clinical trial. Any tool that aids in making sense of data and uncovering all possible outcomes will be beneficial to the creation of safe, holistically researched treatments as well as contribute to faster timelines and lower R&D costs by mitigating manual analysis. However, there are key emerging use cases that stand to see significant value from the use of these technologies.
Remote & Hybrid Trials
Following the pandemic, remote and hybrid RCTs are primed to become the new standard for trials. Some things, such as blood work and physical examination, will always require in-person visits, but the realized capability to glean data remotely offers contract research organizations the opportunity to expand their recruitment efforts and better retain patients for the duration of the RCT.
Strategies for ensuring the quality of data from remote trials is on par with what regulators have come to expect from traditional trials are currently being explored. By their nature, remote RCTs primarily glean patient-reported outcomes. Furthermore, measures must be taken to fill in gaps in the absence of physically seeing the patient. For example, if patients are asked for their pain level on a scale of 1 to 10, this might be more easily gleaned in person while a patient report could vary significantly by individual. As this trial format becomes more and more ubiquitous, AI and NLP capabilities help structure this information and notice trends that can contextualize findings for deeper understanding and accurate conclusions.
Longer Clinical Trials
Similar to the post-market environment, patient and drug information is often available to clinical researchers in text-based formats, such as patient questionnaires. This necessitates the use of NLP tools to analyze and process that information. This capability can be particularly valuable in longer RCTs, during which researchers must analyze patient histories and drug results over an extended period of time. Many RCTs have long document trails and questionnaires that can add up to hundreds of pages of patient data that researchers must analyze.
High Risk Drug Trials
The ultimate goal of an RCT is to determine if the benefits of a drug outweigh its risk so that it can go to market and help patients. To this end, AI is especially valuable in higher risk drugs, in which a researcher may know that a treatment cures or alleviates an illness or condition but comes with the potential for unpleasant side effects. NLP and AI can be applied to datasets to produce word clouds of potential signals that patients would be more likely to experience negative side effects. This can be valuable in helping researchers notice safety trends and issues they were not looking for to begin with.
REGULATORY & INDUSTRY ADOPTION OF AI IN CLINICAL TRIALS
As the technology matures and its use cases for the industry expand, AI capabilities have shifted from a buzzword add-on to treatment development and delivery processes to a foundational tool for success. Leaders in the pharmaceutical industry have long been viewed of skeptics of digital transformation. However, the challenges they are increasingly faced with in research as well as post-market to process data in near real time are ones they cannot solve with more hiring. These hurdles have been the catalyst for a change in tune that can be seen not just across the industry, but with regulatory bodies as well.
The industry will surely benefit from the adoption of AI-driven safety practices. In the highly regulated pharmaceutical industry, leaders must feel confident the AI they implement can intuitively adapt to data privacy rules, regulatory reporting requirements, and ultimately glean the insight necessary to validate the safety of products. Regulators are similarly exploring the ways in which AI can be implemented reliably for clinical development.
Regulations around how AI can be used is forthcoming from the Food and Drug Administration (FDA) and others. In the meantime, regulators are certainly encouraging companies to implement AI to aid in the exploration of its value. They recognize that anything that cuts the cost of clinical trials opens up more opportunities for more innovative drugs, especially those for smaller and more rare groups of people, are a good thing.
SUMMARY
A higher level of analytics is required to succeed in the face of evolving challenges in life sciences. Advancing our understanding of human health through better, more insightful decisions will promote a new era of innovation in drug development as we simultaneously explore new classes of medicines like mRNA and precision treatments as well as new research methodologies like remote trials. The proven promise and potential of AI and ML to accelerate drug discovery while cutting costs and risks will be the underpinning for ushering in this new era. The insights these technologies enable researchers to uncover will go beyond routine processes to support the discovery of new product indications. By continuing to explore the ability of these technologies to deliver better and safer treatments, pharmaceutical companies can benefit not only the advancement of the medical community, but the quality of life of patients around the world.
REFERENCES
- Seagate Launches New Data-Readiness Index Revealing Impact Across Four Global Industries as 30 Percent of Data Forecasted to Be Real-Time by 2025, Business Wire, November 2018, https://www.businesswire.com/news/home/20181126005585/en/Seagate-Launches-New-Data-Readiness-Index-Revealing-Impact.
- Artificial intelligence will be most disruptive technology across pharmaceutical industry in 2021 and beyond, Global Data, January 2021, https://www.globaldata.com/artificial-intelligence-will-disruptive-technology-across-pharmaceutical-industry-2021-beyond/.
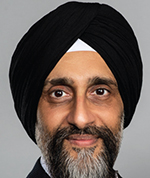
Updesh Dosanjh, as Practice Leader for the Pharmacovigilance Technology Solutions business unit of IQVIA, is responsible developing the overarching strategy regarding AI and Machine Learning as it relates to safety and pharmacovigilance. He has more than 25 years of knowledge and experience in the management, development, implementation, and operation of processes and systems within the life sciences and other industries. Most recently, he was with Foresight and joined IQVIA as a result of an acquisition. He earned his Bachelor’s degree in Materials Science from Manchester University and his Master’s degree in Advanced Manufacturing Systems and Technology from Liverpool University.
Total Page Views: 5096