Issue:October 2024
ARTIFICIAL INTELLIGENCE - Deciding Whether to Automate With AI? 6 Key Practices to Consider
INTRODUCTION
It seems artificial intelligence (AI) has permeated industries of all kinds, and its rapid evolution has been nothing short of revolutionary. The data supports this bold assertion, as PwC’s 2023 Emerging Technology Survey finds 55% of respondents say their company has invested in AI, and that it was among the top three investment priorities in the past 12 months, more than any other technology. A McKinsey Survey finds that AI is having a “breakout year” and the use of Generative AI is already widespread, as 79% say they have had some exposure to AI at work.
The life sciences industry has also been exploring using AI, as it has the potential to be incredibly beneficial, but it should be approached with care. Research from the National Institutes of Health cite both the drawbacks and potential of AI in healthcare. The potential lies in making healthcare more personalized, predictive, preventative, and interactive, while raising concerns about data security and privacy, as hackers often target medical records during data breaches.
Another challenge is communicating and translating complex health information and science jargon, as this requires a specific skillset, because if the information is not correct, it could have dire health consequences for patients. As AI translation gets better, studies show, cultural bias can arise due to an imbalance of training data that overrepresents certain languages or dialects, cultural contexts, or historical biases. This can result in misunderstandings and misinterpretations. Also, without accurate and relatable translations, there is a risk of introducing errors by those who manage clinical trials in diverse site locations.
In addition, patients seeking information may get steered the wrong way if they ask “Dr. Google” for help. Not only will some people find information that is complex and likely meant for a different audience, but they could easily find an unreliable source that contributes to health misinformation. This problem is magnified when people speaking a language other than English would benefit from health information provided in their native language. A recent study from the University of Manchester (UK) found that poorly translated materials related to a commonly given cognitive test had spelling errors, and one out of four questions required cultural adaptation to make them understandable in daily use by native speakers. This highlights the need to ensure translation of health materials is prioritized and carefully crafted.
6 BEST PRACTICES TO CONSIDER BEFORE DECIDING ON AUTOMATION
Before deciding if or how to use AI in life sciences, it’s critical to weigh the pros and cons. There is a vital need to customize constantly evolving AI applications and innovations to create tailored, effective technologies that reflect life science organizations’ regulatory and organizational frameworks. Ensuring health literacy and equity relies on providing accurate, culturally relevant materials that empower patients to make informed decisions about their healthcare in collaboration with their providers. While AI can enhance efficiency in automating repetitive tasks, maintaining a human element can be more difficult to achieve, and why it is crucial for actual translators to produce culturally sensitive and accurate materials. Here are 6 key best practices to consider before deciding to automate or not:
1. Validation & Regulation
It’s important to validate AI models through testing and compare them with existing models to ensure reliability and effectiveness. Generative AI is still susceptible to “hallucinations” and many AI models are trained on large amounts of public data that contain stereotypes, biases, and prejudices. If the data is a few years old, it may not reflect recent societal efforts to be more inclusive. Problems could include:
Sampling Bias: when some populations are either overrepresented or underrepresented.
Labeling Bias: when humans perpetuate their own bias into labeling or classifying data. Algorithmic Discrimination: embedding bias in the optimization processes applied during training, making the software itself biased.
Contextual Bias: a lack of understanding of the larger social and cultural contexts.
Cyclical Bias (or self-reinforcing bias): when an AI system is used in a real-world setting and its decision impacts other data collections.
Gender Bias: MIT researchers examined gender bias in machine learning, revealing that AI can perpetuate harmful biases. For instance, words such as “anxious” and “depressed” are often categorized as feminine. Additionally, translating gender-neutral language poses challenges, as AI frequently resorts to guessing genders. This can result in stereotyping male roles like doctor, and professions like nurse to be associated with females.
Racial Bias: Racial bias in algorithms can result in racial or ethnic minorities needing to be more severely ill to receive equal diagnosis, treatment, or resources compared to white patients. To address this issue, an expert panel assembled by the Agency for Healthcare Research and Quality and the National Institute on Minority Health and Health Disparities has recently proposed guidelines to reduce bias in healthcare AI.
According to a recent article from the law firm ArentFox Schiff, there are several main areas where rigorous AI oversight is needed in the life sciences industry, including drug discovery and development. This article explains how the use of AI in drug discovery can raise legal questions regarding patent eligibility, ownership of AI-generated inventions, and potential liability issues in cases of adverse effects from AI-generated drugs. Regulation of this process is needed along with establishing guidelines.
The Tufts Center for the Study of Drug Development and the Drug Information Association collaborated with 8 pharmaceutical and biotechnology companies on a study which examined the adoption and impact of AI on drug development. Although 7 in 10 respondents said they used AI in some capacity, a broad range of use was reported by AI type. Patient selection and recruitment for clinical studies was the most common AI activity. The study also found that AI use has been prevalent in drug discovery, as many companies have set up in-house initiatives or partnerships with AI companies. Some of these companies are now using AI approaches for repurposing drugs and finding new uses for existing drugs or late-stage drug candidates. Another way companies are using AI is for phenotypic drug discovery “in which compounds are screened in cells or animal models for compounds able to cause a desirable change, without knowledge of the biological target.”
Given the increasing presence of AI in the healthcare industry, regulatory agencies are in the process of creating guidelines as they increasingly recognize the potential benefits of AI in improving health outcomes, which is why life science companies need to stay on top of guidance. The Department of Health and Human Services (HHS) has been at the forefront of most AI regulatory activities, but other government agencies, like the US FDA, are also becoming involved due to AI’s impact in healthcare. The Bipartisan Policy Center issued a brief detailing the current regulatory landscape for AI. It provides insights into how regulatory agencies are approaching AI regulations, and what guidelines or recommendations they are considering.
2. Data Quality/Privacy
Ensure that the data to train AI models is of the highest quality and represents the populations it’s meant to serve. Prioritize patient privacy and comply with relevant regulations such as GDPR or HIPAA. Trained linguists can play a vital role in post-editing AI translations to ensure accuracy, data quality, and cultural sensitivity. They can also identify and fix biases in the data, retrain data sets with revised translations, and annotate or tag data to help AI adapt its results based on factors such as culture, race, and gender. Over time, this continuous improvement process can lead to fewer fixes and more accurate translations. Given that nearly 1 in 5 Americans speak a language other than English at home, it’s clear that incorporating language considerations into the clinical and technology workflow needs to start before the patient seeks care.
3. Establish Automated Quality Checks
These checks can include linguistic analysis tools that compare AI translations to human translations, as well as algorithms that detect patterns of bias in the data. By integrating automated quality checks into the translation process, businesses can ensure AI translations meet the highest standards of accuracy and cultural sensitivity. AI models should also be interpretable, so that their decision can be understood by experts. Transparency in the AI development process, including the data and the model’s decision-making process, is important to build trust and understanding.
4. Foster Collaboration & Ethical Considerations
AI developers, healthcare professionals, and regulators need to work together to ensure AI solutions meet real-world needs and are implemented ethically. In an article written for BMC Medical Ethics, an “embedded ethics” approach was recommended, in which ethicists and developers address issues together on a continuous basis, as an effective way to inject ethical considerations into the development of medical AI.
5. Custom-Designed Tools
By customizing AI tools that incorporate industry-specific terminology and cultural nuances, businesses can reduce bias in AI while improving delivery of accurate communication. Training LLM datasets with these elements (or prompting a GenAI review tool to take them into consideration) allows you to be more efficient without sacrificing your intended message.
6. Risk Management Policies
Regulations around AI are constantly evolving, and due to the potential impact to patient safety, regulated (GxP) AI usage must be thoroughly evaluated for risks. Following the Guidance on the Application of ISO 14971 to Artificial Intelligence and Machine Learning or the Actions to Address Risks and Opportunities elements of ISO 42001, organizations can implement effective AI strategies while taking into consideration key risks – including bias, data security, and patient safety.
“Artificial Intelligence (Al) can bring benefits to healthcare – improved clinical outcomes, improved efficiencies, and improvement in the management of healthcare itself. However, the implementation of new technologies such as Al can also present risks that could jeopardize patient health and safety, increase inequalities and inefficiencies, undermine trust in healthcare, and adversely impact the management of healthcare.” – Guidance on the Application of ISO 14971 to Artificial Intelligence and Machine Learning
Although AI offers the potential to revolutionize healthcare, its deployment should be deliberate and well-planned. By blending AI’s efficiency with human supervision, organizations can unlock AI’s full capabilities in enhancing healthcare results and advancing health equity.
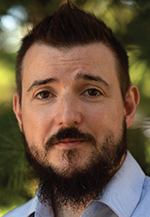
Dan Milczarski is the Chief Technology Officer at CQ fluency. Connect. Automate. Innovate — those are the pillars he and his team focus on to establish CQ fluency as a top-tier, tech-enabled language service provider. Focusing on process automation, custom development solutions using AI and machine learning, his team of solution architects, engineers, developers, and product support specialists ensure CQ fluency customers have the best language technology in place to help them be more efficient.
Total Page Views: 3059