Issue:October 2018
ARTIFICIAL INTELLIGENCE - 3Ds Powering AI in Drug Discovery – Domain Expertise, Deep Learning & Data
ABSTRACT
Artificial intelligence is attracting significant attention and investment for drug discovery. At least 97 relevant start-ups and 28 pharmaceutical companies use AI in some way. But not all applications are equal. Companies that combine domain expertise, deep learning, and proprietary data stand apart. In this article, we explore this combination in further detail. We counter skepticism about AI with concrete data on recent exponential progress. We provide examples of companies applying these advances to drug discovery. And we show how those combining domain expertise, deep learning, and proprietary data are excelling.
INTRODUCTION
In December 2017, a start-up of about 20 people did something unusual in drug discovery. After 15 months of starting a project, it was preparing a Phase 2a clinical trial.1 This after identifying lead candidates, completing preclinical validation, and submitting a publication. Even more shocking: its budget was $100,000.2
The start-up, Healx (pronounced heal-ix), owes its efficiency to artificial intelligence (AI). It uses AI to repurpose and combine existing drugs to treat rare diseases. For this project, it focused on fragile X. It’s the most common inherited cause of autism and learning disabilities. Affecting 1 in 4,000 males and 1 in 8,000 females, it’s well-researched. But it still has no effective treatments. Thanks to Healx, it may soon. And more diseases could follow.
Healx demonstrates the power of combining domain expertise, deep learning, and proprietary data. The result is a scalable platform for drug discovery. But the model isn’t confined to a single start-up, or start-ups in general. The “3D” approach is powering a new wave of AI-driven R&D. One that might (finally) achieve time- and cost-savings from the technology that the industry seeks.
HAVEN’T WE HEARD THIS ALL BEFORE?
To skeptics, this might sound like familiar AI hype. After all, the field has promised much since the 1950s. “We think that a significant advance can be made,” wrote leading computer scientists in 1955 when proposing the first workshop on AI (a term they coined to describe the field), “if a carefully selected group of scientists work on it together for a summer.”3 A summer!
The exuberance earned the field funding in the 1960s. But disillusionment set in when the hype went unfulfilled. In the 1970s, funding dried up, beginning a so-called “AI winter.” A shift to work on narrow, brittle rules-based expert systems dominated the 1980s. A few pioneers of today’s neural networks persisted in more ambitious work, but at the margins.
In 1997, IBM rekindled public interest in AI with Deep Blue’s victory over Garry Kasparov.4 The media amplified the excitement, with headlines such as “The Brain’s Last Stand.”5 But critics labeled it a victory of brute force computation, not intelligence.6 The hype again deflated.
Beginning in the mid-2000s, public excitement swelled again. And this time, with more unequivocal success. In 2005, five robot cars completed DARPA’s Grand Challenge after none did the year before (setting the stage for the coming self-driving car revolution).7 The success came in part from advances in machine learning.
In 2011, IBM once again stoked people’s hopes (and fears) about the potential of AI.8 Its Watson system combined natural language processing algorithms to answer questions on Jeopardy! and beat the world’s top players. Watson needed 90 servers to do it.9 But unlike with Deep Blue, fewer critics denied it some claim on some form of intelligence.
But IBM’s dominance of AI news was short-lived. In 2012, University of Toronto researchers changed the conversation — and the dominant algorithms.10 Competing on a benchmark image classification task, their “AlexNet” system achieved unprecedented improvement. It did so using layers of connected artificial neurons running on graphics processing units (GPUs). Once confined to the margins, this approach, called deep learning, took center stage.
EXPONENTIAL GROWTH IN PERFORMANCE & PAPERS
Since then, progress has been exponential. In 2016, Google’s DeepMind used deep learning in AlphaGo to beat top player Lee Sedol at Go.11 A year later, it unveiled AlphaGo Zero, which taught itself to play Go, and beat AlphaGo.12 Another variant, AlphaZero, beat a top-ranked chess program.13 Processing power used in AlphaGo Zero was 300,000 times greater than in AlexNet.14 It doubles every 3.5 months for the largest neural networks.15 Performance on tough tasks such as image classification is keeping pace. Machines went from subhuman to superhuman in 5 years.16
This time, the hype seems justified. And drug discovery isn’t immune. In fact, there have been more papers on AI in drug discovery this decade than all prior years combined.17 As recently as 1997, there were 36. Last year, there were 552, a greater than 15x annual increase in a decade.
And the advances aren’t only in research. Start-ups and established companies are commercializing them. By our count, there are now at least 97 start-ups using AI for drug discovery.18 We have also identified 28 pharmaceutical companies that have disclosed using the technology.19
Nor is this all vaporware. In addition to Healx, other companies have now progressed treatments discovered in silico. Companies with AI-driven therapies in trial include BenevolentAI, Berg, BioXcel, Lantern, and Recursion.20-24 Many others have licensed promising compounds to pharma partners for development.
COMPLEMENTING ALGORITHMS WITH SPECIALIZED DATA & KNOWLEDGE
Deep learning is driving this process, but not alone. For one thing, deep learning benefits from lots of data. The benchmark database for image classification, for example, contains more than 14 million images.25 Whereas a human child can point to a cat after seeing one example, today’s machines cannot. They need thousands. For specialized applications, this data often doesn’t exist. And if it does, often not in a format that’s good for machine learning. Today’s AI-driven drug discovery start-ups wouldn’t be possible without specialized data. So they’ve invested in amassing it.
Along with deep learning and data, domain expertise has been essential. Technology start-ups whose leaders lack healthcare experience tend to underestimate their challenges.26 These include not only technical hurdles, but also regulatory and cultural hurdles. As start-ups get more specialized, their need for specialized domain expertise does too. “Healthcare” is too general a domain if your focus is oncology. Oncology is too general a domain if your focus is immuno-oncology. And on it goes.
Healx is a great example. Its domain is repurposing and combining drugs for rare diseases. Expertise in this domain comes from leadership including drug discovery veteran Dr. David Brown, who is the co-inventor of Viagra, one of the most successful repurposed drugs of all time.27 Deep learning powers Healx’s platform, HealNet, which predicts novel disease-drug relationships.28 Data comes from public sources, such as research papers and clinical trials. But also proprietary sources, such as rare disease advocacy groups.29 Combined, Healx’s domain expertise, deep learning technology, and data assets have proven potent. In July 2018, investors recognized its potential with a $10-million investment.30
But Healx is far from alone. The trend amongst start-ups using AI for drug discovery is to increase specialization. In April, for example, MIT spin-out ReviveMed received $1.5 million in seed funding.31 Its focus is metabolomics. Its founder is a domain expert, computational biologist Leila Pirhaji. Its competitive advantage is a proprietary metabolomics database and partnerships to grow it. Another example is CytoReason, whose founder is Shai Shen-Orr, a leader in the field.32 Its focus is systems immunology, and its competitive advantage is a proprietary dataset including experimental data. The list goes on. Envisagenics for RNA.33 BioAge for aging.34 The future isn’t IBM Watson designing everyone’s drugs. It’s the combination of specific expertise, technology, and data to solve specific problems.
A MANDATE FOR SUCCESS IN HEALTHCARE
Of course, if you’re familiar with the concept of the “long tail,” none of this should be surprising.35 Low-cost digital technology is famous for allowing niche businesses to thrive. But there’s more to this story.
Combining domain expertise, deep learning, and data isn’t only trendy. It’s a mandate. Without domain expertise, companies suffer. They don’t understand the problem space or the drug discovery process. And they can be overconfident in technology’s power to overcome non-technical challenges. This includes regulatory hurdles, privacy constraints, and the often slow pace of healthcare.
Without deep learning, it’s too hard to extract value from large biological datasets. The larger and more feature-rich they are, the harder it gets.
And without such data, you can’t maximize deep learning’s potential. It’s like filling a swimming pool with a few inches of water. Proprietary data is particularly important. Processing power and data storage are commodities. Software libraries for deep learning are free and open source. Large, free biology datasets are downloadable for all. Proprietary data is key to building and sustaining a competitive advantage. We learned all of this first-hand early in 2018.
OUR JOURNEY FROM THE LAB TO A GOOGLE INVESTMENT
At BenchSci, we decode the world’s biological data to reduce the time, uncertainty, and cost of biomedical research. We use machine learning to read scientific papers and extract data on reagent use. Researchers can search BenchSci instead of PubMed when selecting reagents. Rather than using keywords, due to machine learning, they can search by protein or gene. And then they can filter by technique, organism, tissue, and 13 other options. Using BenchSci, researchers can select antibodies up to 24x faster. And they can shave months off an R&D project’s timeline.
But as we learned, such product-market fit is only the starting point for investors. In early 2018, we met with 30 venture capital firms to raise a series A investment. These days, they see a lot of AI companies. And their feedback was unanimous. If an AI start-up has technical expertise but no domain expertise, they should get it. If they use machine learning but not advances in deep learning, they should apply them. And if they use only public data, they should develop proprietary sources. And, for good measure, a data feedback loop: using data to build a product that gathers data. Only then do they have a shot at big success.
We were lucky to pass these tests. Our CSO, Tom Leung, felt the pain of selecting reagents doing cancer research. He and our science team bring domain expertise. Our CTO, David Chen, applies his neuroscience background to develop machine learning advances. He and his team provide the deep learning. And our data comes from public sources, vendors, closed-access publications, and platform use. There’s the proprietary data, and a data feedback loop for good measure. Only by clearing the hurdles could we earn investment. This included investment from leaders in AI, such as Google’s AI-focused venture fund.36
PHARMA COMPANIES ARE CATCHING ON
But start-ups and investors aren’t the only ones to have noted the importance of these factors. So have the world’s largest pharmaceutical companies. Now they’re reorganizing their business around domain expertise, data, and deep learning too.
Novartis CEO Vas Narasimhan, for example, is remaking the company around “medicines and data science.”37 Novartis restructured its Global Drug Development (GDD) IT infrastructure, connecting disparate datasets. This includes 20 years worth of data from clinical trials. With the first phase of its project complete, it’s now looking to apply machine learning. “What if we were to combine all our data sets together, access the data, and make it specific to disease areas so that scientists can ask the questions they weren’t able to ask before?” said Achim Plueckebaum, Global Head of Drug Development IT, in a recent interview.38 Jay Bradner, Head of the Novartis Institutes for BioMedical Research (NIBR), leads a similar charge.39 He reports that 4% of NIBR’s 6,000 scientists are now data scientists.40
GSK is undertaking a similar revamp.41 John Baldoni, SVP, Head of In-Silico Drug Discovery Unit, has a rallying cry. He wants to go from target to treatment in 12 months (versus 6 years). Once again, domain expertise, data, and deep learning play a central role. GSK hired Chief Data Officer Mark Ramsey from Samsung. He led the consolidation of 2,500 distinct structured data sources. And GSK established partnerships with start-ups that leverage deep learning in different domains. Several intend to find new uses for GSK’s now-consolidated proprietary data.
SUMMARY
So, whether you work in a start-up or a large pharmaceutical company, the trend seems clear. This time, the hype over AI seems justified. But not everyone will reap equal benefits. Emerging winners have a few things in common. They focus on specific applications and are experts in their domain. They apply deep learning advances to extract value from large, feature rich datasets. And they develop proprietary, domain-relevant datasets, often with feedback loops.
If this sounds like your company, you have a good shot at success. If not, get inspired! Researchers are already using AI to develop drugs in months, not decades. For hundreds of thousands of dollars, not billions. And with AI, progress is exponential. Processing power for the largest neural networks will double in 3.5 months. So what are you waiting for?
REFERENCES
- Fragile X case study. Healx. https://healx.io/our-work/fragile-x-case-study/. Accessed August 13, 2018.
- Smith S. Tim Guilliams and David Brown, Healx, AI for Rare Diseases. Artificial Intelligence in Drug Discovery. https://anchor.fm/artificial-intelligence-indrug-discovery/episodes/Tim-Guilliamsand-David-Brown–Healx–AI-for-Rare-Diseases-e1snm7. Published July 26, 2018. Accessed August 13, 2018.
- McCarthy J, Minsky ML, Rochester N, Shannon, CE. A Proposal for the Dartmouth Summer Research Project on Artificial Intelligence. http://www-formal.stanford.edu/jmc/history/dartmouth/dartmouth.html. Published August 31, 1955. Accessed August 13, 2018.
- Weber B. Swift and Slashing, Computer Topples Kasparov. The New York Times. https://www.nytimes.com/1997/05/12/nyregion/swift-and-slashing-computer-topples-kasparov.html. Published May 12, 1997. Accessed August 13, 2018.
- Achenbach J. In Chess Battle, Only the Human Has His Wits About Him. The Washington Post. http://www.washingtonpost.com/wp-srv/tech/analysis/kasparov/post3.htm. Published May 10, 1997. Accessed August 13, 2018.
- Gardner M. Those Mindless Machines. The Washington Post. https://www.washingtonpost.com/archive/opinions/1997/05/25/those-mindless-machines/228695c9-10c2-4d5d-8179-6dc4d8e04926/. Published May 25, 1997. Accessed August 13, 2018.
- Russell S. DARPA Grand Challenge Winner: Stanley the Robot!. Popular Mechanics. https://www.popularmechanics.com/technology/robots/a393/2169012/. Published January 8, 2006. Accessed August 13, 2018.
- Gabbatt A. IBM computer Watson wins Jeopardy clash. The Guardian. https://www.theguardian.com/technology/2011/feb/17/ibm-computer-watsonwins-jeopardy. Published February 17, 2011. Accessed August 13, 2018.
- Leb-Ram M. IBM’s Watson is changing careers. Fortune. http://fortune.com/2012/02/03/ibmswatson-is-changing-careers/. Published February 3, 2012. Accessed August 13, 2018.
- Krizhevsky A, Sutskever I, Hinton GE. ImageNet Classification with Deep Convolutional Neural Networks. Advances in Neural Information Processing Systems 25 (NIPS 2012). http://papers.nips.cc/paper/4824-imagenet-classification-with-deep-convolutional-neural-networks.pdf. Published 2012. Accessed August 13, 2018.
- Metz C. Google’s AI Wins Fifth and Final Game Against Go Genius Lee Sedol. Wired. https://www.wired.com/2016/03/googles-ai-wins-fifth-final-game-go-genius-leesedol/. Published March 15, 2016. Accessed August 13, 2018.
- Greenemeier L. AI versus AI: Self-Taught AlphaGo Zero Vanquishes Its Predecessor. Scientific American. https://www.scientificamerican.com/article/ai-versus-ai-self-taught-alphago-zero-vanquishes-its-predecessor/. Published October 18, 2017. Accessed August 13, 2018.
- Silver D, Hubert T, Schrittwieser J, et al. Mastering Chess and Shogi by Self-Play with a General Reinforcement Learning Algorithm. arXiv.org. https://arxiv.org/abs/1712.01815. Published December 5, 2017. Accessed August 13, 2018.
- AI and Compute. OpenAI Blog. https://blog.openai.com/ai-and-compute/. Published May 16, 2018. Accessed August 13, 2018.
- AI and Compute. OpenAI Blog. https://blog.openai.com/ai-and-compute/. Published May 16, 2018. Accessed August 13, 2018.
- 2017 Annual Report. Artificial Intelligence Index. http://cdn.aiindex.org/2017-report.pdf. Published November 2017. Accessed August 13, 2018.
- PubMed Search. https://www.ncbi.nlm.nih.gov/pubmed/?term=(%22artificial+intelligence%22+or%22machine+learning%22+or+%22deep+learning%22+or+%22neural+networ%22)+and+(drug+or+drugs). Accessed August 13, 2018.
- Smith S. 97 Startups Using Artificial Intelligence in Drug Discovery. BenchSci Blog. https://blog.benchsci.com/startups-usingartificial-intelligence-in-drug-discovery. Published August 7, 2018 (last updated). Accessed August 13, 2018.
- Smith S. 28 Pharma Companies Using Artificial Intelligence in Drug Discovery. BenchSci Blog. https://blog.benchsci.com/pharma-companies-using-artificial-intelligence-in-drugdiscovery. Published August 2, 2018 (last updated). Accessed August 13, 2018.
- BEN-2001 in Parkinson Disease Patients With Excessive Daytime Sleepiness (CASPAR). ClinicalTrials.gov. https://clinicaltrials.gov/ct2/show/NCT03194217. Published June 21, 2017. Accessed August 13, 2018.https://clinicaltrials.gov/ct2/show/NCT03194217.
- Berg Health Pipeline. Berg Health. https://berghealth.com/pipeline/. Accessed August 13. 2018.
- BioXcel Therapeutics Pipeline. BioXcel Therapeutics. http://www.bioxceltherapeutics.com/pipeline.php. Accessed August 13, 2018.
- Lantern Pharma Pipeline. Lantern Pharma. http://www.lanternpharma.com/pipelinenew/. Accessed August 13, 2018.
- Recursion Pharmaceuticals Pipeline. Recursion Pharmaceuticals. https://www.recursionpharma.com/pipeline/. Accessed August 13, 2018.
- Summary and Statistics. ImageNet. http://image-net.org/about-stats. Published April 30, 2010 (last updated). Accessed August 13, 2018.
- Lowe, D. The Case of Verge Genomics. In the Pipeline. http://blogs.sciencemag.org/pipeline/archives/2018/07/17/the-case-of-vergegenomics. Published July 17, 2018. Accessed August 13, 2018.
- About Healx. Healx. https://healx.io/about/#team. Accessed August 13, 2018.
- HealNet. Healx. https://healx.io/technology/. Accessed August 13, 2018.
- Because every rare disease patient deserves a treatment. Healx. https://healx.io/patient-groups/. Accessed August 13, 2018.
- Healx raises $10m as investors back AI to find rare disease treatments faster. Healx. https://healx.io/news/healxraises-10m-as-investors-back-ai-to-find-raredisease-treatments-faster/. Published July 26, 2018. Accessed August 13, 2018.
- MIT Spinout, ReviveMed, Raises $1.5M to Advance its AI-Driven Metabolomic Platform for Drug Discovery. ReviveMed. http://www.revivemed.com/2018/04/18/seed-fund-pressrelease/. Published April 18, 2018. Accessed August 13, 2018.
- Home Page. CytoReason. http://www.cytoreason.com/. Accessed August 13, 2018.
- Home Page. Envisagenics. https://www.envisagenics.com/. Accessed August 13, 2018.
- Home Page. BioAge Labs. http://bioagelabs.com/. Accessed August 13, 2018.
- Anderson, A. The Long Tail. Wired. https://www.wired.com/2004/10/tail/. Published October 1, 2004. Accessed August 13, 2018.
- O’Kane, J. Alphabet’s AI venture capital firm makes first investment in Canada with BenchSci. The Globe and Mail. https://www.theglobeandmail.com/business/article-alphabets-ai-venture-capitalfirm-makes-first-investment-in-canada/. Published May 2, 2018. Accessed August 13, 2018.
- Narasimhan, V. Reimagining Novartis as a ‘medicines and data science’ company. LinkedIn Pulse. https://www.linkedin.com/pulse/reimagining-novartis-medicines-data-science-company-vas-narasimhan/. Published January 12, 2018. Accessed August 13, 2018.
- Davis, J. Novartis Seeks Hidden Cures in Machine Learning, AI. InformationWeek. https://www.informationweek.com/bigdata/big-data-analytics/novartis-seekshidden-cures-in-machine-learning-ai/d/d-id/1332269. Published July 11, 2018. Accessed August 13, 2018.
- McConaghie, A. Jay Bradner: leading Novartis into age of digitally-enabled discovery. PharmaPhorum. https://pharmaphorum.com/views-analysis-digital/jay-bradner-leading-novartisage-digitally-enabled-discovery/. Accessed August 13, 2018.
- Ramsey, L. “We like to think of ourselves as the lead turtle in the race of the turtles”: How Big Pharma is turning to Silicon Valley to supercharge drug development. Business Insider. https://www.businessinsider.com/novartis-jay-bradner-on-silicon-valley-intersecting-with-pharma-2018-6. Accessed August 13, 2018.
- Smith, S. 6 Steps to AI Leadership in Pharma: An Interview with John Baldoni of GSK. LinkedIn Pulse. https://www.linkedin.com/pulse/6-stepsai-leadership-pharma-interview-john-baldoni-gsk-simon-smith/. Accessed August 13, 2018.
To view this issue and all back issues online, please visit www.drug-dev.com.
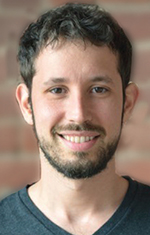
Liran Belenzon is Chief Executive Officer of BenchSci, which is decoding the world’s biological data to reduce the cost, time, trial and error, and redundancy of biomedical research. Prior to BenchSci, he co-founded Israel’s first B2B e-commerce marketplace. He also served as a commander in the Israeli Defence Forces. He is passionate about using data and algorithms to improve reproducibility, efficiency, and cost-effectiveness in drug discovery. Mr. Belenzon earned his MBA from the University of Toronto’s Rotman School of Management.
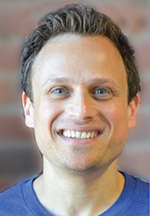
Simon Smith is Chief Growth Officer at BenchSci. Prior to BenchSci, he was SVP, Strategy at Klick Health, where he consulted on digital strategy for the commercialization of drugs in neurology, gastroenterology, endocrinology, and oncology. During this time, he led digital strategy for two US drug launches: a novel antidepressant, and a novel biologic for inflammatory bowel disease. Mr. Smith earned his undergraduate degree in Journalism from Ryerson University, and his Master of Arts in the History and Philosophy of Science and Technology from the University of Toronto.
Total Page Views: 8473