Issue:September 2024
ARTIFICIAL INTELLIGENCE - Accelerating Drug Discovery & Development: The AI Revolution is Here
THE PARADIGMS, THEY ARE A-CHANGIN’
In the realm of drug discovery and development, the integration of artificial intelligence (AI) is not just a trend; it’s a paradigm shift. Traditional methods, characterized by lengthy time lines, high costs, and significant failure rates, have long been a source of frustration and unsuccessful attempts at delivering new drug products to market.1 But that is set to change … profoundly.
Enter AI, which offers more than just a beacon of hope; its skillful integration with existing drug discovery and development methods and human expertise promises more certainty in less time — with lower costs — benefiting sponsors, patients, and all other stakeholders.2 The following delves into how AI is transforming the drug discovery sector by enhancing predictability and efficiency. It will also explain our unique approach, and why Logica® stands at the forefront of this ongoing revolution, democratizing access to cutting-edge drug discovery tools for companies of all sizes.
THE AI-DRIVEN TRANSFORMATION IN DRUG DISCOVERY
The journey of drug discovery has always been fraught with challenges. However, the advent of AI is rewriting the narrative. By embedding AI into the drug discovery process, we’re witnessing a significant enhancement in every phase of discovery, from target identification to lead optimization and more. This integration is not merely about accelerating the process, but about improving it by instilling a level of predictability and efficiency that were previously unattainable using traditional methods.
THE POWER OF DATA IN REALIZING AI’S POTENTIAL
At the heart of AI’s success in drug discovery is the quality of the data ingested.3 AI thrives on data — preferably, lots of it — and its integration into the drug discovery process creates a powerful feedback loop. This loop, enriched by deep experimental expertise — again, more is better — not only refines AI models, but also ensures they evolve in tandem with emerging scientific insights, thereby enhancing their predictive accuracy and operational effectiveness. Consequently, this symbiosis between AI and data transcends traditional discovery methods, paving the way for a new era of innovation where breakthroughs are faster and more aligned with human biology.
THE ROLE OF EXPERIMENTAL DATA IN AI-DRIVEN DISCOVERY
The synergy between AI and experimental data is pivotal. The “wet science” data serve as the foundation for training AI models, optimizing outputs, and improving program success. This symbiotic relationship ensures that AI models are not operating in a vacuum but are continually refined by real-world experimental results, leading to more accurate predictions and optimized strategies.4
THE FOUNDATION OF AI MODELS
Experimental data are the lifeblood of AI in drug discovery, encompassing a wide range of information — from biochemical interactions and phenotypic responses to pharmacokinetics and toxicology profiles. These data do more than just feed AI models; they shape their architecture, guiding the learning process to reflect biological realities. By training AI models with diverse and comprehensive datasets, we ensure that the insights and predictions they generate are grounded in the complex nuances of human biology.
OPTIMIZING OUTPUTS THROUGH CONTINUOUS LEARNING
One of the most compelling aspects of AI in drug discovery is its ability to learn and improve over time. Each new experiment contributes data that can be used to refine the model’s predictions, making them increasingly accurate. This iterative process is akin to an ongoing dialogue between the laboratory bench and computational algorithms, where each cycle of feedback sharpens AI’s focus and enhances its predictive power. For instance, data from a failed compound can reveal as much about target pathways as a successful one, teaching the AI to navigate the chemical space with greater discernment.
IMPROVING PROGRAM SUCCESS WITH DATA-DRIVEN DECISIONS
The ultimate goal of marrying AI with experimental data is to elevate the success rates of drug discovery programs, a long-standing sore spot in this industry. By leveraging AI’s analytical prowess, researchers can identify the most promising compounds early in the discovery process, prioritize them for development, and anticipate potential challenges in safety and efficacy. This approach not only accelerates the pace of discovery, but also allows resources to be allocated more effectively, focusing the attention of sponsors on candidates with the highest likelihood of clinical success.
REAL-WORLD APPLICATIONS
Logica’s approach exemplifies the power of this synergy. By integrating vast datasets from Charles River’s experimental platforms with Valo Health’s AI capabilities, Logica is equipped to develop models that accurately predict the outcomes of drug discovery endeavors. For example, AI can be used to model the pharmacodynamics of a new oncology drug, using data from early stage experiments. The model’s predictions can then be used by team members to adjust the compound’s structure, enhancing its efficacy, and reducing off-target effects, thereby streamlining the path to preclinical trials. This iterative, data-driven process is built to accelerate development cycles and increase the likelihood of clinical success — the collective goal of our industry. This approach represents a paradigm shift in how we understand and apply the principles of pharmacology and drug design, marking a new era of precision and efficiency in the quest to bring potentially lifesaving treatments to market.
ENGINEERING THE FUTURE: AI-DESIGNED MOLECULES & BEYOND
The future of drug discovery is being reshaped by the convergence of AI and experimental data, a partnership that promises to revolutionize how we design molecules and approach therapeutic challenges.5 As we look ahead, AI’s role in drug discovery is poised to transition from an auxiliary tool to a central figure in the design, testing, and optimization of new compounds. This shift is predicated on AI’s ability to process vast datasets, drawing insights that would be unattainable to human researchers alone. The integration of AI with cutting-edge experimental techniques will enable the design of molecules with unprecedented specificity and efficacy, reducing the time and cost associated with bringing new drugs to market.
ENHANCED PREDICTIVE MODELS & PERSONALIZED MEDICINE
The evolution of AI models, fueled by richer and more comprehensive datasets, will lead to more accurate predictions of drug behavior and interactions within the human body. This advancement holds the key to personalized medicine, where treatments can be tailored to the genetic makeup of individual patients.6 AI’s ability to sift through genetic information and correlate it with drug responses will open new avenues for customized therapies, making treatments more effective and reducing the incidence of adverse reactions.
NAVIGATING THE COMPLEXITY OF BIOLOGICAL SYSTEMS
As AI technologies mature, their application will extend beyond traditional drug design to explore complex biological systems and disease pathways. AI models will be instrumental in uncovering novel targets and understanding the multifaceted nature of diseases like oncology, CNS, cardiovascular, and autoimmune disorders, to name a few. By simulating the interactions within these systems, AI will identify new therapeutic opportunities and guide the development of drugs that can modulate disease processes more effectively.
THE GROWING SYNERGY OF AI & EXPERIMENTAL VALIDATION
The future will also see a deeper integration of AI with experimental validation, creating a seamless feedback loop that accelerates the discovery process. This synergy will ensure that AI-designed molecules are not only theoretically effective but also validated through empirical data. The continuous exchange of data between AI models and laboratory results will refine AI predictions, making the drug discovery process more efficient and reliable.
Additionally, this integration will facilitate the development of more sophisticated AI algorithms, thereby enhancing the predictive accuracy for drug efficacy and safety profiles before clinical trials commence. Furthermore, the democratization of drug discovery through AI enables all levels of research to leverage cutting-edge research tools, broadening the scope of innovation and potentially reducing the time and cost to market for new treatments.
SUMMARIZING WHAT’S TO COME
The horizon for AI in drug discovery is expansive, promising to streamline the discovery of new therapies and usher in an era of personalized, effective, and accessible treatments. As AI and experimental science fuse, the drug discovery landscape will be transformed, with Logica fully equipped and focused on leading the charge toward this exciting future. This shift is expected to accelerate innovation, diversify the pipeline of drug candidates, and potentially reduce the overall cost of bringing new treatments to market, marking a significant step forward in making advanced drug discovery tools accessible to a broader range of researchers and companies.
LOGICA: LEADING THE AI REVOLUTION IN DRUG DISCOVERY
Logica, a collaboration between Charles River and Valo Health, is an example that exemplifies the possibilities for AI’s integration into drug discovery. Under a team of drug discovery veterans, Logica is pioneering a new approach. By marrying AI with “wet science” data, Logica is not only accelerating the discovery process, but also intends to bolster the success rates of advanceable drug leads.
AI in drug discovery represents a paradigm shift, not just in how we approach the science of finding new medicines but in how we envision the future of pharmaceutical research. At Logica, we’re not just using AI; we’re integrating it with the invaluable insights provided by wet lab data to create a seamless, iterative process. This approach isn’t engineered to just speed up discovery; it’s designed to enrich it, ensuring that every step of the process is informed by the most accurate, up-to-date data available. We’re excited for what’s becoming possible in drug discovery — and we’re excited to be part of proving that when AI and human expertise combine, the potential is limitless.
EMBRACING THE AI REVOLUTION: A NEW ERA IN DRUG DISCOVERY
As we stand on the brink of a new era in drug discovery, the integration of artificial intelligence (AI) with experimental data heralds a transformative shift in how we approach the creation of new medicines. This revolution, characterized by a seamless blend of computational power and biological insight, is not merely about enhancing the efficiency of drug discovery processes; it’s about fundamentally redefining what is possible in the quest to treat and cure diseases. The burgeoning field of digital biology, powered by AI, is poised to turn biology into an engineering discipline, opening unprecedented opportunities for drug discovery.7 Furthermore, the rapid advancement of AI-designed drugs through both the discovery and preclinical stages, as demonstrated by recent clinical trials, underscores AI’s potential to significantly accelerate the drug development process. Logica, through its pioneering efforts, exemplifies the potential of this synergy, driving forward with innovations that promise to reshape the landscape of pharmaceutical research and development.
The journey of AI in drug discovery, from its nascent integration to becoming an indispensable tool, illustrates a path filled with challenges, learning, and ultimately, immense rewards. The ability of AI to sift through and make sense of vast datasets, to predict outcomes with increasing accuracy, and to uncover insights that elude human cognition, is a testament to the power of this technology. Yet, it is the marriage of AI with the rich, nuanced data from experimental research that truly unlocks its potential. This partnership does not replace the human element but rather enhances it, allowing scientists to explore new hypotheses, to test the boundaries of current knowledge, and to accelerate the pace at which new treatments can be developed and brought to market.
Looking to the future, the promise of AI in drug discovery is boundless. With each advancement in AI technology and each new dataset generated from experimental research, we edge closer to a world where drug discovery is more predictive, personalized, and potent. The role of initiatives like Logica becomes increasingly crucial as we navigate this uncharted territory, blending the art of science with the precision of algorithms to forge new paths in healthcare. As we embrace the AI revolution, we do so with the knowledge that we are not just witnessing a change in how we discover drugs but are participating in a historic moment that will define the future of medicine.
REFERENCES
- Fleming, N. How artificial intelligence is changing drug discovery. Nature. Published 2018 May 30.
- Ayers, M., et al. Adopting AI in Drug Discovery. Boston Consulting Group. Published 2022 March 29.
- Blanco-Gonzalez, A., et al. The Role of AI in Drug Discovery: Challenges, Opportunities, and Strategies. Pharmaceuticals. Published 2023 June 18. 18;16(6):891.
- Barzilay, R., et al. The race for a cure. Royal Society of Chemistry. Published 2020 June 3.
- Jiménez-Luna, J., et al. Artificial intelligence in drug discovery: recent advances and future perspectives. Expert Opinion on Drug Discovery. Published 2021 April 2.
- Khatami, S.G., et al. Using predictive machine learning models for drug response simulation by calibrating patient-specific pathway signatures. Nature. Published 2021 October 27.
- Regalado, A. An AI-driven “factory of drugs” claims to have hit a big milestone. MIT Technology Review. Published 2024 March 20.
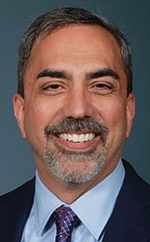
Dr. Emilio Cordova, Executive Director of Logica, Charles River, has served in this position since June 2023. Prior to his appointment at Logica, he was CEO of SAMDI Tech, Inc. for 8 years, culminating in the successful acquisition by Charles River in 2023. He brings over 25 years of management and executive experience in contract research. Throughout his career, he held leadership positions in sales, marketing, and business development including positions at Worldwide Clinical Trials, Bioanalytical Systems, Inc., and AIT Bioscience. He earned his Ph.D. from the University of Miami and his MBA from Purdue University’s Krannert School of Management. In addition, he completed an NIH post-doctoral fellow appointment at Harvard University under the guidance of Dr. George Whitesides.
Total Page Views: 3630