Issue:April 2024
ARTIFICIAL INTELLIGENCE - Embracing AI Requires Digital Literacy. How Will Your Organization Prepare?
INTRODUCTION
Artificial intelligence (AI) and machine learning (ML) present incredible business opportunities. But it’s not enough to say, “Our business will prioritize AI.” Often, a big gap exists between the technical expertise of scientific leaders on the one hand and the strategic expertise of business leaders on the other. These groups do not always speak the same language — they either do not understand the technical capabilities and limitations of new tools or they do not see how tools might effectively change the business. To be effective, both scientists and business leaders need a strong grounding in how data science can serve their goals.
Some big pharmaceutical companies have already recognized these challenges and instigated programs to support internal digital literacy around AI and ML. As these tools become more accessible for many organizations, it is time to embark on a learning and training journey.
Perhaps your organization already has exceptional processes in place around innovation, change management, and ongoing skill development. If not, building digital literacy around ML and AI is also a great opportunity to strengthen your training strategy.
The most crucial step in building an effective plan is to figure out who needs to know what, and when. Sure, training end-users is important — eventually. First, though, decision-makers need a clear understanding of the opportunities to set goals and priorities. Then, implementation leaders need enough information to vet tools and communicate their vision.
A strong training roadmap for digital literacy begins at the top, and it begins far in advance of any major AI or ML project rollouts.
DECISION-MAKERS
When the race is on, it can feel tempting to jump in and start making decisions. But to be effective, leaders need to be humble about what they know and don’t know. Ask: “Do I know enough yet to triage and prioritize where in my business this is going to make an impact?” And “Do I know enough to build teams around the most promising opportunities?” For most leadership teams, it’s time to skill up. Business leaders and technical stakeholders do not need PhDs in data science. But they do have to know enough to create logical priorities about where to invest first. Then, they need to bring their teams along with them. When preparing an organization to embrace AI, leaders should start with a few key considerations.
First, they should learn the broad strokes of what AI can and cannot do. Models are, simply put, prediction machines. Machine learning models and other tools can make predictions just as well as — and sometimes better than — humans, potentially leading to huge time savings. But while models predict, they do not promise; we can’t easily validate or regulate the information they produce. Human decision-making is still vital. Prediction Machines: The Simple Economics of Artificial Intelligence is one good introduction; MIT, Harvard, Carnegie Mellon, and other academic institutions also offer AI intensives for business leaders.1,2
To make AI useful in a pharmaceutical context, business leaders also need to understand the decisions their teams make every day, along with the information currently used to make those decisions. Would it help to make the same decisions, but cheaper and faster? Or is it more important to aim for better decisions, using data that teams can’t currently process? Opportunities and goals will vary across the drug discovery process. Pockets of the business are low risk, high reward: ML can have obvious wins for R&D and discovery. But because AI predictions are not traceable, the opportunities and risks are still not clear in more regulated stages of the biopharmaceutical lifecycle, like clinical trials and drug production.
In addition to identifying opportunities, leaders must also learn about the state of their data.3 To be useful, AI requires good data hygiene. With incorrect information, the wrong amount of information, or even the right information organized poorly, models will make bad predictions. AI models must be trained on experimental failures as well as successes; they also need well-labeled data in which the experimental context is clear and accessible. Frank Nestle, the Global Head of Research and Chief Scientific Officer of Sanofi made this point in a fireside chat at the BIO conference last June.4 He gave a 45-minute talk about all the amazing advances from AI in pharma. But at the end of his talk, he pointed out the key challenge: that AI won’t work nearly as well as it should until companies make deep investments in structured data. A major challenge today is that early adopters want to jump in and grab a tool and play with it. But to be successful, you must understand your organization’s data needs — and, likely, build serious infrastructure to make your data usable.
To assess opportunities and roadblocks, leaders need to do deep internal learning. Bring together the pockets of knowledge that already exist: Create a forum for your thought leaders to surface insights and help you develop guardrails. Pull in data science teams, IT business partners, and engineering organizations; get them talking with folks from Quality Assurance and Regulatory Affairs. Learning is collaborative: work together to identify how to strategically align your business for the opportunities and how to choose which opportunities will have the best return.
IMPLEMENTATION LEADERS
Many of the voices giving input into AI strategy will also be the people responsible for implementing the strategic decisions that come out of that initial learning journey. Once business leaders set a direction, implementation-level decision-makers will need to choose the right tools, vendors, and approaches to move the business forward. Many of these decision-makers will not be data scientists. They will also need to skill up — but maybe not quite as much as you might think. A biologist does not need to get a PhD in data science to weigh in on which tools might be the best fit for their part of the business.
For most companies, it makes much more sense to buy AI and data tools than to build them from scratch. Because AI is new and different, there is always the pressure to build internally if the right tool is not yet on the market. But this can involve regulatory risk and huge overheads.
We are at the beginning of a renaissance of low-code and no-code AI tools, as an evolving ecosystem of industry and academic collaborators bring new data science solutions to market. Unless the goal is to become an AI company first, the next step is usually to find the right partners to support your internal journey.
Tool-level decision-makers do not need the skills to build models in-house; they just need the skills to tell good from bad. It’s like driving a car. New drivers need to know the rules of the road. They also need to know enough to say, “That seems like a bad noise; probably time to take the car to the shop.” But they don’t need to know how to change the tires or change the oil. They don’t need to know how to build and design a car — just that it would be better to drive than to walk.
Likewise, people responsible for vetting new tools need to learn about the ecosystem of viable options, the problems their teams need to solve, and the state of their data. Hopefully, they have been at the table during earlier phases of the company’s AI learning journey, along with executives, and have that level of clarity.
Next, they can use the buying journey to learn more deeply. Talk with academics and potential partners to understand the key players and the lay of the land. Talk with peers about what has worked well for them. Test drive possible solutions alongside internal data scientists to flag potential implementation challenges and to iterate on RFPs as needed. Build enough competence to identify good from bad and to identify the partners that will be able to unlock the desired end state first.
Executives should also see this stage as a learning opportunity — and expect changes. Perhaps this deeper dive will uncover data connections that need to be built or goals that may need to be adjusted due to external limitations. Seen as setbacks, these discoveries can be disappointing. Seen as learning opportunities, they are a chance to solidify strategic priorities.
END USERS
Finally, once an initial ecosystem of homegrown and external tools has been identified, the next step is to train end users. At this stage, vendors will be a key resource. A vendor with experience successfully supporting organizations through digital maturity should be an excellent collaborator. Vendors can help set up architectures, think through user needs, and provide training resources for leveraging tools effectively. Interactive classroom training, on-demand training, train-the-trainer models, and custom solutions can all be part of the mix — both during the adoption phase and on an ongoing basis. A good partner will help scaffold learning, from initial change management to compliance training for core functionalities that are baked into your company’s regular training process.
But the most important step in end-user training is making sure end users understand the “why.” The biggest pitfall when implementing a new tool is often understanding the business case for that tool at the level of the user. End users need to be stakeholders; implementation by fiat will not support adoption.
Instead, embed the bigger vision and the potential business impact at every step in your change management roadmap, so that users who are being asked to make challenging changes can do so with a sense of purpose. Good external partners can help with this process by sharing examples of success stories that align with your goals. At IDBS, for example, we work closely with customers globally to support their data journeys, enabling more effective AI/ML investments. Through these interactions, we have learned how critical business, IT, and scientific stakeholder engagement is to overall project success.
Involve representative end users early in the company’s AI learning process. When employees are bought into why the outcomes of a new tool or new data hygiene process are crucial, it can make it easier to value things that don’t benefit their work directly.
The person using the tool isn’t always the person who realizes the value of that tool. Still, during and after the rollout, be genuinely open to end-user feedback. Ask: Where do we need to apply innovation? What do we need to change with partners? When end users simply need to do something unpleasant, are there ways to make it less onerous? How can users be supported and celebrated for the impact of their efforts?
Leaders shouldn’t opt out of learning once vendor contracts are signed. Instead, learn what’s working and what can be improved. To the extent that new tools may cause changes to the organizational chart, create training pathways for new roles.
SUMMARY
There is no magic course you can take on how to conquer the pharmaceutical industry with AI: It doesn’t exist because nobody’s done it yet. That means “How should we train our people?” is not really the right question. Instead, it is better to ask, “How can we continue to learn and improve together?”
It can be instructive to think back to the transformations in automation that were occurring 20 years ago. Today, it is easy to find training on how to use liquid handling in your lab or which methods are best in class and why. But 20 years ago, those training courses did not exist. That’s where we are with AI and ML today.
Bring together the right people internally and the right partners externally — and make continuous learning an organizational identity — and perhaps your company will be the case study of the future.
REFERENCES
- https://store.hbr.org/product/prediction-machines-updated-and-expanded-the-simple-economics-of-artificial-intelligence/10598
- https://mit-online.getsmarter.com/presentations/lp/mit-sloan-artificial-intelligence-in-pharma-and-biotech-online-short-course/
- Nat Mater. 2019 May; 18(5): 435–441. Published online 2019 Apr 18. doi: 10.1038/s41563-019-0338-z
- https://www.bio.org/events/bio-international-convention/sessions/241940.
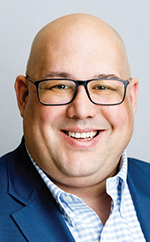
Dave Watrous is VP, Customer Success at IDBS and brings over 20 years of experience in leading teams in the CDMO, CRO, and Life Science markets. Before IDBS, he held roles in BioPharma companies including Covance and Cytiva. He has an academic background in the life sciences, with a BSc in Genetics, Cell Biology & Development, and another in Biochemistry, both from the University of Minnesota. He then pursued graduate studies in Cancer Biology at the University of Wisconsin.
Total Page Views: 4109